Tag Archives: Data Science
How to Add Rows to DataFrames in R Using dplyr: Examples
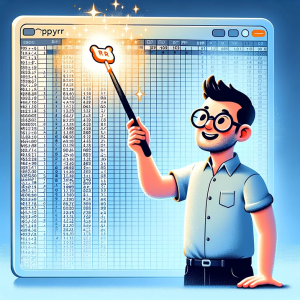
Data manipulation is a fundamental aspect of data analysis, and R, with its dplyr package, offers an efficient and readable way to perform such tasks. In my experience working with various datasets, I have often encountered situations where I needed to add rows to an existing DataFrame. The dplyr package, part of the tidyverse collection, makes these tasks intuitive and efficient. In this blog post, I’ll share two common scenarios: adding a single row and adding multiple rows to a DataFrame using dplyr. If you would want to learn about how to add rows to Pandas Dataframe using Python, check out my related post – Pandas Dataframe: How to Add …
Two samples Z-test for Means: Formula & Examples
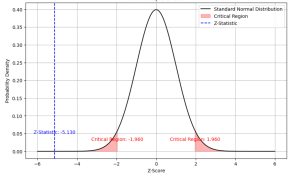
Last updated: 21st Nov, 2023 Statistical hypothesis testing is an essential tool in inferential statistics that enables researchers to make informed decisions about the population parameters based on sample statistics. One common hypothesis test for comparing two sample means is the Two-Sample Z-Test. In statistics, a two-sample z-test for means is used to determine if the means of two populations are equal. This test is used when the population standard deviations are known. As data scientists, it is of utmost importance to be able to understand and conduct this test accurately. In this blog, we will delve deeper into the Two-Sample Z-Test for means, exploring its formula, assumptions, and examples …
Histogram Plots using Matplotlib & Pandas: Python
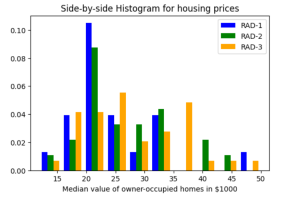
Executing the above code will print the following Histogram. Plotting multiple Histograms Side-by-Side using Matplotlib & Pandas When you want to understand the distribution of data with respect to different characteristics, you could plot the side-by-side or multiple histograms on the same plot. For example, when you want to understand the distribution of housing prices with respect to different values of accessibility to radial highways, you would want to print the histograms side-by-side on the same plot. Here is the code representing the printing of histogram plots side-by-side on the same plot: Here is how the side-by-side histogram plot would look like: Creating Stacked Histogram Plots using Matplotlib & Pandas …
Confusion Matrix Concepts, Python Code Examples
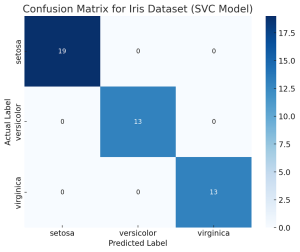
The confusion matrix is an essential tool in the field of machine learning and statistics for evaluating the performance of a classification model. It’s particularly useful when dealing with binary or multi-class classification problems. In this post, you will learn about the confusion matrix with examples and how it could be used as performance metrics for classification models in machine learning. What is Confusion Matrix? A confusion matrix is a table used to describe the performance of a classification model on a set of test data for which the true values are known. It’s most useful when you need to know more about the accuracy of the model than just …
Maximum Likelihood Estimation: Concepts, Examples
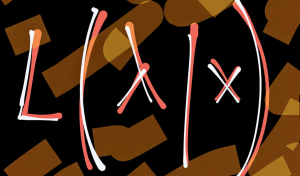
Maximum Likelihood Estimation (MLE) is a fundamental statistical method for estimating the parameters of a statistical model that make the observed data most probable. MLE is grounded in probability theory, providing a strong theoretical basis for parameter estimation. This is becoming more so important to learn fundamentals of MLE concepts as it is at the core of generative modeling (generative AI). Many models used in machine learning and statistics are based on MLE, including logistic regression, survival models, and various types of machine learning algorithms. MLE is particularly important for data scientists because it underpins many of the probabilistic machine learning models that are used today. These models, which are …
Wilcoxon Signed Rank Test: Concepts, Examples
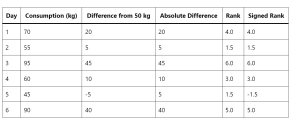
How can data scientists accurately analyze data when faced with non-normal distributions or small sample sizes? This is a challenge that often arises in the dynamic field of data science, where making precise inferences is crucial. Enter the Wilcoxon Signed Rank Test—a non-parametric statistical method that stands as a powerful alternative to the traditional t-test. This blog post aims to unravel the concepts and practical applications of the Wilcoxon Signed Rank Test, offering key insights for data scientists and researchers navigating complex data landscapes. The beauty of the Wilcoxon Signed Rank Test lies in its wide applicability across numerous fields. From healthcare, where it can compare the efficacy of different …
R-squared in Linear Regression Models: Concepts, Examples
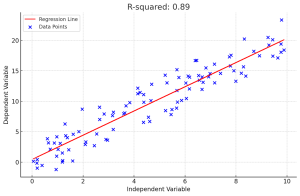
In linear regression, R-squared (R2) is a measure of how close the data points are to the fitted line. It is also known as the coefficient of determination. Understanding the concept of R-squared is crucial for data scientists as it helps in evaluating the goodness of fit in linear regression models, compare the explanatory power of different models on the same dataset and communicate the performance of their models to stakeholders. In this post, you will learn about the concept of R-Squared in relation to assessing the performance of multilinear regression machine learning model with the help of some real-world examples explained in a simple manner. Before doing a deep dive, …
Hierarchical Clustering: Concepts, Python Example
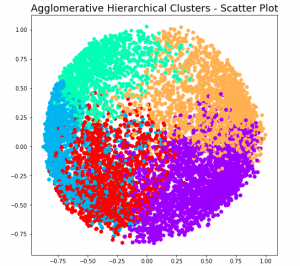
Hierarchical clustering a type of unsupervised machine learning algorithm that stands out for its unique approach to grouping data points. Unlike its counterparts, such as k-means, it doesn’t require the predetermined number of clusters. This feature alone makes it an invaluable method for exploratory data analysis, where the true nature of data is often hidden and waiting to be discovered. But the capabilities of hierarchical clustering go far beyond just flexibility. It builds a tree-like structure, a dendrogram, offering insights into the data’s relationships and similarities, which is more than just clustering—it’s about understanding the story your data wants to tell. In this blog, we’ll explore the key features that …
Minimum Description Length (MDL): Formula, Examples
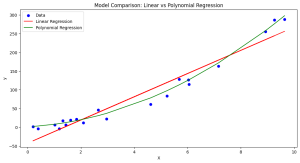
Learning the concepts of Minimum Description Length (MDL) is valuable for several reasons, especially for those involved in statistics, machine learning, data science, and related fields. One of the fundamental problems in statistics and data analysis is choosing the best model from a set of potential models. The challenge is to find a model that captures the essential features of the data without overfitting. This is where methods such as MDL, AIC, BIC, etc. comes to rescue. MDL offers a principled way to balance model complexity against the goodness of fit. This is crucial in many areas, such as machine learning and statistical modeling, where overfitting is a common problem. …
Pearson vs Spearman: Choosing the Right Correlation Coefficient
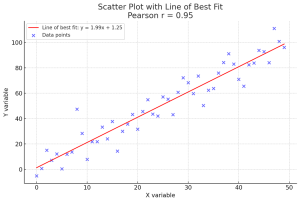
Are you as a data scientist trying to decipher relationship between two or more variables within vast datasets to solve real-world problems? Whether it’s understanding the connection between physical exercise and heart health, or the link between study habits and exam scores, uncovering these relationships is crucial. But with different methods at our disposal, how do we choose the most suitable one? This is where the concept of correlation comes into play, and particularly, the choice between Pearson and Spearman correlation coefficients becomes pivotal. The Pearson correlation coefficient is the go-to metric when both variables under consideration follow a normal distribution, assuming there’s a linear relationship between them. Conversely, the …
Pearson Correlation Coefficient: Formula, Examples
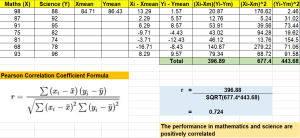
In the world of data science, understanding the relationship between variables is crucial for making informed decisions or building accurate machine learning models. Correlation is a fundamental statistical concept that measures the strength and direction of the relationship between two variables. However, without the right tools and knowledge, calculating correlation coefficients and p-values can be a daunting task for data scientists. This can lead to suboptimal decision-making, inaccurate predictions, and wasted time and resources. In this post, we will discuss what Pearson’s r represents, how it works mathematically (formula), its interpretation, statistical significance, and importance for making decisions in real-world applications such as business forecasting or medical diagnosis. We will …
t-distribution vs Normal distribution: Differences, Examples
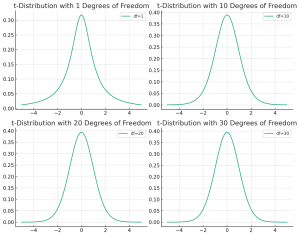
Understanding the differences between the t-distribution and the normal distribution is crucial for anyone delving into the world of statistics, whether they’re students, professionals in research, or data enthusiasts trying to make sense of the world through numbers. But why should one care about the distinction between these two statistical distributions? The answer lies in the heart of hypothesis testing, confidence interval estimation, and predictive modeling. When faced with a set of data, choosing the correct distribution to describe it can greatly influence the accuracy of your conclusions. The normal distribution is often the default assumption due to its simplicity and the central limit theorem, which states that the means …
Problems with Categorical Variables: Examples
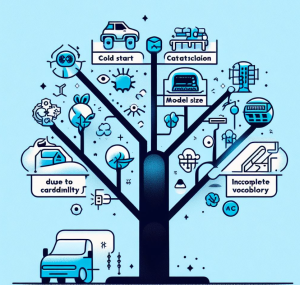
Have you ever encountered unfamiliar words while learning a new language and didn’t know their meanings? Or tried to fit all your belongings into a suitcase, only to realize it’s too full? Or started reading a book series from the third book and felt lost? These scenarios in our daily lives surprisingly resemble some challenges we face with categorical variables in machine learning. Categorical variables, while essential in many datasets, bring with them a unique set of challenges. In this article, we’ll be discussing three major problems associated with categorical features: Let’s explore each with real-life examples and supporting Python code snippets. Incomplete Vocabulary The “Incomplete Vocabulary” problem arises when …
Data Analytics for Car Dealers: Actionable Insights
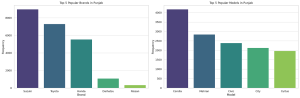
Are you starting a car dealership and wondering how to leverage data to make informed business decisions? In today’s data-driven world, analytics can be the difference between a thriving business and a failing one. This blog aims to provide actionable insights for car dealers, especially those starting new car dealer business, to excel in various business aspects. I will cover inventory management, pricing strategy, marketing and sales, customer service, and risk mitigation, all backed by data analytics. I will continue to update this blog with more methods in time to come. The data used for analysis can be found on the Kaggle.com – Ultimate Car Price Prediction Dataset. First and …
Insurance & Linear Regression Model Example
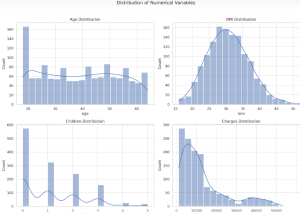
Ever wondered how insurance companies determine the premiums you pay for your health insurance? Predicting insurance premiums is more than just a numbers game—it’s a task that can impact millions of lives. In this blog, we’ll demystify this complex process by walking you through an end-to-end example of predicting health insurance premium charges by demonstrating with Python code example. Specifically, we’ll use a linear regression model to predict these charges based on various factors like age, BMI, and smoking status. Whether you’re a beginner in data science or a seasoned professional, this blog will offer valuable insights into building and evaluating regression models. What is Linear Regression? Linear Regression is …
Chi-square test – Formula, Concepts, Examples
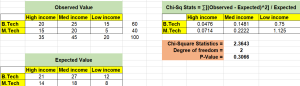
The Pearson’s Chi-square (χ2) test is a statistical test used to determine whether the distribution of observed data is consistent with the distribution of data expected under a particular hypothesis. The Chi-square test can be used to compare or evaluate the independence of two distributions, or to assess the goodness of fit of a given distribution to observed data. In this blog post, we will discuss different types of Chi-square tests, the concepts behind them, and how to perform them using Python / R. As data scientists, it is important to have a strong understanding of the Chi-square test so that we can use it to make informed decisions about …
I found it very helpful. However the differences are not too understandable for me