Tag Archives: sklearn
K-Fold Cross Validation in Machine Learning – Python Example
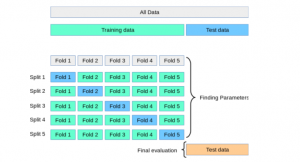
Last updated: 3rd Jan, 2024 In this post, you will learn about K-fold Cross-Validation concepts used while training machine learning models with the help of Python code examples. K-fold cross-validation is a data splitting technique that can be implemented with k > 1 folds. K-Fold Cross Validation is also known as k-cross, k-fold cross-validation, k-fold CV, and k-folds. The k-fold cross-validation technique can be implemented easily using Python with scikit learn (Sklearn) package which provides an easy way to implement training of k-fold cross-validation models. It is important to learn the concepts of k-fold cross-validation concepts in order to perform model tuning with the end goal to choose a model which has …
Micro-average, Macro-average, Weighting: Precision, Recall, F1-Score
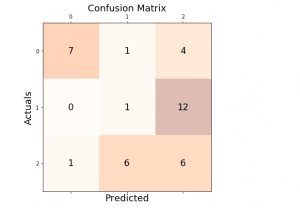
Last updated: 30th Dec, 2023 In this post, you will learn about how to use micro-averaging and macro-averaging methods for evaluating scoring metrics (precision, recall, f1-score) for multi-class classification machine learning problem. You will also learn about weighting method used as one of the other averaging choices of metrics such as precision, recall and f1-score for multi-class classification problem. The concepts will be explained with Python code examples. What & Why of Micro, Macro-averaging and Weighting metrics? Micro and macro-averaging methods are used in the evaluation of classification models, to compute performance metrics like precision, recall, and F1-score. These methods are especially relevant in scenarios involving multi-class or multi-label classification. In case of multi-class classification, …
ROC Curve & AUC Explained with Python Examples
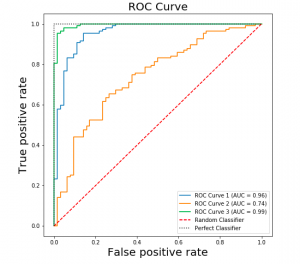
Last updated: 29th Dec, 2023 Confusion among data scientists regarding ROC Curve and AUC often stems from misunderstanding their relationship. The ROC Curve visualizes true positive vs false positive rates at various thresholds, while AUC quantifies the overall ability of a model to discriminate between classes, with higher values indicating better performance. In this post, you will learn about ROC Curve and AUC concepts along with related concepts such as True positive and false positive rate with the help of Python examples. It is very important to learn ROC, AUC and related concepts as it helps in selecting the most appropriate machine learning classification models based on the model performance. What is …
Accuracy, Precision, Recall & F1-Score – Python Examples
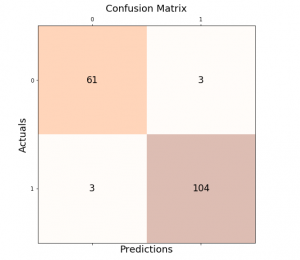
Last updated: 29th Dec, 2023 Classification models are used in classification problems to predict the target class of the data sample. The classification machine learning models predicts the probability that each instance belongs to one class or another. It is important to evaluate the performance of the classifications model in order to reliably use these models in production for solving real-world problems. The performance metrics include accuracy, precision, recall, and F1-score. Because it helps us understand the strengths and limitations of these models when making predictions in new situations, model performance is essential for machine learning. The most common question asked is what is accuracy, precision, recall and f1 score? In …
Lasso Regression in Machine Learning: Python Example
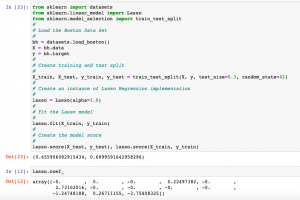
Last updated: 6th Dec, 2023 Lasso regression, sometimes referred to as L1 regularization, is a technique in linear regression that incorporates regularization to curb overfitting and enhance the performance of machine learning models. It works by adding a penalty term to the cost function that encourages the model to select only the most important features and set the coefficients of less important features to zero. This makes Lasso regression a popular method for feature selection and high-dimensional data analysis. In this post, you will learn concepts, formula, advantages and limitations of Lasso regression along with Python Sklearn examples. The other two similar forms of regularized linear regression are Ridge regression and …
Handling Class Imbalance in Machine Learning: Python Example
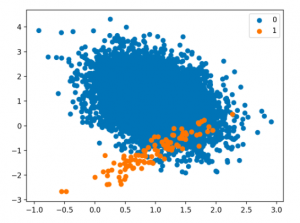
Techniques for Handling Class Imbalance Class imbalance may not always impact performance, and using imbalance-specific methods can sometimes worsen results. Xu-Ying Liu, Jianxin Wu, and Zhi-Hua Zhou, Exploratory Undersampling for Class-Imbalance Learning Above said, there are different techniques such as the following for handling class imbalance when training machine learning models with datasets having imbalanced classes. Python packages such as Imbalanced Learn can be used to apply techniques related to under-sampling majority classes, upsampling minority classes, and SMOTE. In this post, techniques related to using class weight will be used for tackling class imbalance. How to create a Sample Dataset having Class Imbalance? In this section, you will learn about how to create an …
Handling Class Imbalance using Sklearn Resample
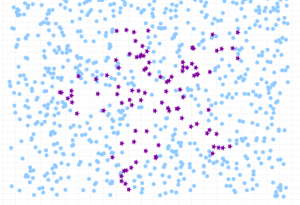
Last updated: 5th Dec, 2023 The class imbalance problem in machine learning occurs when the classes in a dataset are not represented equally, leading to a significant difference in the number of instances for different classes. This imbalance can cause a classification model to be biased towards the majority class, resulting in poor performance on the minority class. Thus, the class imbalance hinders data scientists by challenging the development of accurate and fair models, as the skewed distribution can lead to misleading training predictions / outcomes and reduced effectiveness in real-world applications where minority classes are critical. In this post, you will learn about how to tackle class imbalance issue …
Learning Curves Python Sklearn Example
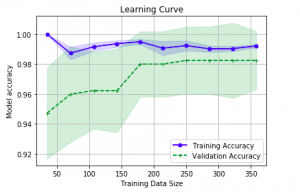
Last updated: 26th Nov, 2023 In this post, you will learn about how to use learning curves to assess the improvement in learning performance (accuracy, error rate, etc.) of a machine learning model while implementing using Python (Sklearn) packages. Knowing how to use learning curves will help you assess/diagnose whether the model is suffering from high bias (underfitting) or high variance (overfitting) and whether increasing training data samples could help solve the bias or variance problem. You may want to check some of the following posts in order to get a better understanding of bias-variance and underfitting-overfitting. Bias-variance concepts and interview questions Overfitting/Underfitting concepts and interview questions What are learning curves? …
Bagging Classifier Python Code Example
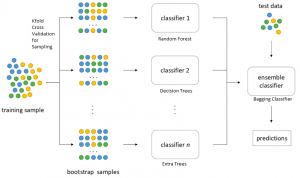
Last updated: 25th Nov, 2023 Bagging is a type of an ensemble machine learning approach that combines the outputs from many learner to improve performance. The bagging algorithm works by dividing the training set into smaller subsets. These subsets are then processed through different machine-learning models. After processing, the predictions from each model are combined. This combination of predictions is used to generate an overall prediction for each instance in the original data. In this blog post, you will learn about the concept of Bagging along with Bagging Classifier Python code example. Bagging can be used in machine learning for both classification and regression problem. The bagging classifier technique is utilized across a …
Confusion Matrix Concepts, Python Code Examples
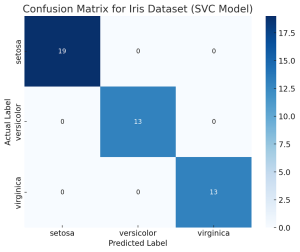
The confusion matrix is an essential tool in the field of machine learning and statistics for evaluating the performance of a classification model. It’s particularly useful when dealing with binary or multi-class classification problems. In this post, you will learn about the confusion matrix with examples and how it could be used as performance metrics for classification models in machine learning. What is Confusion Matrix? A confusion matrix is a table used to describe the performance of a classification model on a set of test data for which the true values are known. It’s most useful when you need to know more about the accuracy of the model than just …
Hierarchical Clustering: Concepts, Python Example
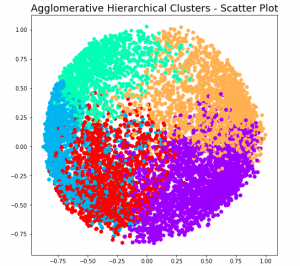
Hierarchical clustering a type of unsupervised machine learning algorithm that stands out for its unique approach to grouping data points. Unlike its counterparts, such as k-means, it doesn’t require the predetermined number of clusters. This feature alone makes it an invaluable method for exploratory data analysis, where the true nature of data is often hidden and waiting to be discovered. But the capabilities of hierarchical clustering go far beyond just flexibility. It builds a tree-like structure, a dendrogram, offering insights into the data’s relationships and similarities, which is more than just clustering—it’s about understanding the story your data wants to tell. In this blog, we’ll explore the key features that …
K-Means Clustering Concepts & Python Example
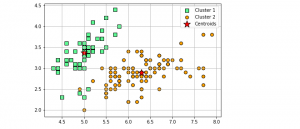
Clustering is a popular unsupervised machine learning technique used in data analysis to group similar data points together. The K-Means clustering algorithm is one of the most commonly used clustering algorithms due to its simplicity, efficiency, and effectiveness on a wide range of datasets. In K-Means clustering, the goal is to divide a given dataset into K clusters, where each data point belongs to the cluster with the nearest mean value. The algorithm works by iteratively updating the cluster centroids until convergence is achieved. In this post, you will learn about K-Means clustering concepts with the help of fitting a K-Means model using Python Sklearn KMeans clustering implementation. You will …
Sklearn Algorithms Cheat Sheet with Examples
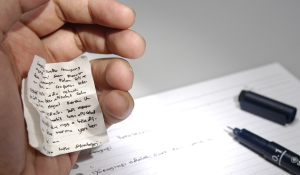
The Sklearn library, short for Scikit-learn, is one of the most popular and widely-used libraries for machine learning in Python. It offers a comprehensive set of tools for data analysis, preprocessing, model selection, and evaluation. As a beginner data scientist, it can be overwhelming to navigate the various algorithms and functions within Sklearn. This is where the Sklearn Algorithms Cheat Sheet comes in handy. This cheat sheet provides a quick reference guide for beginners to easily understand and select the appropriate algorithm for their specific task. In this cheat sheet, I have compiled a list of common supervised and unsupervised learning algorithms, along with their Sklearn classes and example use …
KMeans Silhouette Score Python Example
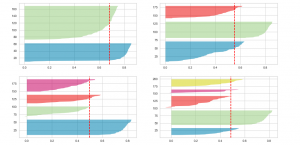
If you’re building machine learning models for solving different prediction problems, you’ve probably heard of clustering. Clustering is a popular unsupervised learning technique used to group data points with similar features into distinct clusters. One of the most widely used clustering algorithms is KMeans, which is popular due to its simplicity and efficiency. However, one major challenge in clustering is determining the optimal number of clusters that should be used to group the data points. This is where the Silhouette Score comes into play, as it helps us measure the quality of clustering and determine the optimal number of clusters. Silhouette score helps us get further clarity for the following …
Python – Draw Confusion Matrix using Matplotlib
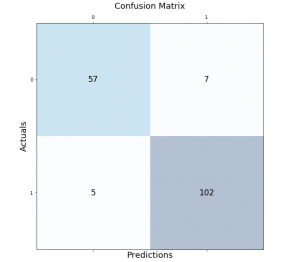
Classification models are a fundamental part of machine learning and are used extensively in various industries. Evaluating the performance of these models is critical in determining their effectiveness and identifying areas for improvement. One of the most common tools used for evaluating classification models is the confusion matrix. It provides a visual representation of the model’s performance by displaying the number of true positives, false positives, true negatives, and false negatives. In this post, we will explore how to create and visualize confusion matrices in Python using Matplotlib. We will walk through the process step-by-step and provide examples that demonstrate the use of Matplotlib in creating clear and concise confusion …
K-Nearest Neighbors (KNN) Python Examples
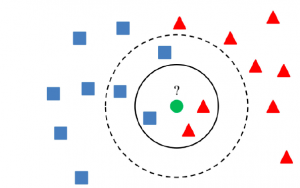
If you’re working with data analytics projects including building machine learning (ML) models, you’ve probably heard of the K-nearest neighbors (KNN) algorithm. But what is it, exactly? And more importantly, how can you use it in your own AI / ML projects? In this post, we’ll take a closer look at the KNN algorithm and walk through a simple Python example. You will learn about the K-nearest neighbors algorithm with Python Sklearn examples. K-nearest neighbors algorithm is used for solving both classification and regression machine learning problems. Stay tuned! Introduction to K-Nearest Neighbors (K-NN) Algorithm K-nearest neighbors is a supervised machine learning algorithm for classification and regression. In both cases, the input consists …
I found it very helpful. However the differences are not too understandable for me