Category Archives: Data Science
Loss Function vs Cost Function vs Objective Function: Examples
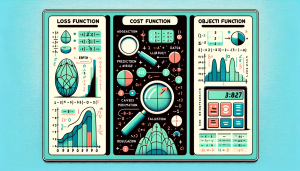
Last updated: 19th April, 2024 Among the terminologies used in training machine learning models, the concepts of loss function, cost function, and objective function often cause a fair amount of confusion, especially for aspiring data scientists and practitioners in the early stages of their careers. The reason for this confusion isn’t unfounded, as these terms are similar / closely related, often used interchangeably, and yet, they are different and serve distinct purposes in the realm of machine learning algorithms. Understanding the differences and specific roles of loss function, cost function, and objective function is more than a mere exercise in academic rigor. By grasping these concepts, data scientists can make …
Free IBM Data Sciences Courses on Coursera
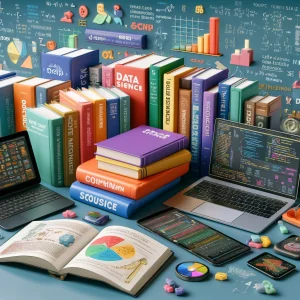
In the rapidly evolving fields of Data Science and Artificial Intelligence, staying ahead means continually learning and adapting. In this blog, there is a list of around 20 free data science-related courses from IBM available on coursera.org that can help data science enthusiasts master different domains in AI / Data Science / Machine Learning. This list includes courses related to the core technical skills and knowledge needed to excel in these innovative fields. Foundational Knowledge: Understanding the essence of Data Science lays the groundwork for a successful career in this field. A solid foundation helps you grasp complex concepts easily and contributes to better decision-making, problem-solving, and the capacity to …
Self-Supervised Learning vs Transfer Learning: Examples
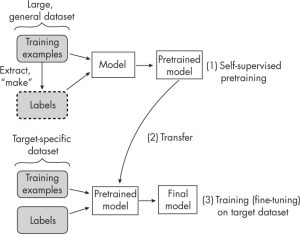
Last updated: 3rd March, 2024 Understanding the difference between self-supervised learning and transfer learning, along with their practical applications, is crucial for any data scientist looking to optimize model performance and efficiency. Self-supervised learning and transfer learning are two pivotal techniques in machine learning, each with its unique approach to leveraging data for model training. Transfer learning capitalizes on a model pre-trained on a broad dataset with diverse categories, to serve as a foundational model for a more specialized task. his method relies on labeled data, often requiring significant human effort to label. Self-supervised learning, in contrast, pre-trains models using unlabeled data, creatively generating its labels from the inherent structure …
Large Language Models (LLMs): Types, Examples
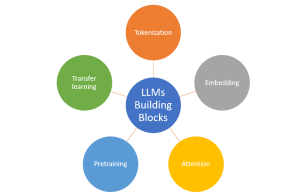
Last updated: 31st Jan, 2024 Large language models (LLMs), being the key pillar of generative AI, have been gaining traction in the world of natural language processing (NLP) due to their ability to process massive amounts of text and generate accurate results related to predicting the next word in a sentence, given all the previous words. These different LLM models are trained on a large or broad corpus of text datasets, which contain hundreds of millions to billions of words. LLMs, as they are known, rely on complex algorithms including transformer architectures that shift through large datasets and recognize patterns at the word level. This data helps the LLMs better understand …
Amazon (AWS) Machine Learning / AI Services List
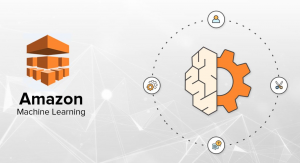
Last updated: 30th Jan, 2024 Amazon Web Services (AWS) is a cloud computing platform that offers machine learning as one of its many services. AWS has been around for over 10 years and has helped data scientists leverage the Amazon AWS cloud to train machine learning models. AWS provides an easy-to-use interface that helps data scientists build, test, and deploy their machine learning models with ease. AWS also provides access to pre-trained machine learning models so you can start building your model without having to spend time training it first! You can get greater details on AWS machine learning services, data science use cases, and other aspects in this book – …
How is ChatGPT Trained to Generate Desired Responses?
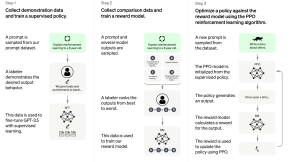
Last updated: 27th Jan, 2024 Training an AI / Machine Learning model as sophisticated as the one used by ChatGPT involves a multi-step process that fine-tunes its ability to understand and generate human-like text. Let’s break down the ChatGPT training process into three primary steps. Note that OpenAI has not published any specific paper on this. However, the reference has been provided on this page – Introducing ChatGPT. Fine-tuning Base Model with Supervised Learning The first phase starts with collecting demonstration data. Here, prompts are taken from a dataset, and human labelers provide the desired output behavior, which essentially sets the standard for the AI’s responses. For example, if the …
Generalization Errors in Machine Learning: Python Examples
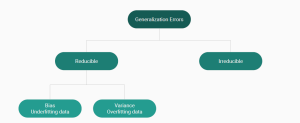
Last updated: 21st Jan, 2024 Machine Learning (ML) models are designed to make predictions or decisions based on data. However, a common challenge, data scientists face when developing these models is ensuring that they generalize well to new, unseen data. Generalization refers to a model’s ability to perform accurately on new, unseen examples after being trained on a limited set of data. When models don’t generalize well, they commit errors. These errors are called generalization errors. In this blog, you will learn about different types of generalization errors, with examples, and walk through a simple Python demonstration to illustrate these concepts. Types of Generalization Errors Generalization errors in machine learning …
NLP Corpus Types (Text & Multimodal): Examples
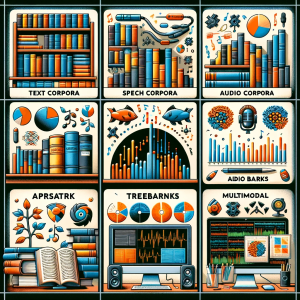
At the heart of NLP lies a fundamental element: the corpus. A corpus, in NLP, is not just a collection of text documents or utterances; it’s at the core of large language models (LLMs) training. Each corpus type serves a unique purpose in terms of training language models that serve different purposes. Whether it’s a collection of written texts, transcriptions of spoken words, or an amalgamation of various media forms, each corpus type holds the key to leveraging different aspects of language to generate value. In this blog, we’re going to explore the significance of these different corpora types in NLP. From the traditional text corpora consisting of written content …
NLP: Different Types of Language Models – Examples
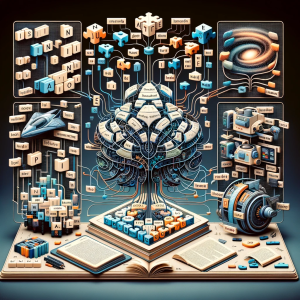
Have you ever wondered how your smartphone seems to know exactly what you’re going to type next? Or how virtual assistants like Alexa and Siri understand and respond to your queries with such precision? The magic is NLP language models. In this blog, we will explore the diverse types of language models in NLP that have evolved over time, each with its unique capabilities and applications. From the simplicity of N-gram models, which predict text based on preceding words, to the sophisticated neural network-based models like RNNs, LSTMs, and the groundbreaking large language models using Transformers, we will learn about the intricacies of these models, examples of real-world applications and …
Bag of Words in NLP & Machine Learning: Examples
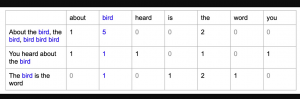
Last updated: 6th Jan, 2024 Most machine learning algorithms require numerical input for training the models. Bag of words (BoW) effectively converts text data into numerical feature vectors, making it compatible with a wide range of machine learning algorithms, from linear classifiers like logistic regression to complex ones like neural networks. In this post, you will learn about the concepts of bag-of-words model and how to train a text classification model using Python Sklearn. Some of the most common text classification problems includes sentiment analysis, spam filtering etc. In these problems, one can apply bag-of-words technique to train machine learning models for text classification. It will be good to understand the …
Natural Language Processing (NLP) Task Examples
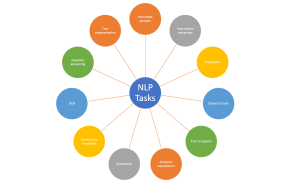
Last updated: 5th Jan, 2024 Have you ever wondered how your phone’s voice assistant understands your commands and responds appropriately? Or how search engines are able to provide relevant results for your queries? The answer lies in Natural Language Processing (NLP), a subfield of artificial intelligence (AI) that focuses on enabling machines to understand and process human language. NLP is becoming increasingly important in today’s world as more and more businesses are adopting AI-powered solutions to improve customer experiences, automate manual tasks, and gain insights from large volumes of textual data. With recent advancements in AI technology, it is now possible to use pre-trained language models such as ChatGPT to …
Cohen Kappa Score Explained: Formula, Example
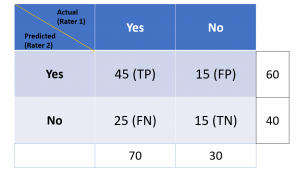
Last updated: 5th Jan, 2024 Cohen’s Kappa Score is a statistic used to measure the performance of machine learning classification models. In this blog post, we will discuss what Cohen’s Kappa Score is and Python code example representing how to calculate Kappa score using Python. We will also provide a code example so that you can see how it works! What is Cohen’s Kappa Score or Coefficient? Cohen’s Kappa Score, also known as the Kappa Coefficient, is a statistical measure of inter-rater agreement for categorical data. Cohen’s Kappa Coefficient is named after statistician Jacob Cohen, who developed the metric in 1960. It is generally used in situations where there …
Validation Techniques for Machine Learning Models: Examples
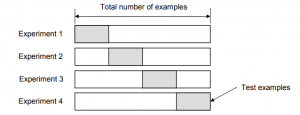
Last updated: 4th Jan, 2024 In the realm of machine learning, the emphasis increasingly shifts towards solving real-world problems with high-quality models. Creating high performant models does not not just depend on raw computational power or theoretical knowledge, but crucially on the ability to systematically conduct and learn from a myriad of different models by trying with hypothesis and related experiments including different algorithms, datasets / features, hyperparameters, etc. This is where the importance of a robust validation strategy and related techniques becomes paramount. Validation techniques, in essence, are the methodologies employed to accurately assess a model’s errors and to gauge how its performance fluctuates with different experiments. The primary …
Machine Learning Definition, Examples, Method, Types
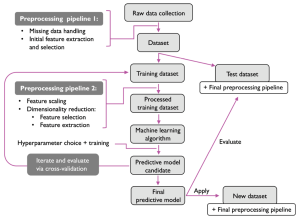
Last updated: 3rd Jan, 2024 Machine learning is a machine’s ability to learn from data. It has been around for decades, but machine learning is now being applied in nearly every industry and job function. In this blog post, we’ll cover a detailed introduction to what is machine learning (ML) including different definitions. We will also learn about different types of machine learning tasks, algorithms, etc along with real-world examples. What is machine learning & how does it work? Definition 1: Simply speaking, machine learning can be defined as an approach to model our beliefs about real-world events. For example, let’s say a person came to a doctor with a …
Machine Learning Models Solution Design: Examples
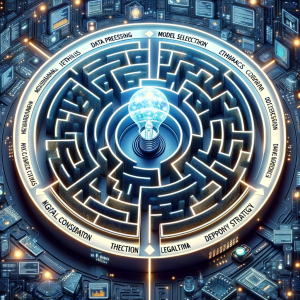
This blog is crafted for data scientists, machine learning (ML) and software engineers, business analysts / product managers, and anyone involved in the ML project lifecycle, aiming to create a reliable solution design and development strategy / plan for successful AI / machine learning project implementation and value realization. The blog revolves around a series of critical solution design questions, meticulously curated to guide teams from the initial conception of a project to its final deployment and beyond. By addressing each of these solution design questions, teams can ensure that they are not only building a model that is technically proficient but also one that aligns seamlessly with business objectives, …
Micro-average, Macro-average, Weighting: Precision, Recall, F1-Score
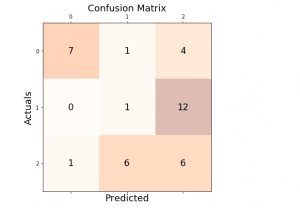
Last updated: 30th Dec, 2023 In this post, you will learn about how to use micro-averaging and macro-averaging methods for evaluating scoring metrics (precision, recall, f1-score) for multi-class classification machine learning problem. You will also learn about weighting method used as one of the other averaging choices of metrics such as precision, recall and f1-score for multi-class classification problem. The concepts will be explained with Python code examples. What & Why of Micro, Macro-averaging and Weighting metrics? Micro and macro-averaging methods are used in the evaluation of classification models, to compute performance metrics like precision, recall, and F1-score. These methods are especially relevant in scenarios involving multi-class or multi-label classification. In case of multi-class classification, …
I found it very helpful. However the differences are not too understandable for me