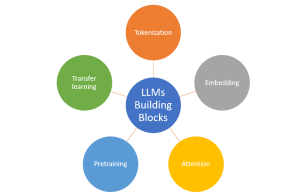
Last updated: 31st Jan, 2024
Large language models (LLMs), being the key pillar of generative AI, have been gaining traction in the world of natural language processing (NLP) due to their ability to process massive amounts of text and generate accurate results related to predicting the next word in a sentence, given all the previous words. These different LLM models are trained on a large or broad corpus of text datasets, which contain hundreds of millions to billions of words. LLMs, as they are known, rely on complex algorithms including transformer architectures that shift through large datasets and recognize patterns at the word level. This data helps the LLMs better understand natural language and how it is used in context and then make predictions related to various NLP tasks such as text generation, summarization, translation, text classification, and even answering questions with a high degree of accuracy.
Large Language Models (LLMs) like those belonging to the GPT, and BERT family have demonstrated a significant advancement over earlier neural network architectures such as Recurrent Neural Networks (RNNs) in the field of Natural Language Processing (NLP). The following is the trend of the number of LLMs introduced over the years. Check out this paper for further detail: A comprehensive overview of LLMs
This blog post aims to provide a comprehensive understanding of large language models, their importance, and their applications in various NLP tasks. We will discuss how these different LLM models work, LLM examples, and the training process involved in creating them. By the end of this post, you should have a solid understanding of why large language models are essential building blocks of today’s AI / generative AI applications.
What are Large Language Models (LLMs)? Why are they called “Large”?
Large Language Models (LLMs) are a class of deep learning models designed to process and understand vast amounts of natural language data. Simply speaking, large language models can be defined as AI/machine learning models that try to solve NLP tasks related to text generation, summarization, translation, question & answering (Q&A), etc., thereby enabling more effective human-machine communication. This is why LLMs need to process & understand huge volumes of text data and learn patterns and relationships between words in sentences.
Large language models (LLMs) are called “large” because they are pre-trained with a large number of parameters (100M+) on large corpora of text to process/understand and generate natural language text for a wide variety of NLP tasks. The LLM family includes BERT (NLU – Natural language understanding), GPT (NLG – natural language generation), T5, etc. The specific LLM models such as OpenAI’s models (GPT3.5, GPT-4 – Billions of parameters), PaLM2, Llama 2, etc demonstrate exceptional performance in various NLP / text processing tasks mentioned before. Some of these LLMs are open-sourced (Llama 2) while other ain’t (such as ChatGPT models).
Attention Mechanism: Fundamental to LLMs
LLMs are built on neural network architectures, particularly the transformer architecture (check the paper, Attention is all you need), which allows them to capture complex language patterns and relationships between words or phrases in large-scale text datasets. LLMs can also be understood as variants of transformer architecture. The transformer architecture relies on attention mechanisms such as self-attention and multihead attention, which allows the model to understand the relationship between words in a text by weighing the importance of different words or phrases in a given context.
- Self-attention mechanism is a mechanism that allows each position in the input sequence to attend to all positions in the same sequence. In other words, it helps the model to understand and interpret a sequence by considering the entire sequence. For instance, when processing a sentence, self-attention allows each word to be contextualized in relation to every other word in that sentence.
- Multihead attention or Multihead self-attention in a transformer represents multiple attention heads where each attention head learns a distinct attention mechanism so that the layer as a whole can learn more complex relationships.
Neural Network Architecture Building Blocks for LLMs
The transformer architecture represents the neural network model for natural language processing tasks based on encoder-decoder architecture, which was introduced in the paper “Attention Is All You Need” by Vaswani et al. in 2017. The transformer architecture consists of two main components: the encoder network and the decoder network.
- Encoder network takes an input sequence and produces a sequence of hidden states. For example, let’s say, the encoder network takes a sequence of words in the source language, say English.
- Input sequence example: For example, consider the sentence “The cat sat on the mat“.
- Input Processing: The encoder processes this sequence word by word. Each word is first converted into a numerical form (like a vector) that represents its meaning. This is typically done using word embeddings.
- Sequence of Hidden States: As it processes each word, the encoder uses self-attention mechanisms to understand the context of each word about every other word in the sentence. This results in a sequence of hidden states, each state being a vector that encodes the contextual information of a word in the sentence. For instance, the hidden state for “cat” would capture not just the meaning of “cat” but also its relationship to “sat,” “on,” “the,” and “mat” in the sentence.
- Decoder network takes a target sequence and uses the encoder’s output to generate a sequence of predictions.
- Translation task example: For example, let’s say, the decoder network aims to generate the translation in the target language, say French. It starts with a starting token (like “<start>”) and generates one word at a time.
- Using Encoder’s Output: The decoder uses the sequence of hidden states produced by the encoder to understand the context of the source sentence.
- Sequence of Predictions: For each word it generates, the decoder uses cross-attention mechanisms to focus on different parts of the encoder’s output. This helps the decoder figure out which words in the English sentence are most relevant to the current word it is trying to predict in French. For example, when translating “The cat sat on the mat” to French, the decoder might focus on the hidden state for “cat” when trying to predict the French word for “cat.”
- Iterative Process: This process is iterative. With each word generated in French, the decoder updates its state and makes the next prediction, until the entire sentence is translated.
Types of LLMs vs Transformer Architecture: Examples
While the original Transformer model consists of both encoder and decoder blocks composed of multiple layers of self-attention, cross-attention, and feedforward neural networks, different types of LLMs may use variations of this transformer architecture. depending upon its intended application. Check this post for greater details – Transformer Architecture Types: Explained with Examples. The picture given below represents the original transformer architecture representing a bunch of multiple-head attention mechanisms and feed-forward networks.
There are three different LLM types based on the above transformer architecture using encoder, decoder, or both networks.
- Autoregressive Language Models (e.g., GPT): Autoregressive models primarily use the decoder part of the Transformer architecture, making them well-suited for natural language generation (NLG) tasks like text summarization, generation, etc. These models generate text by predicting the next word in a sequence given the previous words. They are trained to maximize the likelihood of each word in the training dataset, given its context. The most well-known example of an autoregressive language model is OpenAI’s GPT (Generative Pre-trained Transformer) series, with GPT-4 being the latest and most powerful iteration. Autoregressive models based on decoder networks primarily leverage layers related to self-attention, cross-attention mechanisms, and feed-forward networks as part of their neural network architecture.
- Autoencoding Language Models (e.g., BERT): Autoencoding models, on the other hand, mainly use the encoder part of the Transformer. It’s designed for tasks like classification, question answering, etc. These models learn to generate a fixed-size vector representation (also called embeddings) of input text by reconstructing the original input from a masked or corrupted version of it. They are trained to predict missing or masked words in the input text by leveraging the surrounding context. BERT (Bidirectional Encoder Representations from Transformers), developed by Google, is one of the most famous autoencoding language models. It can be fine-tuned for a variety of NLP tasks, such as sentiment analysis, named entity recognition and question answering. Autoencoding models based on encoder networks primarily leverage layers related to self-attention mechanisms and feed-forward networks as part of their neural network architecture.
- The third one is the combination of autoencoding and autoregressive such as the T5 (Text-to-Text Transfer Transformer) model. Developed by Google in 2020, T5 LLM can perform natural language understanding (NLU) and natural language generation (NLG). T5 LLM can be understood as a pure transformer using both encoder and decoder networks.
Different LLM Models & Use Case Scenarios
While traditional NLP algorithms typically only look at the immediate context of words, LLMs consider large swaths of text to better understand the context. Here are two LLM examples scenarios showcasing the use of autoregressive and autoencoding LLMs for text generation and text completion, respectively.
Autoregressive LLMs Example
Let’s take an example of how autoregressive models work. As learned earlier, the autoregressive models such as GPT, generate a coherent and contextually relevant sentence based on the given input prompt.
Let’s say the input to the autoregressive model is the following:
“Introducing new smartphone, the UltraPhone 3000, which is designed to”
The generated text can be:
“redefine your mobile experience with its cutting-edge technology and unparalleled performance.”
Autoencoding LLMs Example
Let’s take another example of how autoencoding models work. As learned earlier, autoencoding models, such as BERT, are used to fill in the missing or masked words in a sentence, producing a semantically meaningful and complete sentence.
Let’s say the input to the autoencoding model is the following:
The latest superhero movie had an _______ storyline, but the visual effects were _______.
The completed text will look like the following:
The latest superhero movie had a decent storyline, but the visual effects were mind-blowing.
How does LLM work? Key Building Blocks
Large Language Models (LLMs) are composed of several key building blocks that enable them to efficiently process and understand natural language data.
The following is an overview of some of the critical components:
- Tokenization: Tokenization is the process of converting a sequence of text into individual words, subwords, or tokens that the model can understand. In LLMs, tokenization is usually performed using subword algorithms like Byte Pair Encoding (BPE) or WordPiece, which split the text into smaller units that capture both frequent and rare words. This approach helps to limit the model’s vocabulary size while maintaining its ability to represent any text sequence.
- Embedding: Embeddings are continuous vector representations of words or tokens that capture their semantic meanings in a high-dimensional space. They allow the model to convert discrete tokens into a format that can be processed by the neural network. In LLMs, embeddings are learned during the training process, and the resulting vector representations can capture complex relationships between words, such as synonyms or analogies.
- Attention: Attention mechanisms in LLMs, particularly the self-attention mechanism used in transformers, allow the model to weigh the importance of different words or phrases in a given context. By assigning different weights to the tokens in the input sequence, the model can focus on the most relevant information while ignoring less important details. This ability to selectively focus on specific parts of the input is crucial for capturing long-range dependencies and understanding the nuances of natural language.
- Pre-training: Pretraining is the process of training an LLM on a large dataset, usually unsupervised or self-supervised, before fine-tuning it for a specific task. During pretraining, the model learns general language patterns, relationships between words, and other foundational knowledge. This process results in a pre-trained model that can be fine-tuned using a smaller, task-specific dataset, significantly reducing the amount of labeled data and training time required to achieve high performance on various NLP tasks.
- Transfer learning: Transfer learning is the technique of leveraging the knowledge gained during pretraining and applying it to a new, related task. In the context of LLMs, transfer learning involves fine-tuning a pre-trained model on a smaller, task-specific dataset to achieve high performance on that task. The benefit of transfer learning is that it allows the model to benefit from the vast amount of general language knowledge learned during pretraining, reducing the need for large labeled datasets and extensive training for each new task.
List of Large Language Models (LLMs): Examples
The following is the chronological display of LLM releases. Light blue rectangles represent “pre-trained” models, while dark rectangles correspond to “instruction-tuned” models. Models on the upper half signify open-source availability, whereas those on the bottom half are closed-source. Check out further details in this paper: A comprehensive overview of large language models.
The following is the list of large language models compared to different parameters.
Model | Disk Size (GB) | Memory Usage (GB) | Parameters (Millions) | Training Data Size (GB) |
---|---|---|---|---|
BERT-Large | 1.3 | 3.3 | 340 | 20 |
GPT-2 117M | 0.5 | 1.5 | 117 | 40 |
GPT-2 1.5B | 6 | 16 | 1,500 | 40 |
GPT-3 175B | 700 | 2,000 | 175,000 | 570 |
T5-11B | 45 | 40 | 11,000 | 750 |
RoBERTa-Large | 1.5 | 3.5 | 355 | 160 |
ELECTRA-Large | 1.3 | 3.3 | 335 | 20 |
If you want to compare and rank the output of 25+ LLMs examples right from your browser, check out Chatbot Arena Leaderboard. The leaderboard lists LLMs such as Gemini Pro, GPT-4-Turbo, GPT-3.5-Turbo, LLaMa-13B, etc.
When to use LLMs? Do I need LLMs for my projects?
Deciding whether to integrate a Large Language Model (LLM) into your project requires a careful evaluation of various factors. With my expertise in AI and machine learning, I can guide you through this process:
- Clearly define your project goals: Determine if your project’s objectives align with the capabilities of LLMs, such as advanced natural language processing, contextual understanding, or generating human-like text. Keep in mind the following capabilities of LLMs and whether you need to address your business problem at hand:
-
Advanced Natural Language Processing (NLP): LLMs can understand and generate human language with a high degree of sophistication. This includes tasks like sentiment analysis, text summarization, and language translation.
-
Contextual Understanding: One of the key strengths of LLMs is their ability to understand context in language. They can infer meaning from preceding text, which is crucial for applications like chatbots, context-sensitive help systems, and personalized content generation.
-
Generating Human-Like Text: LLMs can create text that closely resembles human writing. This is particularly useful for content creation, such as writing articles, generating creative writing, or automating responses in customer service applications.
-
- Analyze your data requirements: LLMs are most effective when dealing with substantial amounts of text or complex linguistic tasks. Assess whether your data necessitates such advanced processing.
- Technical readiness of team: Evaluate if your team possesses the necessary technical skills. Also, consider the infrastructure required for deploying LLMs, like computational resources or cloud services.
- Financial implications vs leadership buy-in: LLMs entail costs related to API usage and infrastructure. It’s crucial to weigh these costs against the potential efficiency gains and value addition to your services or products. Analyze the costs involved in implementing an LLM (like development time, infrastructure costs, and potential API fees) against the expected benefits (like improved efficiency, accuracy, or user satisfaction).
- Compliance & Ethics: Using LLMs must align with data privacy laws. Additionally, ethical considerations, like mitigating biases in language models, are paramount.
- Assess whether you need LLMs to solve your problem: Sometimes, simpler or more specialized tools can suffice. Evaluate if an LLM is genuinely necessary or if there are less complex alternatives.
Industry Use Cases Examples of LLMs
Large Language Models have a wide range of applications due to their advanced natural language processing capabilities. Here are five of the most appropriate and impactful use cases:
1. Customer Service Automation
- Chatbots and Virtual Assistants: LLMs can power sophisticated chatbots and virtual assistants that provide human-like interactions. They can handle customer inquiries, offer support, and provide information 24/7, enhancing customer experience and reducing the workload on human staff. This use case is applicable in different industry domains including e-commerce, banking & financial services, healthcare, etc.
- Email and Social Media Responses: Automating responses to customer emails and social media inquiries can significantly improve response times and consistency in customer service. LLMs are getting used to writing emails by many for different purposes including sales and marketing.
2. Content Creation and Curation
- Automated Journalism and Article Writing: LLMs can generate news articles, blog posts, and other written content quickly and efficiently. They can also be used to curate and summarize content from various sources.
- Creative Writing: In the creative domain, LLMs can assist in generating ideas, dialogues, or even entire plots for stories, scripts, and advertising copy.
3. Language Translation and Localization
- Multilingual Translation: LLMs can provide high-quality translations across multiple languages, facilitating communication in global businesses and helping break language barriers.
- Localization Services: Beyond translation, LLMs can adapt content to specific cultures and locales, ensuring that the translations are culturally appropriate and contextually accurate.
4. Education and Research
- Tutoring and Educational Tools: LLMs can be used to create personalized educational experiences, offering tutoring, language learning assistance, and help in understanding complex subjects.
- Academic Research: In research, LLMs can assist in literature reviews, data analysis, and even in drafting research papers or reports.
5. Data Analysis and Business Intelligence
- Sentiment Analysis: Analyzing customer feedback, market trends, and social media posts to gauge public sentiment on various topics, products, or services.
- Business Reports and Market Analysis: LLMs can synthesize vast amounts of data into coherent and insightful business reports, market analyses, and executive summaries.
These use cases demonstrate the versatility of LLMs in offering solutions that are not only efficient and cost-effective but also enhance user engagement, creativity, and the overall decision-making process in various sectors.
Token-based Pricing for LLMs & Embeddings
The following spreadsheet consists of information about different LLMs and their respective pricing structures. It consists of pricing of each model to the pricing of other models like “GPT-4”, “Amazon Bedrock”, “Claude 2”, “GPT-4 Turbo”, and “GPT-3.5”. Another tab such as “Embeddings” contains information about various text embedding models and their pricing.
https://docs.google.com/spreadsheets/d/1NX8ZW9Jnfpy88PC2d6Bwla87JRiv3GTeqwXoB4mKU_s/edit#gid=0
White Papers for Learning LLMs
White papers are an excellent resource for gaining an in-depth understanding of the concepts and advancements in the field of large language models. From the development of neural machine translation to the latest pre-training methods for natural language generation and comprehension, these papers provide a comprehensive view of the evolution of language models. The following list includes some of the most influential papers in the field:
- Neural Machine Translation by Jointly Learning to Align and Translate (2014) by Bahdanau, Cho, and Bengio, https://arxiv.org/abs/1409.0473
- Attention Is All You Need (2017) by Vaswani, Shazeer, Parmar, Uszkoreit, Jones, Gomez, Kaiser, and Polosukhin, https://arxiv.org/abs/1706.03762
- BERT: Pre-training of Deep Bidirectional Transformers for Language Understanding (2018) by Devlin, Chang, Lee, and Toutanova, https://arxiv.org/abs/1810.04805
- Improving Language Understanding by Generative Pre-Training (2018) by Radford and Narasimhan, https://www.semanticscholar.org/paper/Improving-Language-Understanding-by-Generative-Radford-Narasimhan/cd18800a0fe0b668a1cc19f2ec95b5003d0a5035
- BART: Denoising Sequence-to-Sequence Pre-training for Natural Language Generation, Translation, and Comprehension (2019), by Lewis, Liu, Goyal, Ghazvininejad, Mohamed, Levy, Stoyanov, and Zettlemoyer, https://arxiv.org/abs/1910.13461
- Harnessing the Power of LLMs in Practice: A Survey on ChatGPT and Beyond (2023) by Yang, Jin, Tang, Han, Feng, Jiang, Yin, and Hu, https://arxiv.org/abs/2304.13712
Frequently Asked Questions (FAQs)
Here are a few frequently asked questions about LLMs:
- What’s the NLP & LLM difference?
- Natural Language Processing (NLP) is an AI / Machine Learning technique used to understand and generate human language. It encompasses technologies like speech recognition and text analysis. Large Language Models (LLMs), like BERT, and GPT belong to different families of NLP namely natural language understanding (NLU) and natural language generation (NLG). These advanced models, trained on vast text datasets, specialize in generating coherent and contextually relevant text.
- What are the most popular LLM models?
- The most popular Large Language Models include OpenAI’s GPT series (like GPT-3 and GPT-4), Google’s BERT and its variants, T5 (Text-To-Text Transfer Transformer), PaEL2, Llama2, etc. These models stand out for their advanced text understanding and generation capabilities.
- What’s the relationship between transfer learning & LLM?
- Transfer learning is integral to Large Language Models (LLMs). LLMs are initially trained on vast, general datasets, gaining broad language understanding. This knowledge is then “transferred” and fine-tuned for specific tasks or domains, enhancing their performance in specialized applications without needing training from scratch, thereby saving time and resources.
- Is ChatGPT a large language model?
- ChatGPT is built on top of a large language model such as GPT-3.5, GPT-4, etc. These LLMs belong to the GPT (Generative Pre-trained Transformer) family, specifically designed as an auto-regressive language model. This means it predicts the next word in a sentence based on the words that precede it. ChatGPT utilizes vast datasets and complex algorithms to understand and generate responses that closely mimic human language, showcasing the advanced capabilities of auto-regressive large language models in natural language generation (NLG).
- How do we evaluate different LLMs?
- Check out this HELM page from Stanford – Holistic Evaluation of Language Models (HELM) (stanford.edu). You would find evaluation metrics of different variants of LLMs such as GPT-4, Claude, Mixtral, PaLM-2, Llama 2, etc. The related HELM paper can be found on Arxiv – HELM.
There is another leaderboard maintained on huggingface to track, rank, and evaluate open LLMs and chatbots – OpenLLM Leaderboard.
- Check out this HELM page from Stanford – Holistic Evaluation of Language Models (HELM) (stanford.edu). You would find evaluation metrics of different variants of LLMs such as GPT-4, Claude, Mixtral, PaLM-2, Llama 2, etc. The related HELM paper can be found on Arxiv – HELM.
- What are the parameters in large language models?
Conclusion
Large language models (LLMs) are powerful tools for processing natural language data quickly and accurately with minimal human intervention. These LLMs can be used for a variety of tasks such as text generation, sentiment analysis, question-answering systems, automatic summarization, machine translation, document classification, and more. With the LLMs’ ability to quickly and accurately process vast amounts of text data, they have become invaluable tools for various applications across different industries. NLP researchers and specialists should familiarize themselves with large language models if they want to stay ahead in this rapidly evolving field. All in all, large language models play an important role in NLP because they enable machines to better understand natural language and generate more accurate results when processing text. By utilizing AI technology such as deep learning neural networks, these models can quickly analyze vast amounts of data and deliver highly accurate outcomes that can be used for various applications in different industries.
- Questions to Ask When Thinking Like a Product Leader - July 3, 2025
- Three Approaches to Creating AI Agents: Code Examples - June 27, 2025
- What is Embodied AI? Explained with Examples - May 11, 2025
[…] learning is at the heart of these large language models (LLMs), enabling GPT models to understand/comprehend and create text that closely resembles human speech, […]
[…] you ever wondered how to seamlessly integrate the vast knowledge of Large Language Models (LLMs) with the specificity of external databases? As the world of machine learning continues to […]
Here is a directory of large language models – https://www.predera.com/blog/exploring-llm-models-a-deep-dive-into-licenses-parameters-companies-and-repositories
Thank you for sharing the information.
Very well written. Thank you Ajitesh. Keep up the great work!
Thank you Somnath
[…] Role of Large Language Models (LLMs): LLMs, with their capabilities to understand and process language, can significantly enhance the […]
[…] of the common challenges faced with the deployment of large language models (LLMs) while achieving low-latency completions (inferences) is the size of the LLMs. The size of LLM throws […]