Category Archives: Large Language Models
Attention Mechanism in Transformers: Examples
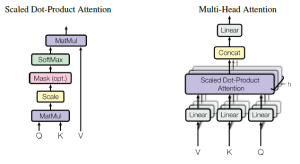
Last updated: 1st Feb, 2024 The attention mechanism allows the model to focus on relevant words or phrases when performing NLP tasks such as translating a sentence or answering a question. It is a critical component in transformers, a type of neural network architecture used in NLP tasks such as those related to LLMs. In this blog, we will delve into different aspects of the attention mechanism (also called an attention head), common approaches (such as self-attention, cross attention, etc.) to calculating and implementing attention, and learn the concepts with the help of real-world examples. You can get good details in this book: Generative Deep Learning by David Foster. You …
Large Language Models (LLMs): Types, Examples
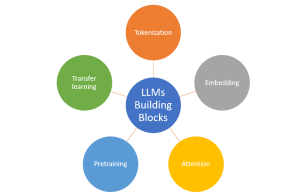
Last updated: 31st Jan, 2024 Large language models (LLMs), being the key pillar of generative AI, have been gaining traction in the world of natural language processing (NLP) due to their ability to process massive amounts of text and generate accurate results related to predicting the next word in a sentence, given all the previous words. These different LLM models are trained on a large or broad corpus of text datasets, which contain hundreds of millions to billions of words. LLMs, as they are known, rely on complex algorithms including transformer architectures that shift through large datasets and recognize patterns at the word level. This data helps the LLMs better understand …
LLM Optimization for Inference – Techniques, Examples
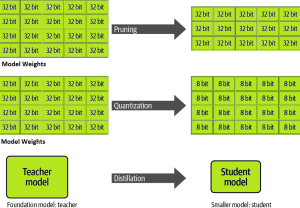
One of the common challenges faced with the deployment of large language models (LLMs) while achieving low-latency completions (inferences) is the size of the LLMs. The size of LLM throws challenges in terms of compute, storage, and memory requirements. And, the solution to this is to optimize the LLM deployment by taking advantage of model compression techniques that aim to reduce the size of the model. In this blog, we will look into three different optimization techniques namely pruning, quantization, and distillation along with their examples. These techniques help model load quickly while enabling reduced latency during LLM inference. They reduce the resource requirements for the compute, storage, and memory. …
LLM Training & GPU Memory Requirements: Examples
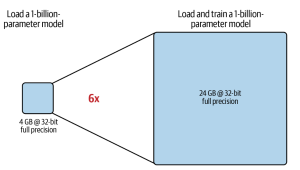
As data scientists and MLOps Engineers, you must have come across the challenges related to managing GPU requirements for training and deploying large language models (LLMs). In this blog, we will delve deep into the intricacies of GPU memory demands when dealing with LLMs. We’ll learn with the help of various examples to better understand how GPU memory impacts the performance and feasibility of training these LLMs. Whether you’re planning to train a foundation (pre-trained) model or fine-tuning an existing model, the insights are aimed to guide you through the crucial considerations of GPU memory allocation. Greater details can be found in this book: Generative AI on AWS. Understanding GPU …
Instruction Fine-tuning LLM Explained with Examples
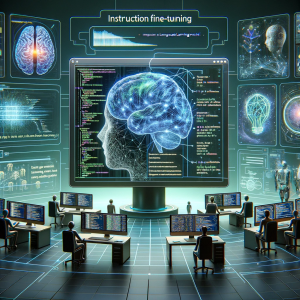
A pre-trained or foundation model is further trained (or fine-tuned) with instructions datasets to help them learn about your specific data and perform humanlike tasks. These models are called instruction fine-tuning LLMs. In this blog, we will learn about the concepts and different examples of instruction fine-tuning models. You might want to check out this book to learn more: Generative AI on AWS. What are Instruction fine-tuning LLMs? Instruction fine-tuning LLMs, also called chat or instruct models, are created by training pre-trained models with different types of instructions. Instruction fine-tuning can be defined as a type of supervised machine learning that improves the foundation model by continuously comparing the model’s …
Distributed LLM Training & DDP, FSDP Patterns: Examples
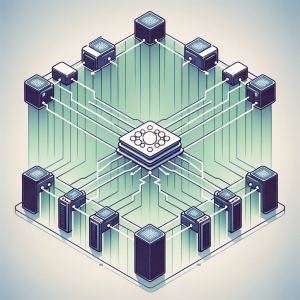
Training large language models (LLMs) like GPT-4 requires the use of distributed computing patterns as there is a need to work with vast amounts of data while training with LLMs having multi-billion parameters vis-a-vis limited GPU support (NVIDIA A100 with 80 GB currently) for LLM training. In this blog, we will delve deep into some of the most important distributed LLM training patterns such as distributed data parallel (DDP) and Fully sharded data parallel (FSDP). The primary difference between these patterns is based on how the model is split or sharded across GPUs in the system. You might want to check out greater details in this book: Generative AI on …
Attention Mechanism Workflow & Transformer: Examples
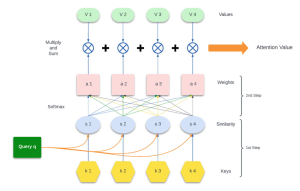
The attention mechanism workflow in the context of transformers in NLP, is a process that enables the model to dynamically focus on certain parts of the input data when performing a task such as machine translation, language understanding, text summarization, etc. Large language models, such as those based on the transformer architecture, rely on attention mechanisms to understand the context of words in a sentence and perform tasks as mentioned earlier. This mechanism selectively weights the significance of different parts of the input. This mechanism is essential for handling sequential data where the importance of each element may vary depending on the context. In this blog, we will learn about …
NLP: Different Types of Language Models – Examples
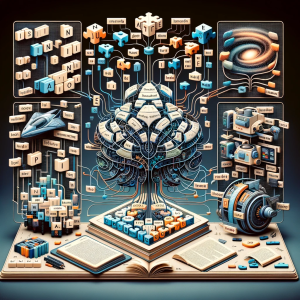
Have you ever wondered how your smartphone seems to know exactly what you’re going to type next? Or how virtual assistants like Alexa and Siri understand and respond to your queries with such precision? The magic is NLP language models. In this blog, we will explore the diverse types of language models in NLP that have evolved over time, each with its unique capabilities and applications. From the simplicity of N-gram models, which predict text based on preceding words, to the sophisticated neural network-based models like RNNs, LSTMs, and the groundbreaking large language models using Transformers, we will learn about the intricacies of these models, examples of real-world applications and …
Large Language Models (LLMs) & Semantic Search: Examples
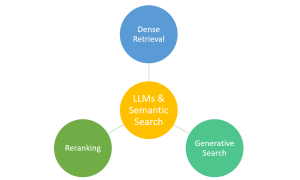
Have you ever marveled at how typing a few words into a search engine yields exactly the information you’re looking for from the vast expanse of the web? This is largely thanks to the advancements in semantic search, bolstered by technologies like Large Language Models (LLMs). Semantic search, which focuses on understanding the intent and contextual meaning behind queries, benefits from LLMs to provide more accurate and relevant results. However, it’s important to note that traditional search engines also rely on a sophisticated mix of algorithms, indexing, and ranking systems. LLMs complement these systems by enhancing their ability to interpret complex queries, making your search experience more intuitive and effective. …
I found it very helpful. However the differences are not too understandable for me