Author Archives: Ajitesh Kumar
Loss Function vs Cost Function vs Objective Function: Examples
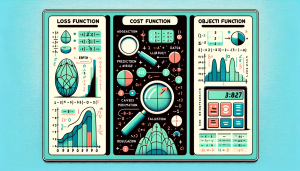
Last updated: 19th April, 2024 Among the terminologies used in training machine learning models, the concepts of loss function, cost function, and objective function often cause a fair amount of confusion, especially for aspiring data scientists and practitioners in the early stages of their careers. The reason for this confusion isn’t unfounded, as these terms are similar / closely related, often used interchangeably, and yet, they are different and serve distinct purposes in the realm of machine learning algorithms. Understanding the differences and specific roles of loss function, cost function, and objective function is more than a mere exercise in academic rigor. By grasping these concepts, data scientists can make …
6 Game-Changing Features of ChatGPT’s Latest Upgrade
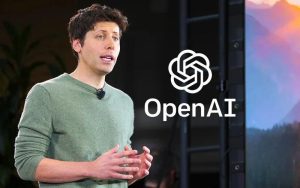
OpenAI has once again set the tech world abuzz with its latest enhancement to ChatGPT, making it a lot easier to use. With a clear focus on user-friendliness and accessibility, this update marks a significant leap forward. Here are the updates on the latest features: Ease of Access: No Sign-Up Required The sign-up barrier has been eliminated, allowing instant access to its ChatGPT. This ensures that access to ChatGPT is just a click away for anyone curious enough to explore it. Customizable Creativity: Choose an Image Style The integration of DALL·E GPT into ChatGPT now includes an option to choose from various image styles, adding a layer of personalization to …
Self-Prediction vs Contrastive Learning: Examples
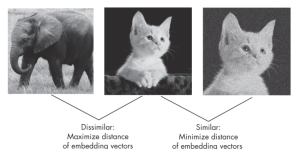
In the dynamic realm of AI, where labeled data is often scarce and costly, self-supervised learning helps unlock new machine learning use cases by harnessing the inherent structure of data for enhanced understanding without reliance on extensive labeled datasets as in the case of supervised learning. Simply speaking, self-supervised learning, at its core, is about teaching models to learn from the data itself, turning unlabeled data into a rich source of learning. There are two distinct methodologies used in self-supervised learning. They are the self-prediction method and contrastive learning method. In this blog, we will learn about their concepts and differences with the help of examples. What is the Self-Prediction …
Free IBM Data Sciences Courses on Coursera
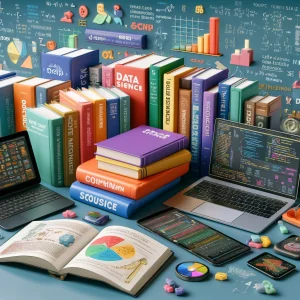
In the rapidly evolving fields of Data Science and Artificial Intelligence, staying ahead means continually learning and adapting. In this blog, there is a list of around 20 free data science-related courses from IBM available on coursera.org that can help data science enthusiasts master different domains in AI / Data Science / Machine Learning. This list includes courses related to the core technical skills and knowledge needed to excel in these innovative fields. Foundational Knowledge: Understanding the essence of Data Science lays the groundwork for a successful career in this field. A solid foundation helps you grasp complex concepts easily and contributes to better decision-making, problem-solving, and the capacity to …
Self-Supervised Learning vs Transfer Learning: Examples
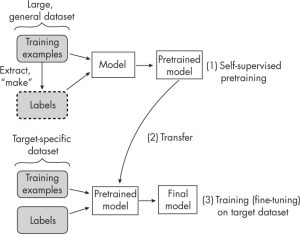
Last updated: 3rd March, 2024 Understanding the difference between self-supervised learning and transfer learning, along with their practical applications, is crucial for any data scientist looking to optimize model performance and efficiency. Self-supervised learning and transfer learning are two pivotal techniques in machine learning, each with its unique approach to leveraging data for model training. Transfer learning capitalizes on a model pre-trained on a broad dataset with diverse categories, to serve as a foundational model for a more specialized task. his method relies on labeled data, often requiring significant human effort to label. Self-supervised learning, in contrast, pre-trains models using unlabeled data, creatively generating its labels from the inherent structure …
OKRs vs KPIs vs KRAs: Differences and Examples
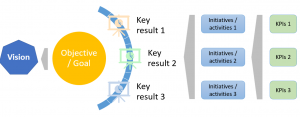
Last updated: 21st Feb, 2024 The difference between OKRs , KPIs, and KRAs is often confused, but the concept is a great way to measure the progress toward achieving your business objectives. As business analysts, product managers, and project or team leaders, it is important to understand the concepts of OKRs, KPIs, & KRAs and what’s the differences between them. In this blog post, we will discuss OKR vs KPI vs KRAs and how they can be used for setting goals/objectives and measuring different aspects of your team’s and organization’s performance in achieving those goals. We’ll also go over real-world examples so you can get a better understanding of how these metrics …
CEP vs Traditional Database Examples
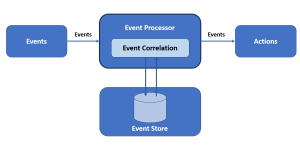
In this blog, we will learn about the differences between complex event processing (CEP) and traditional database querying with the help of examples. We will learn about how these two methodologies tackle data to extract meaningful insights but in fundamentally different ways. In complex event processing, data flows dynamically which is then matched with pre-defined patterns thereby generating insights in real-time. Traditional Database Querying In a conventional database querying scenario, the data is stored first, and then queries are run against this stored data to find patterns or retrieve information. This process is reactive, in that the query is formulated based on a need to find out something specific about …
Attention Mechanism in Transformers: Examples
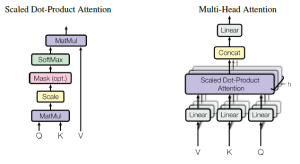
Last updated: 1st Feb, 2024 The attention mechanism allows the model to focus on relevant words or phrases when performing NLP tasks such as translating a sentence or answering a question. It is a critical component in transformers, a type of neural network architecture used in NLP tasks such as those related to LLMs. In this blog, we will delve into different aspects of the attention mechanism (also called an attention head), common approaches (such as self-attention, cross attention, etc.) to calculating and implementing attention, and learn the concepts with the help of real-world examples. You can get good details in this book: Generative Deep Learning by David Foster. You …
NLP Tokenization in Machine Learning: Python Examples
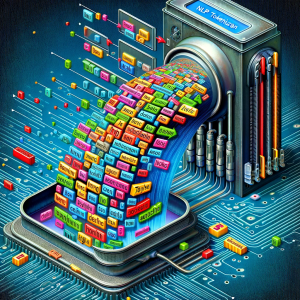
Last updated: 1st Feb, 2024 Tokenization is a fundamental step in Natural Language Processing (NLP) where text is broken down into smaller units called tokens. These tokens can be words, characters, or subwords, and this process is crucial for preparing text data for further analysis like parsing or text generation. Tokenization plays a crucial role in training machine learning models, particularly Large Language Models (LLMs) like GPT (Generative Pre-trained Transformer) series, BERT (Bidirectional Encoder Representations from Transformers), and others. Tokenization is often the first step in preparing text data for machine learning. LLMs use tokenization as an essential data preprocessing step. Advanced tokenization techniques (like those used in BERT) allow …
Large Language Models (LLMs): Types, Examples
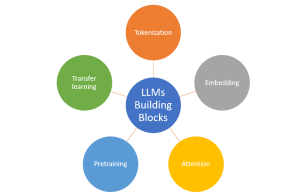
Last updated: 31st Jan, 2024 Large language models (LLMs), being the key pillar of generative AI, have been gaining traction in the world of natural language processing (NLP) due to their ability to process massive amounts of text and generate accurate results related to predicting the next word in a sentence, given all the previous words. These different LLM models are trained on a large or broad corpus of text datasets, which contain hundreds of millions to billions of words. LLMs, as they are known, rely on complex algorithms including transformer architectures that shift through large datasets and recognize patterns at the word level. This data helps the LLMs better understand …
Amazon (AWS) Machine Learning / AI Services List
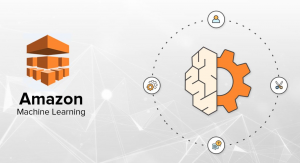
Last updated: 30th Jan, 2024 Amazon Web Services (AWS) is a cloud computing platform that offers machine learning as one of its many services. AWS has been around for over 10 years and has helped data scientists leverage the Amazon AWS cloud to train machine learning models. AWS provides an easy-to-use interface that helps data scientists build, test, and deploy their machine learning models with ease. AWS also provides access to pre-trained machine learning models so you can start building your model without having to spend time training it first! You can get greater details on AWS machine learning services, data science use cases, and other aspects in this book – …
Problem, Symptoms & Root Cause Analysis (RCA) Examples
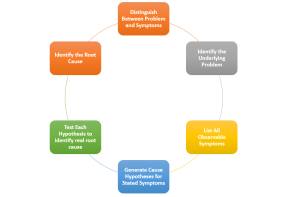
Last updated: 30th Jan, 2024 Have you found yourself stuck in a cycle of solving the same or similar problems over and over again? Ever wondered why some solutions seem to only offer a temporary fix? Have you wondered if you have identified the correct problem or if you are trying to fix one of the symptoms? The key lies in your understanding of how we define problem statements, associated symptoms, root causes, and approach to problem-solving, which is fundamentally rooted in analytical thinking and critical thinking. What exactly is the difference between a problem and its symptoms? And why is it crucial to conduct a root cause analysis to …
LLM Optimization for Inference – Techniques, Examples
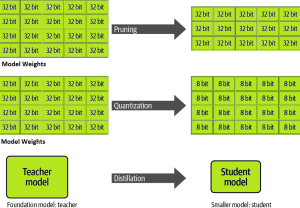
One of the common challenges faced with the deployment of large language models (LLMs) while achieving low-latency completions (inferences) is the size of the LLMs. The size of LLM throws challenges in terms of compute, storage, and memory requirements. And, the solution to this is to optimize the LLM deployment by taking advantage of model compression techniques that aim to reduce the size of the model. In this blog, we will look into three different optimization techniques namely pruning, quantization, and distillation along with their examples. These techniques help model load quickly while enabling reduced latency during LLM inference. They reduce the resource requirements for the compute, storage, and memory. …
How is ChatGPT Trained to Generate Desired Responses?
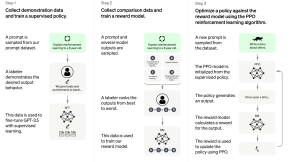
Last updated: 27th Jan, 2024 Training an AI / Machine Learning model as sophisticated as the one used by ChatGPT involves a multi-step process that fine-tunes its ability to understand and generate human-like text. Let’s break down the ChatGPT training process into three primary steps. Note that OpenAI has not published any specific paper on this. However, the reference has been provided on this page – Introducing ChatGPT. Fine-tuning Base Model with Supervised Learning The first phase starts with collecting demonstration data. Here, prompts are taken from a dataset, and human labelers provide the desired output behavior, which essentially sets the standard for the AI’s responses. For example, if the …
AI-Ready Data Explained with Examples
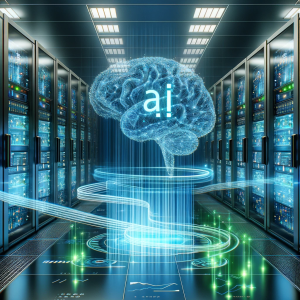
AI-ready data usually refers to data that has been prepared in such a way that it can be effectively used for training artificial intelligence (AI) and generative AI models. In this blog, we will learn about what are the most common attributes of AI-ready data. The following are the top most 5 attributes that AI-ready data would need to have. Data must be: Check out this Gartner paper for further details – We Shape AI, AI shapes us.
First Principles Thinking Explained with Examples
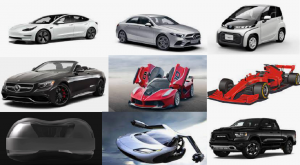
Last updated: 23rd Jan, 2024 Can innovation be taught and learned methodically? Can there be an innovation playbook using which, given a need to create a thing, product, or solve a complex problem, a set of well-defined steps be followed? How has Elon Musk been super successful time and again in creating game-changing innovative products that created tremendous value for end-users and society at large? The answers to these questions can be found with a reasoning technique called first principles thinking. The first principles thinking is often associated with Elon Musk, who uses this approach to come up with his business ideas, create innovative product designs, and build winning products …
I found it very helpful. However the differences are not too understandable for me