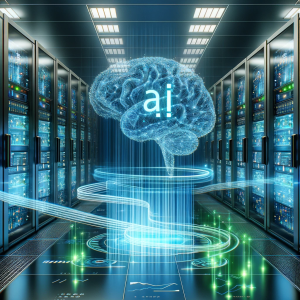
AI-ready data usually refers to data that has been prepared in such a way that it can be effectively used for training artificial intelligence (AI) and generative AI models. In this blog, we will learn about what are the most common attributes of AI-ready data. The following are the top most 5 attributes that AI-ready data would need to have. Data must be:
- Accurate: Data must be correct and reliable. For AI models, having accurate data is critical because models learn from the data they are fed. Inaccuracies in data can lead to incorrect AI models and thus unreliable outputs.
- Enriched: Enriching data typically involves adding context, metadata, or supplementary information to raw data to make it more useful for training AI models.
Tagging is a common method of enriching data which involves labeling the data with informative tags that can be used to identify certain characteristics or features of the data points. By tagging data with accurate and detailed labels, you can improve the training of machine learning models. For instance, in image recognition, each image could be tagged with labels describing the objects in the image (like ‘dog’, ‘car’, ‘tree’, etc.). This allows the AI model to learn from these tags and more accurately recognize and classify images. Tagging can also make it easier to search and filter through large datasets. - Unbiased: AI relies on data to make decisions, and if that data is biased, the AI’s decisions will be too. Unbiased data is essential to create fair and ethical AI systems. The goal is to have “wide data” apart from “big data“. Wide Data, on the other hand, emphasizes the breadth and diversity of the data. It’s not just about having a vast amount of data but also having data that captures a broad spectrum of scenarios, behaviors, and characteristics.
One of the most common techniques to get unbiased data is to ensure that the data is collected from a variety of sources can help minimize the risk of bias. Make sure that the data does not overrepresent or underrepresent any particular group of people. Ensure to set up an ongoing process to monitor for and correct data biases as they are identified.
As per Gartner, By 2025, it is predicted that 70% of organizations will be compelled to shift their focus from big data to small and wide data, providing more context for analytics - Secure: Data must be protected from unauthorized access and breaches. Security is crucial to maintaining the integrity of the data and the trustworthiness of the AI systems that use it.
An organization wanting to use large language models (LLMs) should plan to license and use an LLM without pumping the organization’s data onto the internet or into someone else’s training data. When LLMs are used on-premises or in a private cloud, the organization can control the retention and deletion policies of the data, ensuring that sensitive information is not stored longer than necessary. The organization has more control over when and how the LLM is updated or modified, allowing for better planning and risk management.
There is a guarantee that the data remains within the legal jurisdiction of the organization, which is particularly important for compliance with certain regulations that dictate where and how data should be stored and processed. - Governed: This refers to the management of data in a way that ensures it meets all legal and ethical standards. Governed data is compliant with data protection laws, privacy standards, and other regulatory requirements. Data governance efforts must not be just about mitigating risks but also about enabling the realization of data’s full potential.
In many large enterprises, data governance is often siloed, with fragmented efforts and diverse groups of stakeholders who have different goals, responsibilities, and perspectives regarding the risks, challenges, and potential benefits associated with data and analytics. It is of utmost importance to bring together various stakeholders, including IT, data science teams, legal, compliance, and business units, to align on data outcomes, understand each other’s data concerns, and collaborate on data governance efforts.
Check out this Gartner paper for further details – We Shape AI, AI shapes us.
- Completion Model vs Chat Model: Python Examples - June 30, 2024
- LLM Hosting Strategy, Options & Cost: Examples - June 30, 2024
- Application Architecture for LLM Applications: Examples - June 25, 2024
Leave a Reply