Tag Archives: machine learning
CNN Basic Architecture for Classification & Segmentation
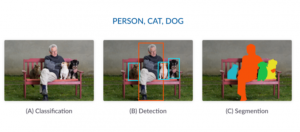
As data scientists, we are constantly exploring new techniques and algorithms to improve the accuracy and efficiency of our models. When it comes to image-related problems, convolutional neural networks (CNNs) are an essential tool in our arsenal. CNNs have proven to be highly effective for tasks such as image classification and segmentation, and have even been used in cutting-edge applications such as self-driving cars and medical imaging. Convolutional neural networks (CNNs) are deep neural networks that have the capability to classify and segment images. CNNs can be trained using supervised or unsupervised machine learning methods, depending on what you want them to do. CNN architectures for classification and segmentation include …
Keras: Multilayer Perceptron (MLP) Example
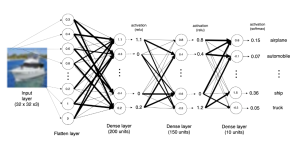
Artificial Neural Networks (ANN) have emerged as a powerful tool in machine learning, and Multilayer Perceptron (MLP) is a popular type of ANN that is widely used in various domains such as image recognition, natural language processing, and predictive analytics. Keras is a high-level API that makes it easy to build and train neural networks, including MLPs. In this blog, we will dive into the world of MLPs and explore how to build and train an MLP model using Keras. We will build a simple MLP model using Keras and train it on a dataset. We will explain different aspects of training MLP model using Keras. By the end of …
Neural Network & Multi-layer Perceptron Examples
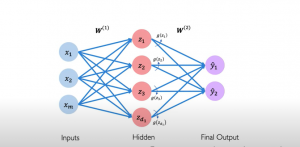
Neural networks are an important part of machine learning, so it is essential to understand how they work. A neural network is a computer system that has been modeled based on a biological neural network comprising neurons connected with each other. It can be built to solve machine learning tasks, like classification and regression problems. The perceptron algorithm is a representation of how neural networks work. The artificial neurons were first proposed by Frank Rosenblatt in 1957 as models for the human brain’s perception mechanism. This post will explain the basics of neural networks with a perceptron example. You will understand how a neural network is built using perceptrons. This …
Generative vs Discriminative Models: Examples
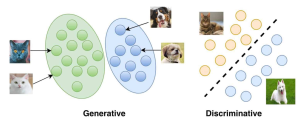
The field of machine learning is rapidly evolving, and with it, the concepts and techniques that are used to develop models that can learn from data. Among these concepts, generative and discriminative models are two widely used approaches in the field. Generative models learn the joint probability distribution of the input features and output labels, whereas discriminative models learn the conditional probability distribution of the output labels given the input features. While both models have their strengths and weaknesses, understanding the differences between them is crucial to developing effective machine learning systems. Real-world problems such as speech recognition, natural language processing, and computer vision, require complex solutions that are able …
Sequence to Sequence Models: Types, Examples
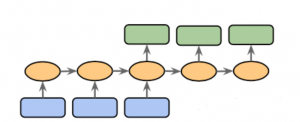
Sequence to sequence (Seq2Seq) modeling is a powerful machine learning technique that has revolutionized the way we do natural language processing (NLP). It allows us to process input sequences of varying lengths and produce output sequences of varying lengths, making it particularly useful for tasks such as language translation, speech recognition, and chatbot development. Sequence to sequence modeling also provides a great foundation for creating text summarizers, question answering systems, sentiment analysis systems, and more. With its wide range of applications, learning about sequence to sequence modeling concepts is essential for anyone who wants to work in the field of natural language processing. This blog post will discuss types of …
Machine Learning Bias Explained with Examples
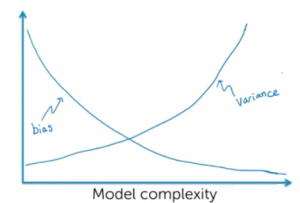
In the artificial intelligence (AI) / machine learning (ML) powered world where predictive models have started getting used more often in decision-making areas, the primary concerns of policy makers, auditors and end users have been to make sure that these systems using the models are not making biased/unfair decisions based on model predictions (intentional or unintentional discrimination). Imagine industries such as banking, insurance, and employment where models are used as solutions to decision-making problems such as shortlisting candidates for interviews, approving loans/credits, deciding insurance premiums etc. How harmful it could be to the end users as these decisions may impact their livelihood based on biased predictions made by the model, thereby, …
Ensemble Methods in Machine Learning: Examples
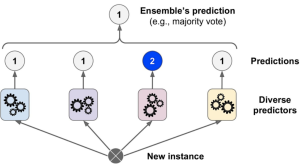
Machine learning models are often trained with a variety of different methods in order to create a more accurate prediction. Ensemble methods are one way to do this, and involve combining the predictions of several different models in order to get a more accurate result. When different models make predictions together, it can help create a more accurate result. Data scientists should care about this because it can help them create models that are more accurate. In this article, we will look at some of the common ensemble methods used in machine learning. Data scientists should care about this because it can help them create models that are more accurate. …
CART Decision Tree Python Example
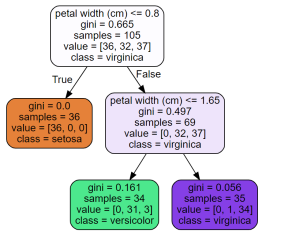
The Classification and Regression Tree (CART) is a supervised machine learning algorithm used for classification, regression. In this blog, we will discuss what CART decision tree is, how it works, and provide a detailed example of its implementation using Python. What is CART & How does it work? CART stands for Classification And Regression Tree. It is a type of decision tree which can be used for both classification and regression tasks based on non-parametric supervised learning method. The following represents the algorithm steps. First and foremost, the data is split into training and test set. Take a feature K and split the training data set into two subsets based on …
Decision Tree Concepts, Examples, Interview Questions
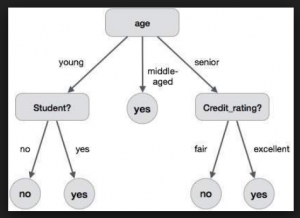
Decision tree is one of the most commonly used machine learning algorithms which can be used for solving both classification and regression problems. It is very simple to understand and use. Here is a lighter one representing how decision trees and related algorithms (random forest etc) are agile enough for usage. In this post, you will learn about some of the following in relation to machine learning algorithm – decision trees vis-a-vis one of the popular C5.0 algorithm used to build a decision tree for classification. In another post, we shall also be looking at CART methodology for building a decision tree model for classification. The post also presents a …
Account Receivables Use Cases for Machine Learning / AI
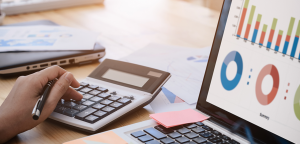
Account receivables (AR) account for a significant portion of total assets and revenue. However, the account receivable process is typically handled manually by accountants or finance staff. This can lead to inefficiencies when it comes to identifying account issues and resolving them quickly. In addition, there are opportunities of leveraging data-driven decision making in different areas related to account receivables. In this blog post, you will learn about account receivables analytics use cases and how AI/machine learning and deep learning techniques can be used to streamline account receivable processes. For product managers and data scientists, this post will prove to be useful to understand different machine learning use cases related …
Bayesian thinking & Real-life Examples
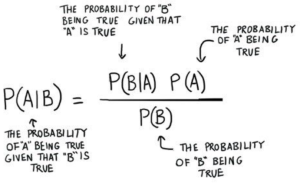
Bayesian thinking is a powerful way of looking at the world, and it can be useful in many real-life situations. Bayesian thinking involves using prior knowledge to make more accurate predictions about future events or outcomes. It is based on the Bayes theorem, which states that the probability of an event occurring is determined by its prior probability combined with new information as it becomes available. It is important for data scientists to learn about Bayesian thinking because it can help them make accurate predictions and draw more meaningful insights from data. In this blog post, we will discuss Bayesian thinking and provide some examples from everyday life to illustrate …
True Error vs Sample Error: Difference
Understanding the differences between true error and sample error is an important aspect of data science. In this blog post, we will be exploring the difference between these two common features of statistical inference. We’ll discuss what they are and how they differ from each other, as well as provide some examples of real-world scenarios where an understanding of both is important. By the end, you should have a better grasp of the differences between true error and sample error. In case you are a data scientist, you will want to understand the concept behind the true error and sample error. These concepts are key to understand for evaluating a …
Confidence Intervals Formula, Examples
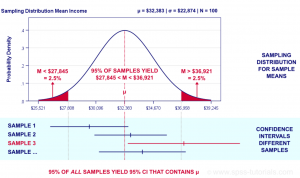
In this post, you will learn about the statistics concepts of confidence intervals in relation to machine learning models with the help of an example and Python code examples. You will learn about how to interpret confidence intervals, what are formulas for confidence intervals with the help of examples. When you get a hypothesis function by training a machine learning classification model, you evaluate the hypothesis/model by calculating the classification error. The classification error is calculated on the sample of the data used for training the model. However, does this classification error for the sample (sample error) also represent (same as) the classification error of the hypothesis/model for the entire …
Types of Probability Distributions: Codes, Examples
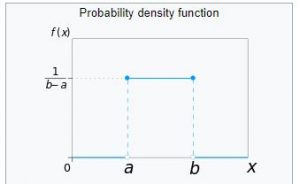
In this post, you will learn the definition of 25 different types of probability distributions. Probability distributions play an important role in statistics and in many other fields, such as economics, engineering, and finance. They are used to model all sorts of real-world phenomena, from the weather to stock market prices. Before we get into understanding different types of probability distributions, let’s understand some fundamentals. If you are a data scientist, you would like to go through these distributions. This page could also be seen as a cheat sheet for probability distributions. What are Probability Distributions? Probability distributions are a way of describing how likely it is for a random …
AI Product Manager Interview Questions
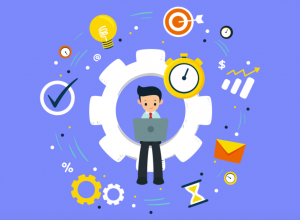
AI has become such an integral part of our lives that it is important to hire professionals who can help create AI / machine learning products that will be used by many people. These AI product manager interview questions will give you insight into your product manager candidate’s experience, skills, and industry knowledge so that you can get prepared in a better manner before appearing for your next interview as an AI product manager. Check out a detailed interview questions and answers with greater focus on machine learning topics. Before getting into the list of interview questions, lets understand what can be the job description of an AI product manager. …
Instance-based vs Model-based Learning: Differences
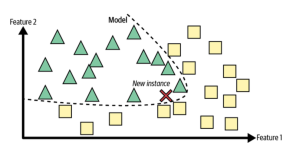
Machine learning is a field of artificial intelligence that deals with giving machines the ability to learn without being explicitly programmed. In this context, instance-based learning and model-based learning are two different approaches used to create machine learning models. While both approaches can be effective, they also have distinct differences that must be taken into account when building a machine learning system. Let’s explore the differences between these two types of machine learning. What is instance-based learning & how does it work? Instance-based learning (also known as memory-based learning or lazy learning) involves memorizing training data in order to make predictions about future data points. This approach doesn’t require any …
I found it very helpful. However the differences are not too understandable for me