Category Archives: Machine Learning
Python Pickle Security Issues / Risk
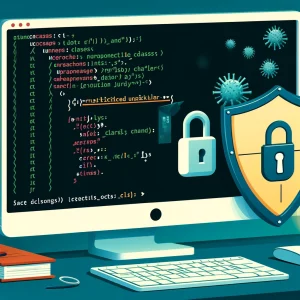
Suppose your machine learning model is serialized as a Python pickle file and later loaded for making predictions. In that case, you need to be aware of security risks/issues associated with loading the Python Pickle file. Security Issue related to Python Pickle The Python pickle module is a powerful tool for serializing and deserializing Python object structures. However, its very power is also what makes it a potential security risk. When data is “pickled,” it is converted into a byte stream that can be written to a file or transmitted over a network. “Unpickling” this data reconstructs the original object in memory. The danger lies in the fact that unpickling …
Pricing Analytics in Banking: Strategies, Examples
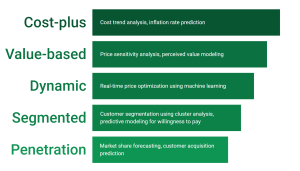
Last updated: 15th May, 2024 Have you ever wondered how your bank decides what to charge you for its services? Or, perhaps how do banks arrive at the pricing (fees, rates, and charges) associated with various banking products? If you’re a product manager, data analyst, or data scientist in the banking industry, you might be aware that these pricing decisions are far from arbitrary. Rather, these pricing decisions are made based on one or more frameworks while leveraging data analytics. They result from intricate pricing strategies, driven by an extensive array of data and sophisticated analytics. In this blog, we will learn about some popular pricing strategies banks execute to …
Machine Learning Lifecycle: Data to Deployment Example
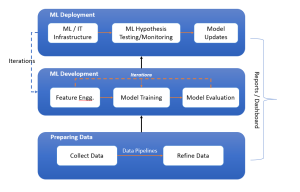
Last updated: 12th May 2024 In this blog, we get an overview of the machine learning lifecycle, from initial data handling to the deployment and iterative improvement of ML models. You might want to check out this book for greater insights into machine learning (ML) concepts – Machine Learning Interviews. The following is the diagram representing the machine learning lifecycle while showcasing three key stages such as preparing data, ML development, and ML deployment. These three stages are explained later in this blog. Stage A: Preparing Data Preparing data for training machine learning models involves collecting data, constructing data pipelines for preprocessing, and refining the data to prepare it for …
Autoencoder vs Variational Autoencoder (VAE): Differences, Example
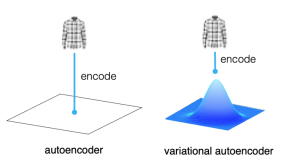
Last updated: 12th May, 2024 In the world of generative AI models, autoencoders (AE) and variational autoencoders (VAEs) have emerged as powerful unsupervised learning techniques for data representation, compression, and generation. While they share some similarities, these algorithms have unique properties and applications that distinguish them. This blog post aims to help machine learning / deep learning enthusiasts understand these two methods, their key differences, and how they can be utilized in various data-driven tasks. We will learn about autoencoders and VAEs, understanding their core components, working mechanisms, and common use cases. We will also try and understand their differences in terms of architecture, objectives, and outcomes. What are Autoencoders? …
Feature Engineering in Machine Learning: Python Examples
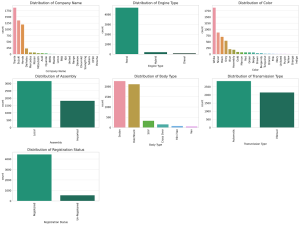
Last updated: 3rd May, 2024 Have you ever wondered why some machine learning models perform exceptionally well while others don’t? Could the magic ingredient be something other than the algorithm itself? The answer is often “Yes,” and the magic ingredient is feature engineering. Good feature engineering can make or break a model. In this blog, we will demystify various techniques for feature engineering, including feature extraction, interaction features, encoding categorical variables, feature scaling, and feature selection. To demonstrate these methods, we’ll use a real-world dataset containing car sales data. This dataset includes a variety of features such as ‘Company Name’, ‘Model Name’, ‘Price’, ‘Model Year’, ‘Mileage’, and more. Through this …
Feature Selection vs Feature Extraction: Machine Learning
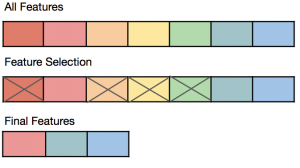
Last updated: 2nd May, 2024 The success of machine learning models often depends on the quality of the features used to train them. This is where the concepts of feature extraction and feature selection come in. In this blog post, we’ll explore the difference between feature selection and feature extraction, two key techniques used as part of feature engineering in machine learning to optimize feature sets for better model performance. Both feature selection and feature extraction are used for dimensionality reduction which is key to reducing model complexity given that higher model complexity often results in overfitting. We’ll provide examples of how they can be applied in real-world scenarios. If …
Model Selection by Evaluating Bias & Variance: Example
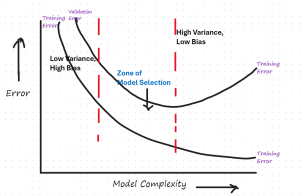
When working on a machine learning project, one of the key challenges faced by data scientists/machine learning engineers is to select the most appropriate model that generalizes well to unseen datasets. To achieve the best generalization on unseen data, the model’s bias and variance need to be balanced. In this post, we’ll explore how to visualize and interpret the trade-off between bias and variance using a residual error vs. model complexity plot. We’ll use a specific plot to guide our discussion. The following is the residual error vs model complexity plot that would need to be drawn for evaluating the model bias vs variance for model selection. We will learn …
Bias-Variance Trade-off in Machine Learning: Examples
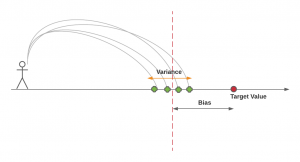
Last updated: 1st May, 2024 The bias-variance trade-off is a fundamental concept in machine learning that presents a challenging dilemma for data scientists. It relates to the problem of simultaneously minimizing two sources of residual error that prevent supervised learning algorithms from generalizing beyond their training data. These two sources of error are related to Bias and Variance. Bias-related errors refer to the error due to overly simplistic machine learning models. Variance-related errors refer to the error due to too much complexity in the models. In this post, you will learn about the concepts of bias & variance in the machine learning (ML) models. You will learn about the tradeoff between bias …
Mean Squared Error vs Cross Entropy Loss Function
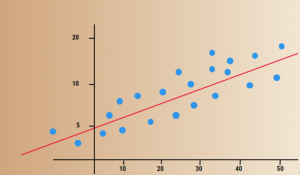
Last updated: 1st May, 2024 As a data scientist, understanding the nuances of various cost functions is critical for building high-performance machine learning models. Choosing the right cost function can significantly impact the performance of your model and determine how well it generalizes to unseen data. In this blog post, we will delve into two widely used cost functions: Mean Squared Error (MSE) and Cross Entropy Loss. By comparing their properties, applications, and trade-offs, we aim to provide you with a solid foundation for selecting the most suitable loss function for your specific problem. Cost functions play a pivotal role in training machine learning models as they quantify the difference …
Cross Entropy Loss Explained with Python Examples
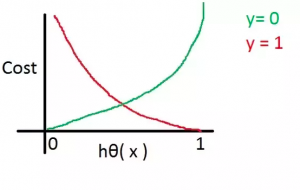
Last updated: 1st May, 2024 In this post, you will learn the concepts related to the cross-entropy loss function along with Python code examples and which machine learning algorithms use the cross-entropy loss function as an objective function for training the models. Cross-entropy loss represents a loss function for models that predict the probability value as output (probability distribution as output). Logistic regression is one such algorithm whose output is a probability distribution. You may want to check out the details on how cross-entropy loss is related to information theory and entropy concepts – Information theory & machine learning: Concepts What’s Cross-Entropy Loss? Cross-entropy loss, also known as negative log-likelihood …
Gradient Descent in Machine Learning: Python Examples
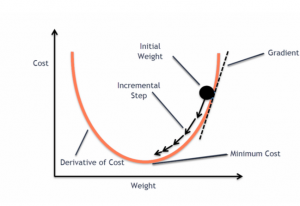
Last updated: 22nd April, 2024 This post will teach you about the gradient descent algorithm and its importance in training machine learning models. For a data scientist, it is of utmost importance to get a good grasp on the concepts of gradient descent algorithm as it is widely used for optimizing/minimizing the objective function / loss function / cost function related to various machine learning models such as regression, neural network, etc. in terms of learning optimal weights/parameters. This algorithm is essential because it underpins many machine learning models, enabling them to learn from data by optimizing their performance. Introduction to Gradient Descent Algorithm The gradient descent algorithm is an optimization …
Loss Function vs Cost Function vs Objective Function: Examples
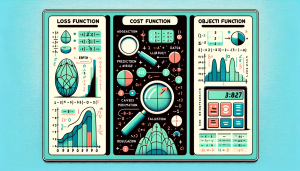
Last updated: 19th April, 2024 Among the terminologies used in training machine learning models, the concepts of loss function, cost function, and objective function often cause a fair amount of confusion, especially for aspiring data scientists and practitioners in the early stages of their careers. The reason for this confusion isn’t unfounded, as these terms are similar / closely related, often used interchangeably, and yet, they are different and serve distinct purposes in the realm of machine learning algorithms. Understanding the differences and specific roles of loss function, cost function, and objective function is more than a mere exercise in academic rigor. By grasping these concepts, data scientists can make …
Self-Prediction vs Contrastive Learning: Examples
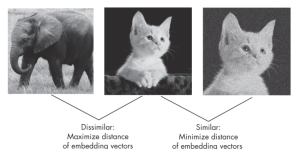
In the dynamic realm of AI, where labeled data is often scarce and costly, self-supervised learning helps unlock new machine learning use cases by harnessing the inherent structure of data for enhanced understanding without reliance on extensive labeled datasets as in the case of supervised learning. Simply speaking, self-supervised learning, at its core, is about teaching models to learn from the data itself, turning unlabeled data into a rich source of learning. There are two distinct methodologies used in self-supervised learning. They are the self-prediction method and contrastive learning method. In this blog, we will learn about their concepts and differences with the help of examples. What is the Self-Prediction …
Free IBM Data Sciences Courses on Coursera
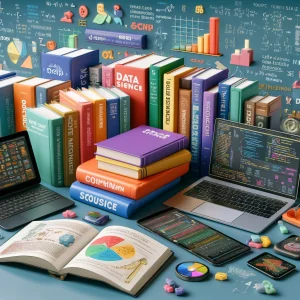
In the rapidly evolving fields of Data Science and Artificial Intelligence, staying ahead means continually learning and adapting. In this blog, there is a list of around 20 free data science-related courses from IBM available on coursera.org that can help data science enthusiasts master different domains in AI / Data Science / Machine Learning. This list includes courses related to the core technical skills and knowledge needed to excel in these innovative fields. Foundational Knowledge: Understanding the essence of Data Science lays the groundwork for a successful career in this field. A solid foundation helps you grasp complex concepts easily and contributes to better decision-making, problem-solving, and the capacity to …
Self-Supervised Learning vs Transfer Learning: Examples
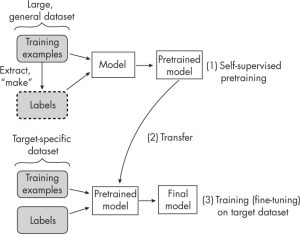
Last updated: 3rd March, 2024 Understanding the difference between self-supervised learning and transfer learning, along with their practical applications, is crucial for any data scientist looking to optimize model performance and efficiency. Self-supervised learning and transfer learning are two pivotal techniques in machine learning, each with its unique approach to leveraging data for model training. Transfer learning capitalizes on a model pre-trained on a broad dataset with diverse categories, to serve as a foundational model for a more specialized task. his method relies on labeled data, often requiring significant human effort to label. Self-supervised learning, in contrast, pre-trains models using unlabeled data, creatively generating its labels from the inherent structure …
NLP Tokenization in Machine Learning: Python Examples
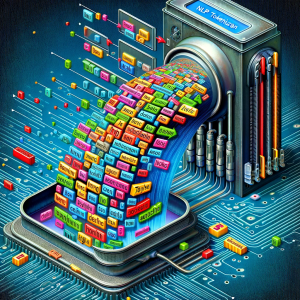
Last updated: 1st Feb, 2024 Tokenization is a fundamental step in Natural Language Processing (NLP) where text is broken down into smaller units called tokens. These tokens can be words, characters, or subwords, and this process is crucial for preparing text data for further analysis like parsing or text generation. Tokenization plays a crucial role in training machine learning models, particularly Large Language Models (LLMs) like GPT (Generative Pre-trained Transformer) series, BERT (Bidirectional Encoder Representations from Transformers), and others. Tokenization is often the first step in preparing text data for machine learning. LLMs use tokenization as an essential data preprocessing step. Advanced tokenization techniques (like those used in BERT) allow …
I found it very helpful. However the differences are not too understandable for me