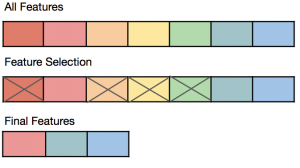
Last updated: 2nd May, 2024
The success of machine learning models often depends on the quality of the features used to train them. This is where the concepts of feature extraction and feature selection come in. In this blog post, we’ll explore the difference between feature selection and feature extraction, two key techniques used as part of feature engineering in machine learning to optimize feature sets for better model performance. Both feature selection and feature extraction are used for dimensionality reduction which is key to reducing model complexity given that higher model complexity often results in overfitting. We’ll provide examples of how they can be applied in real-world scenarios. If you want to improve your machine learning models, understanding the basics of feature selection and feature extraction is essential. So, let’s dive in and explore these concepts in more detail.
Feature Selection Concepts & Techniques
Feature selection is a process in machine learning that involves selecting the most relevant subset of features out of the original features in the dataset, to be used as inputs for training the model. The goal of feature selection is to improve model performance on unseen datasets by reducing the number of irrelevant or redundant features that may introduce high variance into the model, thereby resulting in the model overfitting.
Benefits of feature selection: By selecting only the most important features, the model can focus on the features that have the most positive impact on the model performance, and ignore irrelevant or redundant features that may lead to the overfitting of the model. This can result in faster training times, and improved accuracy on unseen data sets based on reduced generalization error.
Disadvantages of feature selection being ignored: If we don’t adopt feature selection when training a machine learning model, we may encounter several problems.
- Including too many features in the model can lead to the curse of dimensionality, where the model becomes computationally expensive and may struggle to generalize well to new data.
- Including irrelevant or redundant features can introduce noise and, thus, variance into the model, leading to overfitting and reduced performance on new data.
The following represents some of the important feature selection techniques:
Regularization techniques such as L1 norm regularization
L1 norm regularization, also known as Lasso regularization, is a common regularization technique used in feature selection. It works by adding a penalty term that encourages the model to select only the most important features, while reducing the weights of irrelevant or redundant features to zero. L1 norm regularization introduces sparsity into the feature weights, meaning that only a subset of the features have non-zero weights. The other features are effectively ignored by the model, resulting in a form of automatic feature selection. L1 norm regularization can be particularly useful in cases where the dataset contains many features, and some of them are irrelevant or redundant.
Feature importance technique for features selection
Feature importance techniques such as using estimator such as Random Forest algorithm to fit a model and select features based on the value of attribute such as feature_importances_ . The feature_importances_ attribute of the Random Forest estimator can be used to obtain the relative importance of each feature in the dataset. The feature_importances_ attribute of the Random Forest estimator provides a score for each feature in the dataset, indicating how important that feature is for making predictions. These scores are calculated based on the reduction in impurity (e.g., Gini impurity or entropy) achieved by splitting the data on that feature. The feature with the highest score is considered the most important, while features with low scores can be considered less important or even irrelevant. The code below
# Import required libraries
from sklearn.datasets import load_iris
from sklearn.model_selection import train_test_split
from sklearn.ensemble import RandomForestClassifier
# Load the IRIS dataset
iris = load_iris()
# Split data into features (X) and target variable (y)
X = iris.data
y = iris.target
# Split data into training and testing sets
X_train, X_test, y_train, y_test = train_test_split(X, y, test_size=0.3, random_state=42)
# Train the Random Forest classifier
rf = RandomForestClassifier(n_estimators=100, random_state=42)
rf.fit(X_train, y_train)
# Get feature importances
importances = rf.feature_importances_
# Print feature importances
for feature, importance in zip(iris.feature_names, importances):
print(f'{feature}: {importance}')
This is what will get printed.

Greedy search algorithms for features selection
Greedy search algorithms such as some of the following which are useful for algorithms (such as K-nearest neighbours, K-NN) where regularization techniques are not supported.
- Sequential forward selection
- Sequential floating forward selection
- Sequential backward selection
- Sequential floating backward selection
Different types of features selection techniques
According to the utilized training data (labeled, unlabeled, or partially labeled), feature selection methods can be divided into supervised, unsupervised, and semi-supervised models. According to their relationship with learning methods, feature selection methods can be classified into the following:
- Filter methods: The filter model only considers the association between the feature and the class label. Filter methods involves ranking features based on a statistical measure and selecting a subset of the top-ranked features for the model. The filter method is independent of the model and can be used with any machine learning algorithm. The most common statistical measures used for ranking features include some of the following:
- Pearson correlation coefficient
- Chi-squared test
- Wrapper methods: Wrapper methods are a class of feature selection techniques that select subsets of features by evaluating the performance of a machine learning model. Unlike filter methods, wrapper methods use the model’s performance on the training data as a criterion for selecting features. They involve repeatedly training and evaluating a model on different subsets of features, and selecting the subset that achieves the best performance. Wrapper methods have several advantages, including their ability to handle complex interactions between features and to select features that are important for a specific model, rather than for the dataset as a whole. However, they can be computationally expensive and may overfit the training data if the number of features is too large. There are several types of wrapper methods, including some of the following:
- Forward selection
- Backward elimination
- Embedded methods: In embedded method, the features are selected in the training process of learning model, and the feature selection result outputs automatically while the training process is finished. Unlike filter and wrapper methods, embedded methods are built into the algorithm and select the most relevant features during model training. Embedded methods typically involve adding a penalty term to the loss function during model training, which encourages the model to select only the most important features. The penalty term can be based on L1 or L2 regularization, and is used to constrain the weights of the features. Features with low weights are effectively ignored by the model, while features with high weights are considered important for making predictions.
According to the evaluation criterion, feature selection methods can be derived from correlation, Euclidean distance, consistency, dependence and information measures. According to the type of output, feature selection methods can be divided into feature rank (weighting) and subset selection models.
Feature Extraction Concepts & Techniques
Feature extraction is about extracting/deriving information from the original features set to create a new features subspace. The primary idea behind feature extraction is to compress the data with the goal of maintaining most of the relevant information. As with feature selection techniques, these techniques are also used for reducing the number of features from the original features set to reduce model complexity, model overfitting, enhance model computation efficiency and reduce generalization error. The following are different types of feature extraction techniques:
- Principal component analysis (PCA) for unsupervised data compression. Here is a detailed post on feature extraction using PCA with Python example. You will get a good understanding of how PCA can help with finding the directions of maximum variance in high-dimensional data and projects the data onto a new subspace with equal or fewer dimensions than the original one. This is explained with example of identifying Taj Mahal (7th wonder of world) from top view or side view based on dimensions in which there is maximum variance. The diagram below shows the dimensions of maximum variance (PCA1 and PCA2) as a result of PCA.
- Linear discriminant analysis (LDA) as a supervised dimensionality reduction technique for maximizing class separability
- Nonlinear dimensionality reduction via kernel principal component analysis (KPCA)
When to use Feature Selection & Feature Extraction
The key difference between feature selection and feature extraction techniques used for dimensionality reduction is that while the original features are maintained in the case of feature selection algorithms, the feature extraction algorithms transform the data onto a new feature space.
Feature selection techniques can be used if the requirement is to maintain the original features, unlike the feature extraction techniques which derive useful information from data to construct a new feature subspace. Feature selection techniques are used when model explainability is a key requirement.
Feature extraction techniques can be used to improve the predictive performance of the models, especially, in the case of algorithms that don’t support regularization.
Unlike feature selection, feature extraction usually needs to transform the original data to features with strong pattern recognition ability, where the original data can be regarded as features with weak recognition ability.
Quiz – Test your knowledge
Here is a quick quiz you can use to check your knowledge on feature selection vs feature extraction.
[wp_quiz id=”10213″]
Resources
- Questions to Ask When Thinking Like a Product Leader - July 3, 2025
- Three Approaches to Creating AI Agents: Code Examples - June 27, 2025
- What is Embodied AI? Explained with Examples - May 11, 2025
I found it very helpful. However the differences are not too understandable for me