Category Archives: Machine Learning
Amazon (AWS) Machine Learning / AI Services List
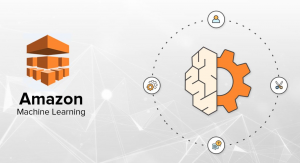
Last updated: 30th Jan, 2024 Amazon Web Services (AWS) is a cloud computing platform that offers machine learning as one of its many services. AWS has been around for over 10 years and has helped data scientists leverage the Amazon AWS cloud to train machine learning models. AWS provides an easy-to-use interface that helps data scientists build, test, and deploy their machine learning models with ease. AWS also provides access to pre-trained machine learning models so you can start building your model without having to spend time training it first! You can get greater details on AWS machine learning services, data science use cases, and other aspects in this book – …
LLM Optimization for Inference – Techniques, Examples
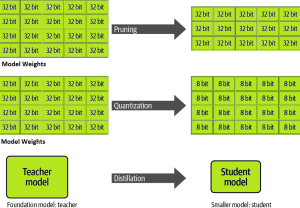
One of the common challenges faced with the deployment of large language models (LLMs) while achieving low-latency completions (inferences) is the size of the LLMs. The size of LLM throws challenges in terms of compute, storage, and memory requirements. And, the solution to this is to optimize the LLM deployment by taking advantage of model compression techniques that aim to reduce the size of the model. In this blog, we will look into three different optimization techniques namely pruning, quantization, and distillation along with their examples. These techniques help model load quickly while enabling reduced latency during LLM inference. They reduce the resource requirements for the compute, storage, and memory. …
How is ChatGPT Trained to Generate Desired Responses?
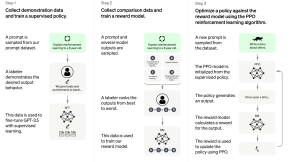
Last updated: 27th Jan, 2024 Training an AI / Machine Learning model as sophisticated as the one used by ChatGPT involves a multi-step process that fine-tunes its ability to understand and generate human-like text. Let’s break down the ChatGPT training process into three primary steps. Note that OpenAI has not published any specific paper on this. However, the reference has been provided on this page – Introducing ChatGPT. Fine-tuning Base Model with Supervised Learning The first phase starts with collecting demonstration data. Here, prompts are taken from a dataset, and human labelers provide the desired output behavior, which essentially sets the standard for the AI’s responses. For example, if the …
Transfer Learning vs Fine Tuning LLMs: Differences
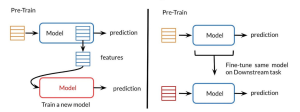
Last updated: 23rd Jan, 2024 Two NLP concepts that are fundamental to large language models (LLMs) are transfer learning and fine-tuning pre-trained LLMs. Rather, true fine-tuning can also be termed as full fine-tuning because transfer learning is also a form of fine-tuning. Despite their interconnected nature, they are distinct methodologies that serve unique purposes when training foundation LLMs to achieve different objectives. In this blog, we will explore the differences between transfer Learning and full fine-tuning, learning about their characteristics and how they come into play in real-world scenarios related to natural language understanding (NLU) and natural language generation (NLG) tasks with the help of examples. We will also learn …
Generalization Errors in Machine Learning: Python Examples
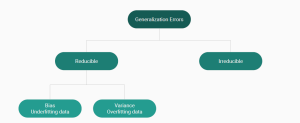
Last updated: 21st Jan, 2024 Machine Learning (ML) models are designed to make predictions or decisions based on data. However, a common challenge, data scientists face when developing these models is ensuring that they generalize well to new, unseen data. Generalization refers to a model’s ability to perform accurately on new, unseen examples after being trained on a limited set of data. When models don’t generalize well, they commit errors. These errors are called generalization errors. In this blog, you will learn about different types of generalization errors, with examples, and walk through a simple Python demonstration to illustrate these concepts. Types of Generalization Errors Generalization errors in machine learning …
Distributed LLM Training & DDP, FSDP Patterns: Examples
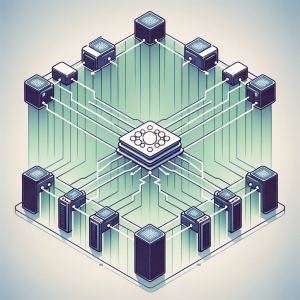
Training large language models (LLMs) like GPT-4 requires the use of distributed computing patterns as there is a need to work with vast amounts of data while training with LLMs having multi-billion parameters vis-a-vis limited GPU support (NVIDIA A100 with 80 GB currently) for LLM training. In this blog, we will delve deep into some of the most important distributed LLM training patterns such as distributed data parallel (DDP) and Fully sharded data parallel (FSDP). The primary difference between these patterns is based on how the model is split or sharded across GPUs in the system. You might want to check out greater details in this book: Generative AI on …
Transformer Architecture Types: Explained with Examples
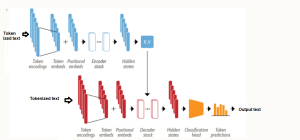
Are you fascinated by the power of deep learning large language models that can generate creative writing, answer complex questions, etc? Ever wondered how these LLMs understand and process human language with such finesse? At the heart of these remarkable achievements lies a machine learning model architecture that has revolutionized the field of Natural Language Processing (NLP) – the Transformer architecture and its types. But what makes Transformer models so special? From encoding sentences into numerical embeddings to employing attention mechanisms that capture the relationships between words, we will dissect different types of Transformer architectures, provide real-world examples, and even dive into the mathematics that governs its operation. Let’s explore …
Blueprint: Deploying Generative AI Applications
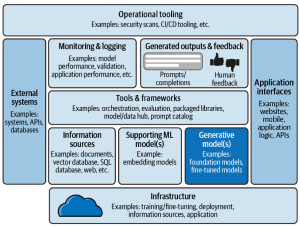
In this blog, we will learn about a comprehensive framework for the deployment of generative AI applications, breaking down the essential components that architects must consider. Learn more about this topic from this book: Generative AI on AWS. The following is a solution / technology architecture that represents a blueprint for deploying generative AI applications. The following is an explanation of the different components of this architectural viewpoint:
BERT vs GPT Models: Differences, Examples
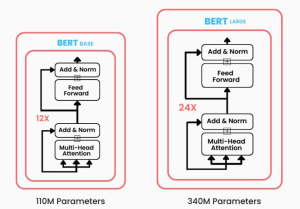
Have you been wondering what sets apart two of the most prominent transformer-based machine learning models in the field of NLP, Bidirectional Encoder Representations from Transformers (BERT) and Generative Pre-trained Transformers (GPT)? While BERT leverages encoder-only transformer architecture, GPT models are based on decoder-only transformer architecture. In this blog, we will delve into the core architecture, training objectives, real-world applications, examples, and more. By exploring these aspects, we’ll learn about the unique strengths and use cases of both BERT and GPT models, providing you with insights that can guide your next LLM-based NLP project or research endeavor. Differences between BERT vs GPT Models BERT, introduced in 2018, marked a significant …
Demystifying Encoder Decoder Architecture & Neural Network
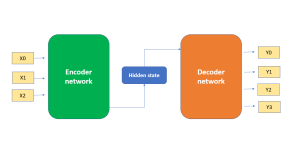
In the field of AI / machine learning, the encoder-decoder architecture is a widely-used framework for developing neural networks that can perform natural language processing (NLP) tasks such as language translation, text summarization, and question-answering systems, etc which require sequence-to-sequence modeling. This architecture involves a two-stage process where the input data is first encoded (using what is called an encoder) into a fixed-length numerical representation, which is then decoded (using a decoder) to produce an output that matches the desired format. In this blog, we will explore the inner workings of the encoder-decoder architecture, how it can be used to solve real-world problems, and some of the latest developments in …
NLP: Different Types of Language Models – Examples
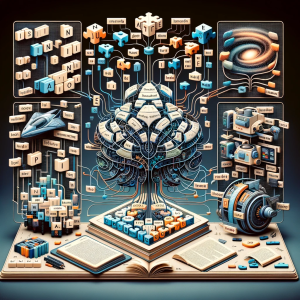
Have you ever wondered how your smartphone seems to know exactly what you’re going to type next? Or how virtual assistants like Alexa and Siri understand and respond to your queries with such precision? The magic is NLP language models. In this blog, we will explore the diverse types of language models in NLP that have evolved over time, each with its unique capabilities and applications. From the simplicity of N-gram models, which predict text based on preceding words, to the sophisticated neural network-based models like RNNs, LSTMs, and the groundbreaking large language models using Transformers, we will learn about the intricacies of these models, examples of real-world applications and …
Bag of Words in NLP & Machine Learning: Examples
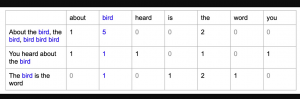
Last updated: 6th Jan, 2024 Most machine learning algorithms require numerical input for training the models. Bag of words (BoW) effectively converts text data into numerical feature vectors, making it compatible with a wide range of machine learning algorithms, from linear classifiers like logistic regression to complex ones like neural networks. In this post, you will learn about the concepts of bag-of-words model and how to train a text classification model using Python Sklearn. Some of the most common text classification problems includes sentiment analysis, spam filtering etc. In these problems, one can apply bag-of-words technique to train machine learning models for text classification. It will be good to understand the …
Cohen Kappa Score Explained: Formula, Example
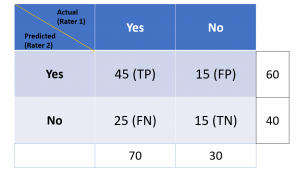
Last updated: 5th Jan, 2024 Cohen’s Kappa Score is a statistic used to measure the performance of machine learning classification models. In this blog post, we will discuss what Cohen’s Kappa Score is and Python code example representing how to calculate Kappa score using Python. We will also provide a code example so that you can see how it works! What is Cohen’s Kappa Score or Coefficient? Cohen’s Kappa Score, also known as the Kappa Coefficient, is a statistical measure of inter-rater agreement for categorical data. Cohen’s Kappa Coefficient is named after statistician Jacob Cohen, who developed the metric in 1960. It is generally used in situations where there …
Validation Techniques for Machine Learning Models: Examples
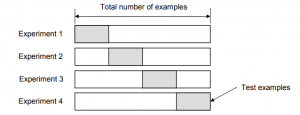
Last updated: 4th Jan, 2024 In the realm of machine learning, the emphasis increasingly shifts towards solving real-world problems with high-quality models. Creating high performant models does not not just depend on raw computational power or theoretical knowledge, but crucially on the ability to systematically conduct and learn from a myriad of different models by trying with hypothesis and related experiments including different algorithms, datasets / features, hyperparameters, etc. This is where the importance of a robust validation strategy and related techniques becomes paramount. Validation techniques, in essence, are the methodologies employed to accurately assess a model’s errors and to gauge how its performance fluctuates with different experiments. The primary …
Machine Learning Definition, Examples, Method, Types
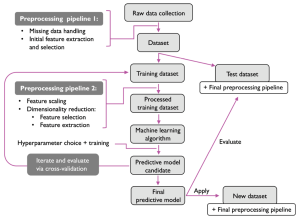
Last updated: 3rd Jan, 2024 Machine learning is a machine’s ability to learn from data. It has been around for decades, but machine learning is now being applied in nearly every industry and job function. In this blog post, we’ll cover a detailed introduction to what is machine learning (ML) including different definitions. We will also learn about different types of machine learning tasks, algorithms, etc along with real-world examples. What is machine learning & how does it work? Definition 1: Simply speaking, machine learning can be defined as an approach to model our beliefs about real-world events. For example, let’s say a person came to a doctor with a …
Machine Learning Models Solution Design: Examples
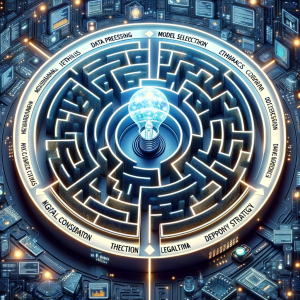
This blog is crafted for data scientists, machine learning (ML) and software engineers, business analysts / product managers, and anyone involved in the ML project lifecycle, aiming to create a reliable solution design and development strategy / plan for successful AI / machine learning project implementation and value realization. The blog revolves around a series of critical solution design questions, meticulously curated to guide teams from the initial conception of a project to its final deployment and beyond. By addressing each of these solution design questions, teams can ensure that they are not only building a model that is technically proficient but also one that aligns seamlessly with business objectives, …
I found it very helpful. However the differences are not too understandable for me