Tag Archives: Deep Learning
Credit Card Fraud Detection & Machine Learning
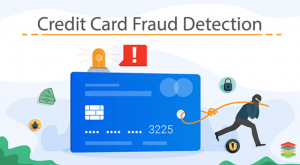
Last updated: 26 Sept, 2024 Credit card fraud detection is a major concern for credit card companies. With credit cards so prevalent in our society, credit card companies must be able to prevent fraud happening with credit card transactions and protect their customers. Machine learning techniques can provide a powerful and effective way of detecting fraud happening with transactions done using credit cards. In this blog post we will discuss ML techniques that data scientists can use to design appropriate fraud detection solutions including algorithms such as Bayesian networks, support vector machines, neural networks and decision trees. What are different types of credit card fraud? The following are different types …
Neural Network Types & Real-life Examples
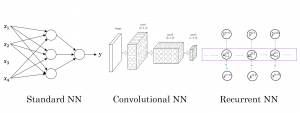
Last updated: 24th Sept, 2024 Neural networks are a powerful tool for data scientists, machine learning engineers, and statisticians. They have revolutionized the field of deep learning and have become an integral part of many real-world applications such as image and speech recognition, natural language processing (NLP), autonomous vehicles, etc. ChatGPT is a classic example how neural network applications has taken world by storm. But what exactly are they and what are their different types? Understanding the different types of neural networks and their real-life examples is crucial. In this blog post, we’ll explore different types of neural networks (ANN, CNN, RNN, LSTM, etc.) , provide real-life examples of how …
Model Parallelism vs Data Parallelism: Examples
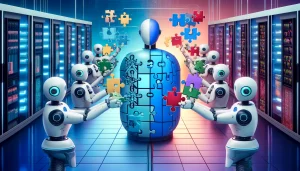
Last updated: 24th August, 2024 Model parallelism and data parallelism are two strategies used to distribute the training of large machine-learning models across multiple computing resources, such as GPUs. They form key categories of multi-GPU training paradigms. These strategies are particularly important in deep learning, where models and datasets can be very large. What’s Data Parallelism? In data parallelism, we break down the data into small batches. Each GPU works on one batch of data at a time. It calculates two things: the loss, which tells us how far off our model’s predictions are from the actual outcomes, and the loss gradients, which guide us on how to adjust the …
Self-Supervised Learning: Concepts, Examples
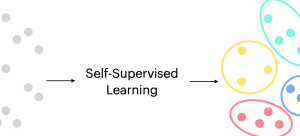
Last updated: 20th August, 2024 Self-supervised learning is an approach to training machine learning models primarily for large corpus of unlabeled dataset. It has gained significant traction due to its effectiveness in various applications related to text and image. Self-supervised learning differs from supervised learning, where models are trained using labeled data, and unsupervised learning, where models are trained using unlabeled data without any pre-defined objectives. Instead, self-supervised learning defines pretext tasks as training models to extract useful features from the data that can be later fine-tuned for specific downstream tasks. The potential of self-supervised learning has already been demonstrated in many real-world applications, such as image classification, natural language …
Autoencoder vs Variational Autoencoder (VAE): Differences, Example
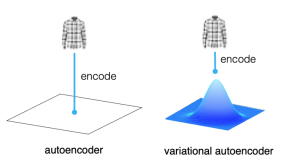
Last updated: 12th May, 2024 In the world of generative AI models, autoencoders (AE) and variational autoencoders (VAEs) have emerged as powerful unsupervised learning techniques for data representation, compression, and generation. While they share some similarities, these algorithms have unique properties and applications that distinguish them. This blog post aims to help machine learning / deep learning enthusiasts understand these two methods, their key differences, and how they can be utilized in various data-driven tasks. We will learn about autoencoders and VAEs, understanding their core components, working mechanisms, and common use cases. We will also try and understand their differences in terms of architecture, objectives, and outcomes. What are Autoencoders? …
Transfer Learning vs Fine Tuning LLMs: Differences
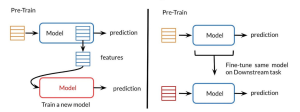
Last updated: 23rd Jan, 2024 Two NLP concepts that are fundamental to large language models (LLMs) are transfer learning and fine-tuning pre-trained LLMs. Rather, true fine-tuning can also be termed as full fine-tuning because transfer learning is also a form of fine-tuning. Despite their interconnected nature, they are distinct methodologies that serve unique purposes when training foundation LLMs to achieve different objectives. In this blog, we will explore the differences between transfer Learning and full fine-tuning, learning about their characteristics and how they come into play in real-world scenarios related to natural language understanding (NLU) and natural language generation (NLG) tasks with the help of examples. We will also learn …
Transformer Architecture in Deep Learning: Examples
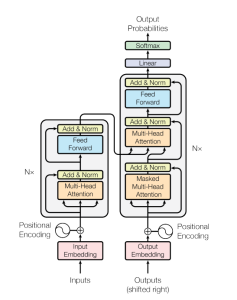
The Transformer model architecture, introduced by Vaswani et al. in 2017, is a deep learning model that has revolutionized the field of natural language processing (NLP) giving rise to large language models (LLMs) such as BERT, GPT, T5, etc. In this blog, we will learn about the details of transformer model architecture with the help of examples and references from the mother paper – Attention is All You Need. Transformer Block – Core Building Block of Transformer Model Architecture Before getting to understand the details of transformer model architecture, let’s understand the key building block termed transformer block. The core building block of the Transformer architecture consists of multi-head attention …
Transformer Architecture Types: Explained with Examples
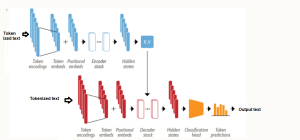
Are you fascinated by the power of deep learning large language models that can generate creative writing, answer complex questions, etc? Ever wondered how these LLMs understand and process human language with such finesse? At the heart of these remarkable achievements lies a machine learning model architecture that has revolutionized the field of Natural Language Processing (NLP) – the Transformer architecture and its types. But what makes Transformer models so special? From encoding sentences into numerical embeddings to employing attention mechanisms that capture the relationships between words, we will dissect different types of Transformer architectures, provide real-world examples, and even dive into the mathematics that governs its operation. Let’s explore …
Pre-trained Models Explained with Examples
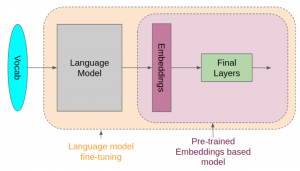
NLP has been around for decades, but it has recently seen an explosion in popularity due to pre-trained models (PTMs), also termed foundation models. This blog post will introduce you to different types of pre-trained (a.k.a. foundation) machine learning models and discuss their usage in real-world examples. Before we get into looking at different types of pre-trained models in NLP, let’s understand the concepts related to pre-trained models. What are Pre-trained Models? Pre-trained models (PTMs) are very large and complex neural network-based deep learning models, such as transformers, that consist of billions of parameters (a.k.a. weights) and have been trained on very large datasets to perform specific NLP tasks. The …
BERT vs GPT Models: Differences, Examples
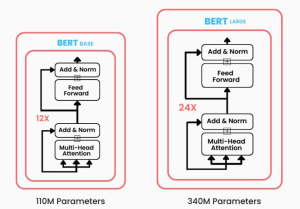
Have you been wondering what sets apart two of the most prominent transformer-based machine learning models in the field of NLP, Bidirectional Encoder Representations from Transformers (BERT) and Generative Pre-trained Transformers (GPT)? While BERT leverages encoder-only transformer architecture, GPT models are based on decoder-only transformer architecture. In this blog, we will delve into the core architecture, training objectives, real-world applications, examples, and more. By exploring these aspects, we’ll learn about the unique strengths and use cases of both BERT and GPT models, providing you with insights that can guide your next LLM-based NLP project or research endeavor. Differences between BERT vs GPT Models BERT, introduced in 2018, marked a significant …
Demystifying Encoder Decoder Architecture & Neural Network
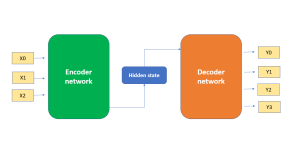
In the field of AI / machine learning, the encoder-decoder architecture is a widely-used framework for developing neural networks that can perform natural language processing (NLP) tasks such as language translation, text summarization, and question-answering systems, etc which require sequence-to-sequence modeling. This architecture involves a two-stage process where the input data is first encoded (using what is called an encoder) into a fixed-length numerical representation, which is then decoded (using a decoder) to produce an output that matches the desired format. In this blog, we will explore the inner workings of the encoder-decoder architecture, how it can be used to solve real-world problems, and some of the latest developments in …
Large Language Models (LLMs) & Semantic Search: Examples
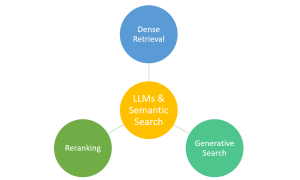
Have you ever marveled at how typing a few words into a search engine yields exactly the information you’re looking for from the vast expanse of the web? This is largely thanks to the advancements in semantic search, bolstered by technologies like Large Language Models (LLMs). Semantic search, which focuses on understanding the intent and contextual meaning behind queries, benefits from LLMs to provide more accurate and relevant results. However, it’s important to note that traditional search engines also rely on a sophisticated mix of algorithms, indexing, and ranking systems. LLMs complement these systems by enhancing their ability to interpret complex queries, making your search experience more intuitive and effective. …
Different Types of CNN Architectures Explained: Examples
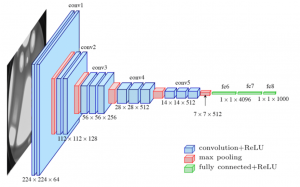
Last updated: 4th Dec, 2023. In the fast-paced world of computer vision and image processing, the problem of image classification consistently stands out: the ability to effectively recognize and classify images. As we continue to digitize and automate our world, the demand for systems that can understand and interpret visual data is growing at an unprecedented rate. The challenge is not just about recognizing images – it’s about doing so accurately and efficiently. Traditional machine learning methods often fall short, struggling to handle the complexity and high dimensionality of image data. This is where Convolutional Neural Networks (CNNs) comes to rescue. And, there are different types of CNN architectures based …
Activation Functions in Neural Networks: Concepts, Examples
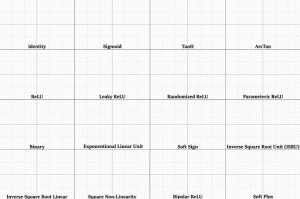
Last updated: 24th Nov, 2023 The activation functions are critical to understanding neural networks. There are many activation functions available for data scientists to choose from, when training neural networks. So, it can be difficult to choose which activation function will work best for their needs. In this blog post, we look at different activation functions and provide examples of when they should be used in different types of neural networks. If you are starting on deep learning and wanted to know about different types of activation functions, you may want to bookmark this page for quicker access in the future. What are activation functions in neural networks? In a …
Encoder Only Transformer Models Quiz / Q&A
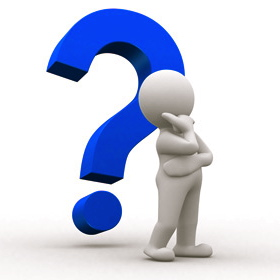
Are you intrigued by the revolutionary world of transformer architectures? Have you ever wondered how encoder-only transformer models like BERT, ELECTRA, or DeBERTa have reshaped the landscape of Natural Language Processing (NLP)? The rapid advancement of machine learning has led to the creation of numerous transformer architectures, each with unique features, applications, and underlying mechanics. Whether you’re a data scientist, machine learning engineer, generative AI enthusiast, or a student eager to deepen your understanding, this quiz offers an engaging and informative way to assess your knowledge and sharpen your skills. It would also help you prepare for your interviews on this topic. Encoder-only transformer models have become a cornerstone in …
Encoder-only Transformer Models: Examples
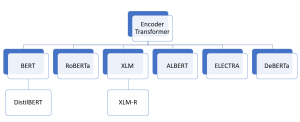
How can machines accurately classify text into categories? What enables them to recognize specific entities like names, locations, or dates within a sea of words? How is it possible for a computer to comprehend and respond to complex human questions? These remarkable capabilities are now a reality, thanks to encoder-only transformer architectures like BERT. From text classification and Named Entity Recognition (NER) to question answering and more, these models have revolutionized the way we interact with and process language. In the realm of AI and machine learning, encoder-only transformer models like BERT, DistilBERT, RoBERTa, and others have emerged as game-changing innovations. These models not only facilitate a deeper understanding of …
I found it very helpful. However the differences are not too understandable for me