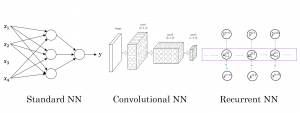
Last updated: 24th Sept, 2024
Neural networks are a powerful tool for data scientists, machine learning engineers, and statisticians. They have revolutionized the field of deep learning and have become an integral part of many real-world applications such as image and speech recognition, natural language processing (NLP), autonomous vehicles, etc. ChatGPT is a classic example how neural network applications has taken world by storm. But what exactly are they and what are their different types? Understanding the different types of neural networks and their real-life examples is crucial.
In this blog post, we’ll explore different types of neural networks (ANN, CNN, RNN, LSTM, etc.) , provide real-life examples of how they’re used. By the end of this post, you’ll have a better understanding of how neural networks work and how they can be used to solve complex problems.
Before jumping into examples, you may want to check out some of my following posts on neural networks:
- Deep Learning Explained Simply in Layman Terms
- Neural network explained with perceptron example
- Perceptron explained with Python example
Also, lets understand some terminologies which will later used in the examples.
What is a Neural Network?
Neural networks are composed of layers of large number of interconnected processing nodes or neurons, and they learn by adjusting the weights of the connections between neurons. Neural network models mimic the workings of the human brain. Neural networks with multiple layers of neurons are called as deep neural networks which represent deep learning. Deep neural networks are found to be capable of recognizing patterns that are too difficult for humans to discern.
The following is a list of different types of problems which can be tackled using deep neural networks:
- Voice recognition
- Image recognition
- Speech recognition
- Language translation
- Pattern recognition
- Anomaly detection
In order to use neural networks effectively, there are a few key requirements that must be met.
- The dataset must be sufficiently large enough, diverse and representative to provide the neural network with enough information to learn from and generalize to unseen data in an accurate manner. The more diverse and representative the data is, the better the network will perform.
- For supervised learning problems, the data must be well-labeled and organized so that the neural network can accurately identify patterns. Poorly labeled data can lead to incorrect learning and poor model performance.
- The data must be of high quality, free of biases, outliers or errors. Cleaning and preprocessing steps, such as normalization, handling missing values, and removing noise, are critical to ensuring the neural network can learn effectively.
- Appropriate neural network architecture must be designed in terms of types of neural network (e.g., CNNs for image data, RNNs for time series, etc.), number of layers, number of nodes in these layers, etc.
- Appropriate training context: The training algorithm should be appropriate including stochastic gradient descent or backpropagation, appropriate selection of hyperparameters including learning rate, batch size, etc.
- Sufficient Training Time and Computational Resources: The neural network must be given enough time to train on the data before it is deployed. Computation resources, including memory (RAM/VRAM) and processing power (CPUs/GPUs/TPUs), play a significant role in determining the speed and feasibility of training. Adequate resources allow the network to iterate through enough data efficiently.
- Regularization and Overfitting Prevention: Techniques such as dropout, L2 regularization, and early stopping can help prevent overfitting, especially when working with deep or large neural networks.
Neural networks can be slow to train and require a large amount of training data. They can also be difficult to interpret and sometimes make errors that are difficult to understand. Despite these limitations, neural networks are a widely-used technique for machine learning and artificial intelligence.
What are different types of Neural Networks?
The following are different types of neural networks:
- Standard artificial neural network (ANN): Standard deep ANN are neural networks with multiple hidden layers. Standard artificial neural networks are composed of a number of interconnected processing nodes, or neurons, that communicate with each other through synapses. In a standard neural network, each neuron (node) receives input from several other neurons and produces an output that is passed to other neurons in the network. The picture below represents the same.
The strength of the connections between neurons (nodes), known as synaptic weights, determines how much influence one neuron (node) has over another. Standard neural networks are designed to learn by adjusting the weights in response to input data. Standard neural networks are trained using a technique called backpropagation. Backpropagation involves adjusting the weights of the connections between the nodes based on how well the network performs on a training set of data. As a result, they are capable of performing complex tasks such as pattern recognition and predictions. - Convolutional neural network (CNN): CNNs are a type of neural network that are well-suited for image classification tasks. CNNs are composed of a series of convolutional layers, which extract features from images, and pooling layers, which down sample the feature maps. In other words, a convolutional layer applies a set of filters to an input image, which results in a set of feature maps that represent different patterns in the image and a pooling layer is used to reduce the dimensionality of the output from the convolutional layer by down sampling the feature maps. CNNs work by convolving an image with a series of filters which are designed to detect one or more specific features in the image. The output of the CNN is then fed into a fully connected layer, which produces the final classification. The first layer of the network usually consists of a convolutional layer, which applies a set of learnable filters to the input image to extract features. Feature maps are fed into the pooling layer which reduces the spatial dimensions (width and height) of the feature maps while retaining their depth (number of channels). The output of the convolutional layers is flattened into a one-dimensional vector, which is then passed to the fully connected layers.
CNNs can learn to detect complex patterns in images, and have been used for a variety of tasks such as object detection and classification. CNNs are also used for facial recognition and identifying people in pictures and videos. In addition, CNNs have been used to create self-driving cars. CNNs are also used in medical imaging applications. For example, CNNs can be used to identify disease symptoms in X-rays and CT scans. Finally, CNNs are used in natural language processing applications. - Recurrent neural network (RNN): RNNs are deep neural networks that has the ability to store information from previous computations and passes it forward so as to work upon this data in a sequential manner. They are a type of neural network where the output of the previous timestep is used as input for the current timestep along with the new input. This creates a relationship between the elements in the sequence, allowing the RNN to learn dependencies between them. This makes RNNs well-suited for tasks such as speech recognition and machine translation, where understanding the context is crucial. Check out my blog on how RNN can be used for sequence to sequence modeling. RNNs can also be used for time series prediction, such as stock market movements or weather patterns. In recent years, RNNs have achieved significant success on a number of challenging tasks. However, training RNNs can be difficult, due to the vanishing gradient problem. This is when the error signal becomes progressively weaker as it is propagated back through the network, making it difficult for the RNN to learn from its mistakes.
- Long short-term memory (LSTM): LSTMs are deep neural networks, which have a mechanism to store the information for long periods. This allows it to learn from experiences that span over many time steps. LSTMs are a type of artificial neural network used for processing sequential data. Unlike traditional neural networks, LSTMs can remember long-term dependencies, making them well-suited for tasks such as machine translation and speech recognition. LSTMs are also used in many other applications, such as predicting stock prices and generating music. One of the key benefits of LSTMs is that they can be trained on relatively small datasets. This is because LSTMs are able to learn from context, which allows them to generalize better than other types of neural networks. As a result, LSTMs have become one of the most popular types of neural networks for Natural Language Processing (NLP) applications. LSTM networks are similar to recurrent neural networks (RNN), but they have a forget gate that allows them to forget information that is no longer needed.
- Stacked Autoencoders: Autoencoders are a type of neural network that are used to learn efficient representations of data. A stacked autoencoder is a type of autoencoder where the input is first encoded by one neural network, and then the output of that network is passed as input to another encoder network. By stacking multiple autoencoders on top of each other, it is possible to learn increasingly complex representations of data. This can be beneficial for tasks such as image recognition, where a deep understanding of the data is required in order to make accurate predictions. In addition, stacked autoencoders can be used to initialize the weights of a deep neural network, which can help to improve the performance of the network.
- Variational autoencoders: Variational autoencoders are a type of neural network that is widely used for dimensionality reduction and generative modeling. The key idea behind variational autoencoders is to learn a latent representation of data that is lower dimensional than the input. This is done by training the network to minimize the KL divergence between the latent representation and the input data. Variational autoencoders have been shown to be very effective at learning complex distributions, and they have been used for applications such as image generation and text generation. The difference between variational autoencoder and stacked autoencoders is that stacked autoencoders learn a compressed version of input data while variational autoencoders learns a probability distribution.
Here is a quick diagram representing the most common type of neural networks including ANN, CNN and RNN.
Real world examples of Deep Neural Networks
The following are some of the examples of real world applications built using different types of deep neural networks such as those listed above:
- Housing price prediction: Standard artificial neural network (ANN) can be used for the real estate market. Deep learning approach can also be used to predict the housing prices in a given area, city or country with high accuracy and low risk involved. The input data can be different home features and the output prediction will be pricing estimate. This is a supervised learning problem.
- Whether user will click on advertisement: Standard ANN can be used to predict whether a user will click on an ad or not. The input data is advertisement and user information and the output can be label such as click (1) or not click (0). This is a supervised learning problem.
- Weather prediction: Recurrent neural network (RNN) or LSTM can be used for predicting weather as the data is temporal or sequential and time-series based. As a matter of fact, a custom or hybrid model built using temporal based network algorithms (RNN / LSTM) and CNN can be used.
- Image classification: Real world applications of image classification includes classification of images with humans, objects and scenes. Business applications for image classification include surveillance, medical diagnosis (healthcare), tagging of images, X-ray interpretation, CT-Scans/MRI interpretation and so on. Deep neural networks have the capability to recognize images at a pixel level which is almost impossible for humans. During Covid-19 times, CNN models were used to classify X-ray/CT-scan images in predicting likelihood of a person suffering from Covid. The input data are different images and output represent different labels. The predictions represent probability that the input is same as one of the output labels. This is a supervised learning problem.
- Machine translation: Deep neural networks can be used to translate languages by learning the semantic representation of words in one language and then mapping them into another language’s word-meaning representations. Recurrent Neural Networks (RNNs) can be used for machine translation problems, where information flows sequentially across time steps. Deep NLP is used for Deep Learning applications in Natural Language Processing (NLP), which is also sometimes referred to as Deep Linguistic Analysis. This is a supervised learning problem.
- Speech recognition: Deep neural networks can be used to recognize speech. Deep learning models for Speech Recognition are Deep Neural Networks trained using Deep Learning techniques/algorithms, specifically Deep Feed-Forward NN (FFNN), Deep Recurrent NN (RNN) and LSTM. The input data can be audio and the output data will be text transcript. A key aspect of learning will comprise of supervised learning.
- Face recognition: With the advent of Deep learning, Deep neural networks can be used for face recognition. Deep neural network models offer a viable solution to the problem of image classification and have gained many improvements in accuracy levels which was not possible with other machine learning algorithms/techniques so far. Deep neural networks such as Deep Convolutional Neural Network (DCNN) and Deep Belief Networks (DBN) can be used for face recognition problems in real-world applications. This can be primarily termed as supervised learning problem.
- Autonomous driving: A custom or hybrid neural network architecture comprising of CNN, ANN etc will be required to build a bunch of models which can be used for autonomous driving. The input to these models will be images, radar information (device put on the top of the car) and the label / output will be position of other vehicles, objects etc which will help in deciding the way of safe driving. As like the above problem, a key aspect of learning will be supervised learning.
Deep neural networks are a powerful tool in the world of Deep Learning and Deep Linguistic Analysis. In this blog post, the examples from different industry verticals were provided. In these examples, different types of deep neural networks (such as ANN, CNN, RNN, LSTM etc) have been used successfully to solve difficult real-world or real-life problems. In case, you want to get trained in Deep Learning, Deep Neural Network or Deep Linguistic Analysis, please feel free to reach out.
- Mathematics Topics for Machine Learning Beginners - July 6, 2025
- Questions to Ask When Thinking Like a Product Leader - July 3, 2025
- Three Approaches to Creating AI Agents: Code Examples - June 27, 2025
I found it very helpful. However the differences are not too understandable for me