Tag Archives: Data Science
SVM RBF Kernel Parameters: Python Examples
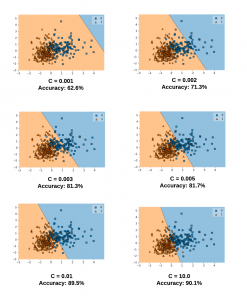
Support vector machines (SVM) are a popular and powerful machine learning technique for classification and regression tasks. SVM models are based on the concept of finding the optimal hyperplane that separates the data into different classes. One of the key features of SVMs is the ability to use different kernel functions to model non-linear relationships between the input variables and the output variable. One such kernel is the radial basis function (RBF) kernel, which is a popular choice for SVMs due to its flexibility and ability to capture complex relationships between the input and output variables. The RBF kernel has two important parameters: gamma and C (also called regularization parameter). …
A/B Testing & Data Science Projects: Examples
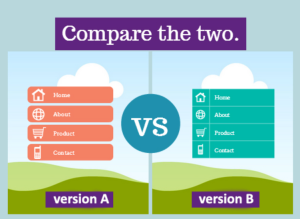
Today, when organization is aiming to become data-driven, it is imperative that their data science and product management teams understand the importance of using A/B testing technique for validating or supporting their decisions. A/B testing is a powerful technique that allows product management and data science teams to test changes to their products or services with a small group of users before implementing them on a larger scale. In data science projects, A/B testing can help measure the impact of machine learning models and the content driven based on the their predictions, and other data-driven changes. This blog explores the principles of A/B testing and its applications in data science. …
Data Science Careers: India’s Job Market & AI Growth
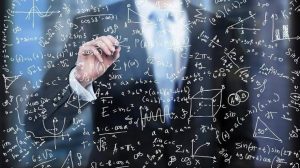
Aspiring data scientists and AI enthusiasts in India have a plethora of opportunities in store, thanks to the country’s booming AI, machine learning (ML), and big data analytics industry. According to a recent report by NASSCOM, India boasts the second-largest talent pool globally in these fields, with a remarkable AI skill penetration score of 3.09 [1]. The nation’s rapid growth in AI talent concentration and scientific publications underscores the immense potential for individuals looking to build a successful data science career in India. As the demand for skilled professionals surges, multiple factors contribute to the thriving industry. The higher-than-average compensation and growth prospects in the field make it an attractive …
Quiz #85: MSE vs R-Squared?
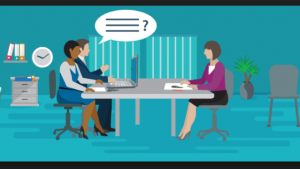
Regression models are an essential tool for data scientists and statisticians to understand the relationship between variables and make predictions about future outcomes. However, evaluating the performance of these models is a crucial step in ensuring their accuracy and reliability. Two commonly used metrics for evaluating regression models are Mean Squared Error (MSE) and R-squared. Understanding when to use each metric and how they differ can greatly improve the quality of your analyses. Check out my related blog on this topic – Mean Squared Error vs R-Squared? Which one to use? To help you test your knowledge on MSE and R-squared (also known as coefficient of determination), we have created …
Data Storytelling Explained with Examples
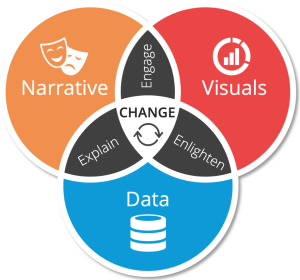
Have you ever told a story to someone, but they just didn’t seem to understand it? They might have been confused about the plot or why the characters acted in certain ways. If this has happened to you before, then you are not alone. Many people struggle with storytelling or rather data storytelling because they do not know how to communicate their data effectively to tell an engaging story. Data storytelling is a powerful tool that can be used to educate, inform or persuade an audience by using different kinds of narration. By using charts, graphs, images and other visuals, data can be made more interesting and engaging. Data storytelling …
Python – Draw Confusion Matrix using Matplotlib
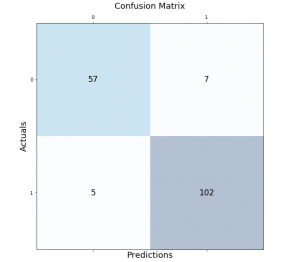
Classification models are a fundamental part of machine learning and are used extensively in various industries. Evaluating the performance of these models is critical in determining their effectiveness and identifying areas for improvement. One of the most common tools used for evaluating classification models is the confusion matrix. It provides a visual representation of the model’s performance by displaying the number of true positives, false positives, true negatives, and false negatives. In this post, we will explore how to create and visualize confusion matrices in Python using Matplotlib. We will walk through the process step-by-step and provide examples that demonstrate the use of Matplotlib in creating clear and concise confusion …
Degree of Freedom in Statistics: Meaning & Examples
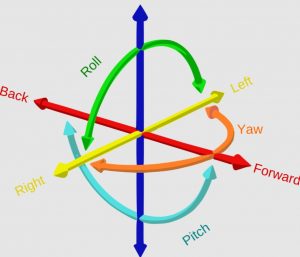
The degree of freedom (DOF) is a term that statisticians use to describe the degree of independence in statistical data. A degree of freedom can be thought of as the number of variables that are free to vary, given one or more constraints. When you have one degree, there is one variable that can be freely changed without affecting the value for any other variable. As a data scientist, it is important to understand the concept of degree of freedom, as it can help you do accurate statistical analysis and validate the results. In this blog, we will explore the meaning of degree of freedom in statistics, its importance in …
Different types of Time-series Forecasting Models
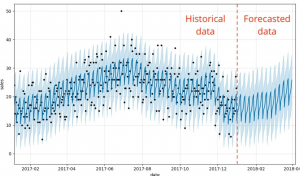
Forecasting is the process of predicting future events based on past and present data. Time-series forecasting is a type of forecasting that predicts future events based on time-stamped data points. Time-series forecasting models are an essential tool for any organization or individual who wants to make informed decisions based on future events or trends. From stock market predictions to weather forecasting, time-series models help us to understand and forecast changes over time. However, with so many different types of models available, it can be challenging to determine which one is best suited for a particular scenario. There are many different types of time-series forecasting models, each with its own strengths …
Support Vector Machine (SVM) Python Example
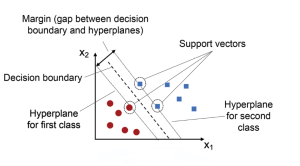
Support Vector Machines (SVMs) are a powerful and versatile machine learning algorithm that has gained widespread popularity among data scientists in recent years. SVMs are widely used for classification, regression, and outlier detection (one-class SVM), and have proven to be highly effective in solving complex problems in various fields, including computer vision (image classification, object detection, etc.), natural language processing (sentiment analysis, text classification, etc.), and bioinformatics (gene expression analysis, protein classification, disease diagnosis, etc.). In this post, you will learn about the concepts of Support Vector Machine (SVM) with the help of Python code example for building a machine learning classification model. We will work with Python Sklearn package for building the …
Fixed vs Random vs Mixed Effects Models – Examples
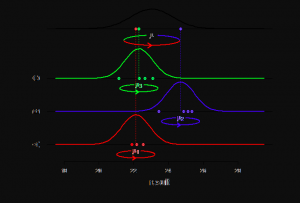
Have you ever wondered what fixed effect, random effect and mixed effects models are? Or, more importantly, how they differ from one another? In this post, you will learn about the concepts of fixed and random effects models along with when to use fixed effects models and when to go for fixed + random effects (mixed) models. The concepts will be explained with examples. As data scientists, you must get a good understanding of these concepts as it would help you build better linear models such as general linear mixed models or generalized linear mixed models (GLMM). What are fixed, random & mixed effects models? First, we will take a real-world example and try and understand …
CNN Basic Architecture for Classification & Segmentation
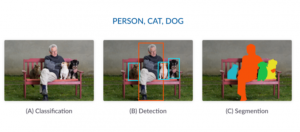
As data scientists, we are constantly exploring new techniques and algorithms to improve the accuracy and efficiency of our models. When it comes to image-related problems, convolutional neural networks (CNNs) are an essential tool in our arsenal. CNNs have proven to be highly effective for tasks such as image classification and segmentation, and have even been used in cutting-edge applications such as self-driving cars and medical imaging. Convolutional neural networks (CNNs) are deep neural networks that have the capability to classify and segment images. CNNs can be trained using supervised or unsupervised machine learning methods, depending on what you want them to do. CNN architectures for classification and segmentation include …
Neural Network & Multi-layer Perceptron Examples
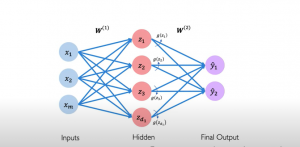
Neural networks are an important part of machine learning, so it is essential to understand how they work. A neural network is a computer system that has been modeled based on a biological neural network comprising neurons connected with each other. It can be built to solve machine learning tasks, like classification and regression problems. The perceptron algorithm is a representation of how neural networks work. The artificial neurons were first proposed by Frank Rosenblatt in 1957 as models for the human brain’s perception mechanism. This post will explain the basics of neural networks with a perceptron example. You will understand how a neural network is built using perceptrons. This …
Positively Skewed Probability Distributions: Examples
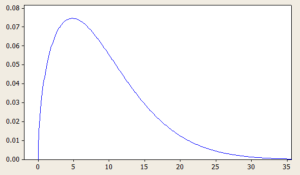
Probability distributions are an essential concept in statistics and data analysis. They describe the likelihood of different outcomes or events occurring and provide valuable insights into the characteristics of a given data set. Skewness is an important aspect of probability distributions that can have a significant impact on data analysis and decision-making. In this blog, we will focus on positively skewed probability distributions and explore some real-life examples where these distributions occur. We will discuss what a positively skewed distribution is, what are its different types with formula and definitions. By the end of this blog, you will have a better understanding of positively skewed distributions and be able to …
Generative vs Discriminative Models: Examples
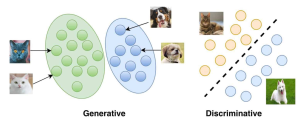
The field of machine learning is rapidly evolving, and with it, the concepts and techniques that are used to develop models that can learn from data. Among these concepts, generative and discriminative models are two widely used approaches in the field. Generative models learn the joint probability distribution of the input features and output labels, whereas discriminative models learn the conditional probability distribution of the output labels given the input features. While both models have their strengths and weaknesses, understanding the differences between them is crucial to developing effective machine learning systems. Real-world problems such as speech recognition, natural language processing, and computer vision, require complex solutions that are able …
Sequence to Sequence Models: Types, Examples
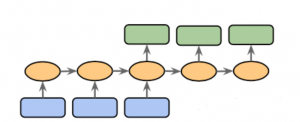
Sequence to sequence (Seq2Seq) modeling is a powerful machine learning technique that has revolutionized the way we do natural language processing (NLP). It allows us to process input sequences of varying lengths and produce output sequences of varying lengths, making it particularly useful for tasks such as language translation, speech recognition, and chatbot development. Sequence to sequence modeling also provides a great foundation for creating text summarizers, question answering systems, sentiment analysis systems, and more. With its wide range of applications, learning about sequence to sequence modeling concepts is essential for anyone who wants to work in the field of natural language processing. This blog post will discuss types of …
Statistics Terminologies Cheat Sheet & Examples
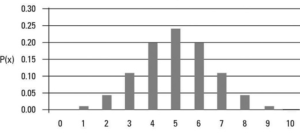
Have you ever felt overwhelmed by all the statistics terminology out there? From sampling distribution to central limit theorem to null hypothesis to p-values to standard deviation, it can be hard to keep up with all the statistical concepts and how they fit into your research. That’s why we created a Statistics Terminologies Cheat Sheet & Examples – a comprehensive guide to help you better understand the essential terms and their use in data analysis. Our cheat sheet covers topics like descriptive statistics, probability, hypothesis testing, and more. And each definition is accompanied by an example to help illuminate the concept even further. Understanding statistics terminology is critical for data …
I found it very helpful. However the differences are not too understandable for me