Author Archives: Ajitesh Kumar
Flutter – Map String Dynamic Code Example
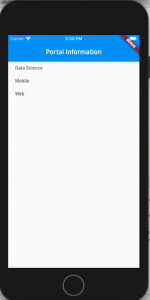
In this post, we will understand the Flutter concepts related to Map<String, Dynamic> with some code example / sample. For greater details, visit Flutter website. Map<String, dynamic> maps a String key with the dynamic value. Since the key is always a String and the value can be of any type, it is kept as dynamic to be on the safer side. It is very useful in reading a JSON object as the JSON object represents a set of key-value pairs where key is of type String while value can be of any type including String, List<String>, List<Object> or List<Map>. Take a look at the following example: Above is an example of JSON object …
SVM Algorithm as Maximum Margin Classifier
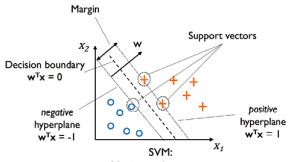
In this post, we will understand the concepts related to SVM (Support Vector Machine) algorithm which is one of the popular machine learning algorithm. SVM algorithm is used for solving classification problems in machine learning. Lets take a 2-dimensional problem space where a point can be classified as one or the other class based on the value of the two dimensions (independent variables, say) X1 and X2. The objective is to find the most optimal line (hyperplane in case of 3 or more dimensions) which could correctly classify the points with most accuracy. In the diagram below, you could find multiple such lines possible. In the above diagram, the objective is to find the …
Security Misconfiguration Example – Upwork
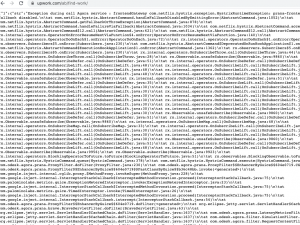
In this post, you will see an example of security misconfiguration which is one of the top 10 security vulnerabilities as per OWASP top 10 security vulnerabilities. Here is what security misconfiguration means? Attackers will often attempt to exploit unpatched flaws or access default accounts, unused pages, unprotected files and directories, etc to gain unauthorized access or knowledge of the system. In this post, you will see the example of unauthorized knowledge of the system. Security Misconfiguration Example This morning, I was checking the Upwork.com when I saw this message when I tried to login. Take a look at exceptions and stack trace. Using the above, I could extract some …
Flutter Interview Questions for Beginners #1
Flutter – When use Stateful vs Stateless Widgets
In this post, you will learn about when to use stateful widgets vs stateless widgets while building a Flutter app. Use Stateful widgets in case of the following scenarios. Note that the StatefulWidget instances themselves are immutable and store their mutable state either in separate State objects that are created by the createState method. When the part of UI which is described using the widget can change dynamically due to some of the following reasons: Due to user-interaction Due to database updates Due to system changes Use Stateless widgets in case of the following scenarios: When the part of UI which is described using the widget depends only on its configuration and the BuildContext in which the …
Flutter – GestureDetector Code Example & Concepts
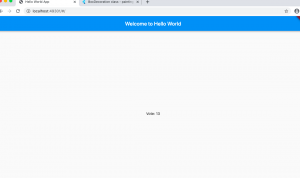
In this post, we will learn about how to use GestureDetector with Stateful widgets in order to record changes to state. The following are key aspects in relation to changing state of the widget based on the users’ gesture. Capture user’s gesture using a widget called as GestureDetector. The following happens as part of GestureDetector widget: Capture events such as onTap, onDoubleTap etc and change the state After the user gesture is captured and the state is changed, the widget is built and replaced with existing widget. The existing widget is flagged as dirty. Here is the code representing the usage of GestureDetector for capturing user gesture, changing the state of the widget and …
Understanding Flutter Widgets with Code Examples
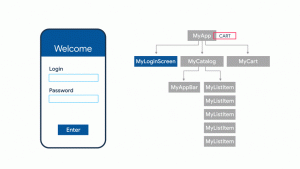
In this post, we will understand some of the following key concepts of Flutter framework. What are widgets? What are stateless widgets? What are stateful widgets? What are Flutter Widgets? A flutter mobile app consists of multiple UIs with each UI built out of one or more widgets. These widgets are nothing but elements of interaction between the app user and the application. Note element of interaction used to describe widget in Wikipedia definition given below. As per the wikipedia, A graphical widget (also graphical control element or control) in a graphical user interface is an element of interaction such as a button or a scroll bar. Controls are software components that a computer user interacts with through direct manipulation …
Flutter Hello World App, Concepts & Code Samples
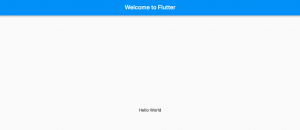
In this post, we will look at quick code samples and related concepts for getting started with Flutter Hello World App. I think the best way to get quickly started without getting bothered about creating Android/iOS simulator or devices is to enable Flutter web app. One can follow instructions in this page related to Flutter web app commands cheat sheet to get started with Flutter web app. Here is the code for Flutter Hello World app. Place this code (replace with existing code created using template project) in lib/main.dart file to get started. Once done, execute the project using command such as following or run from the Android IDE. Basic Concepts for Understanding …
Flutter Web App Commands – Cheatsheet
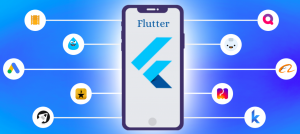
In this post, I will list down some of the key commands (cheatsheet) which can help one build and release web application (app) using Flutter. In order for the following commands to work, follow the steps given below: Install Flutter: Make sure you have installed Flutter. Follow the steps in this page for setting up / installing flutter. Create Flutter Project: Once installed, create a template project for getting started with a project. Use this page for creating a flutter project. Flutter Commands for Web Once flutter is set up and a template project is created, execute the following one-time commands for setting up Flutter web development environment. These commands can be run from anywhere …
Top 5 Data Analytics Methodologies
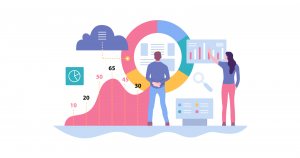
Here is a list of top 5 data analytics methodologies which can be used to solve different business problems and in a way create business value for any organization: Optimization: Simply speaking, an optimization problem consists of maximizing or minimizing a real function by systematically choosing input values (also termed as decision variables) from within an allowed set and computing the value of the function. An optimization problem consists of three things: A. Objective function B. Decision variables C. Constraint functions (this is optional) Linear / Non-linear programming with constrained / unconstrained optimization Linear programming with constrained optimization Objective function and one or more constraint functions are linear with decision variables as continuous variables Linear programming with unconstrained optimization Objective function …
Contract Management Use Cases for Machine Learning
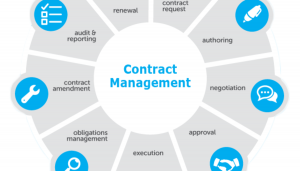
This post briefly represent the contract management use cases which could be solved using machine learning / data science. These use cases can also be termed as predictive analytics use cases. This can be useful for procurement business functions in any manufacturing companies which require to procure raw materials from different suppliers across different geographic locations. The following are some of the examples of industry where these use cases and related machine learning techniques can be useful. Pharmaceutical Airlines Food Transport Key Analytics Questions One must understand the business value which could be created using predictive analytics use cases listed later in this post. One must remember that one must start with questions …
Different Types of Classification Learning Algorithms
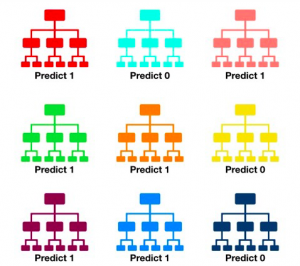
In this post, you will learn about different types of classification machine learning algorithms that are used for building models. Here are four different classes of machine learning algorithms for solving classification problems: Probabilistic modeling Kernel methods Trees based algorithms Neural network Probabilistic Modeling Algorithms Probabilistic modeling is about modeling probability of whethar a data point belongs to one class or the other. In case of need to train machine learning models to classify a data point into multiple classes, probabilistic modeling approach will let us model the probability of a data point belonging to a particular class. Mathematically, it can be represented as P(C|X) and read as probability of class C happening …
Why Deep Learning is called Deep Learning?
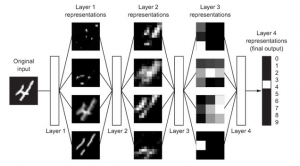
In this post, you will learn why deep learning is called as deep learning. You may recall that deep learning is a subfield of machine learning. One of the key difference between deep learning and machine learning is in the manner the representations / features of data is learnt. In machine learning, the representations of data need to be hand-crafted by the data scientists. In deep learning, the representations of data is learnt automatically as part of learning process. As a matter of fact, in deep learning, layered representations of data is learnt. The layered representations of data are learnt via models called as neural networks. The diagram below represents …
Difference – Artificial Intelligence & Machine Learning
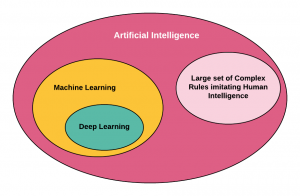
In this post, you learn the difference between artificial intelligence & machine learning. Artificial intelligence represents a set of computer programs that imitate human intelligence. The diagram below represents the key difference between AI and Machine Learning. Basically, machine learning is a part of AI landscape. One can do AI without doing machine learning or deep learning. Thus, an organization can claim that they have AI-based systems without having machine learning or deep learning based systems. All machine learning or deep learning based systems can be termed as AI systems. But, all AI systems may not be termed as machine learning systems. The following are key building blocks of an …
Deep Learning – Learning Feature Representations
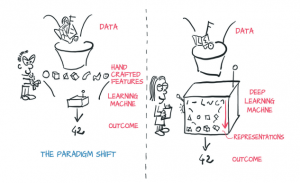
In this post, you learn about what is deep learning with a focus on feature engineering. Here is a quick diagram which represents the idea behind deep learning that Deep learning is about learning features in an automatic manner while optimizing the algorithm. The above diagram is taken from the book, Deep learning with Pytorch. One could learn one of the key differences between training models using machine learning and deep learning algorithms. With machine learning models, one need to engineer features (called as feature engineering) from the data (also called as representations) and feed these features in machine learning algorithms to train one or more models. The model performance …
Machine Learning Models Evaluation Infographics
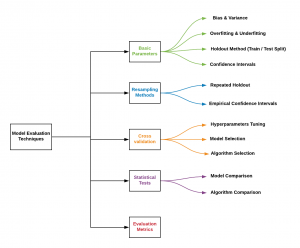
In this post, you will get an access to a self-explanatory infographics / diagram representing different aspects / techniques which need to be considered while doing machine learning model evaluation. Here is the infographics: In the above diagram, you will notice that the following needs to be considered once the model is trained. This is required to be done to select one model out of many models which get trained. Basic parameters: The following need to be considered for evaluating the model: Bias & variance Overfitting & underfitting Holdout method Confidence intervals Resampling methods: The following techniques need to be adopted for evaluating models: Repeated holdout Empirical confidence intervals Cross-validation: Cross …
I found it very helpful. However the differences are not too understandable for me