Angular – Firebase Authentication using Email Password
In this post, you will learn about angular code sample or example related to firebase authentication using email and password in an Angular app. The code has been tested with Angular 7.* and latest version of AngularFire. You will learn some of the following in this post: Login code example using Firebase Method, signInWithEmailAndPassword LoginComponent code for invoking Firebase Authentication using AuthService Login Code Example using Firebase Method, signInWithEmailAndPassword The code could be put inside a file such as auth.service.ts. Pay attention to the fact that an instance of Observable is returned from the login api. In the above code, a GenericResponse object is used for capturing the error message details. The following represents the code for GenericResponse object. …
ML Model Fairness Research from IBM, Google & Others
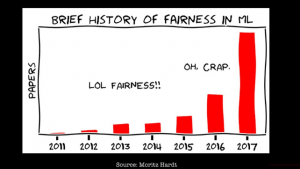
In this post, you would learn about details (brief information and related URLs) on some of the research work done on AI/machine learning model ethics & fairness (bias) in companies such as Google, IBM, Microsoft, and others. This post will be updated from time-to-time covering latest projects/research work happening in various companies. You may want to bookmark the page for checking out the latest details. Before we go ahead, it may be worth visualizing a great deal of research happening in the field of machine learning model fairness represented using the cartoon below, which is taken from the course CS 294: Fairness in Machine Learning course taught at UC Berkley. IBM Research for ML Model Fairness …
Angular 7 Hello World App – Instructions
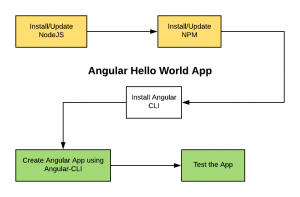
In this post, you would learn about how to quickly get started with Angular 7 hello world app. Here are the steps to build the Angular App. Actually, the steps could be used to build an Angular app with any version of Angular such as Angular 6 or Angular 5. Install NodeJS: Install the NodeJS. Angular requires Node.js version 8.x or 10.x. If you already have Node installed, check the version using the command, node -v and update the NodeJS to latest version appropriately using instructions provided on this page, How to update NodeJS and NPM to next versions. In case you do not have NodeJs installed, go to NodeJS website and download the appropriate NodeJS version. Install …
Fairness Metrics – ML Model Sensitivity for Bias Detection
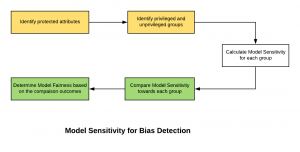
There are many different ways in which machine learning (ML) models’ fairness could be determined. Some of them are statistical parity, the relative significance of features, model sensitivity etc. In this post, you would learn about how model sensitivity could be used to determine model fairness or bias of model towards the privileged or unprivileged group. The following are some of the topics covered in this post: How could Model Sensitivity be used to determine Model Bias or Fairness? Example – Model Sensitivity & Bias Detection How could Model Sensitivity determine Model Bias or Fairness? Model sensitivity could be used as a fairness metrics to measure the model bias towards the privileged or unprivileged group. Higher the …
How to Start DevOps or DevSecOps in your Organization
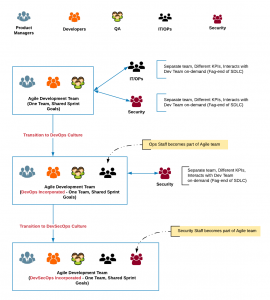
Is your organization starting to face issues related to delay in moving software changes into production due to build failures, environment-related failures, collaboration related issues between dev, QA, security professionals? Is your organization starting to face stiff competition from startups and other competitors due to delay in moving new features to customers in a faster manner? Is your organization looking to serve the customers in a faster manner with new features and bug fixes? If these are some of your concerns, you may want to start considering the adoption of DevOps or DevSecOps principles in your software development lifecycle. In this post, you would learn about some of the following …
Data Science Project Folder Structure
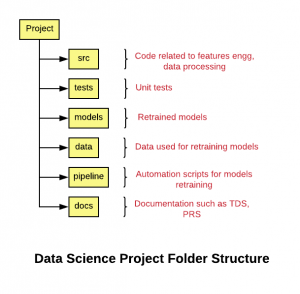
Have you been looking out for project folder structure or template for storing artifacts of your data science or machine learning project? Once there are teams working on a particular data science project and there arises a need for governance and automation of different aspects of the project using build automation tool such as Jenkins, one would feel the need to store the artifacts in well-structured project folders. In this post, you will learn about the folder structure using which you could choose to store your files/artifacts of your data science projects. Folder Structure of Data Science Project The following represents the folder structure for your data sciences project. Note that the project structure is created keeping in mind integration with build and automation jobs. …
Job Description – Chief Artificial Intelligence (AI) Officer
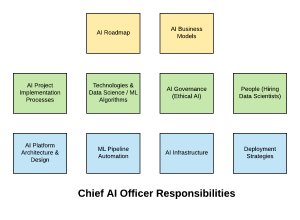
Whether your organization needs a chief artificial intelligence (AI) officer is a topic where there have been differences of opinions. However, the primary idea is to have someone who heads or leads the AI initiatives across the organization. The designation could be chief AI officer, Vice-president (VP) – AI research, Chief Analytics Officer, Chief Data Officer, AI COE Head or maybe, Chief Data Scientist etc. One must understand that building AI/machine learning models and deploying them in production is just one part of the whole story. Aspects related to AI governance (ethical AI), automation of AI/ML pipeline, infrastructure management vis-a-vis usage of cloud services, unique project implementation methodologies etc., become of prime importance once you are done with the hiring of data scientists for …
Bias Detection in Machine Learning Models using FairML
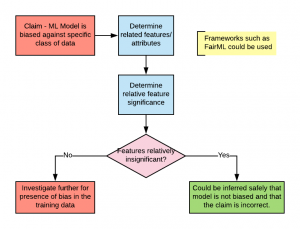
Detecting bias in machine learning model has become of great importance in recent times. Bias in the machine learning model is about the model making predictions which tend to place certain privileged groups at a systematic advantage and certain unprivileged groups at a systematic disadvantage. And, the primary reason for unwanted bias is the presence of biases in the training data, due to either prejudice in labels or under-sampling/over-sampling of data. Especially, in banking & finance and insurance industry, customers/partners and regulators are asking the tough questions to businesses regarding the initiatives taken by them to avoid and detect bias. Take an example of the system using a machine learning model to …
Security Attacks Analysis of Machine Learning Models
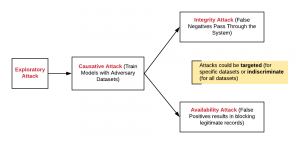
Have you wondered around what would it be like to have your machine learning (ML) models come under security attack? In other words, your machine learning models get hacked. Have you thought through how to check/monitor security attacks on your AI models? As a data scientist/machine learning researcher, it would be good to know some of the scenarios related to security/hacking attacks on ML models. In this post, you would learn about some of the following aspects related to security attacks (hacking) on machine learning models. Examples of Security Attacks on ML Models Hacking machine learning (ML) models means…? Different types of Security Attacks Monitoring security attacks Examples of Security Attacks on ML Models Most of …
JupyterLab & Jupyter Notebook Cheat Sheet Commands
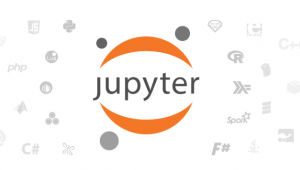
Are you starting to create machine learning models (using python programming) using JupyterLab or Jupyter Notebook? This post list down some commands which are found to be very useful while one (beginner data scientist) is getting started with using JupyterLab notebook for building machine learning models. Notebook Operations: The following command helps to perform operations with the notebook. Ctrl + S: Save the notebook Ctrl + Q: Close the notebook Enter: While on any cell, you want to enter edit mode, press Enter. Cells Operation: The following commands help with performing operations on cells: J: Select the cell below the current cell; This command would be used to go through cells below the …
Missing Data Imputation Techniques in Machine Learning
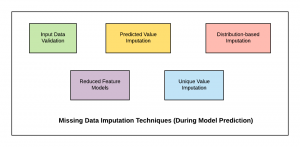
Have you come across the problem of handling missing data/values for respective features in machine learning (ML) models during prediction time? This is different from handling missing data for features during training/testing phase of ML models. Data scientists are expected to come up with an appropriate strategy to handle missing data during, both, model training/testing phase and also model prediction time (runtime). In this post, you will learn about some of the following imputation techniques which could be used to replace missing data with appropriate values during model prediction time. Validate input data before feeding into ML model; Discard data instances with missing values Predicted value imputation Distribution-based imputation Unique value imputation Reduced feature models Below is the diagram …
Code of Ethics in Artificial Intelligence (AI) – Key Traits
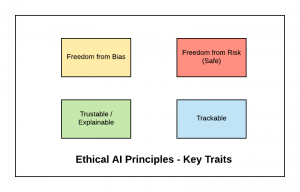
Do you know that organizations have started paying attention to whether AI/machine learning (ML) models are doing unbiased, safe and trustable predictions based on ethical principles? Have you thought through consequences if AI/machine learning (ML) models you created for your clients make predictions which are biased towards a class of customer, thus, hurting other customers? Have you imagined scenarios in which customers blame your organization of benefitting a section of customers (preferably their competitors), thus, filing a case against your organization and bring bad names and loss to your business? Have you imagined the scenarios when ML models start making incorrect predictions which could result in loss of business? If above …
Ethical AI – Lessons from Google AI Principles
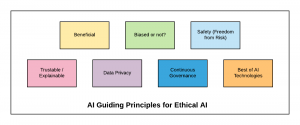
Is your organization using AI/machine learning for many of its products or planning to use AI models extensively for upcoming products? Do you have an AI guiding principles in place for stakeholders such as product management, data scientists/machine learning researchers to make sure that safe and unbiased AI (as appropriate) is used for developing AI-based solutions? Are you planning to create AI guiding principles for the AI stakeholders including business stakeholders, customers, partners etc? If the answer to above is not in affirmation, it is recommended that you should start thinking about laying down AI guiding principles, sooner than later, in place to help different stakeholders such as executive team, …
Why take Google Machine Learning Crash Course?
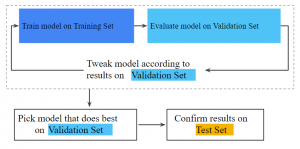
This post represents my thoughts on why you should take the Google Machine Learning (ML) Crash Course. Most importantly, this course would benefit both the beginners and also the intermediate level data scientists/machine learning researchers. Each of the topics is covered as with videos, reading text and programming exercises. You learn some of the following as part of doing the course: ML concepts which help learn concepts related to building machine learning models such as training/validating/testing the models, feature engineering, model overfitting, regularization techniques to penalize complex models, neural networks etc. ML engineering concepts which help learn different aspects of machine learning system such as ML systems components, offline/online training, offline/online prediction, …
How to Choose Right Machine Learning Algorithms?
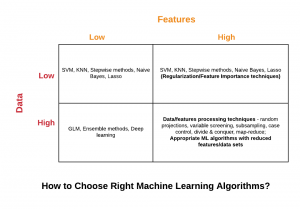
In this post, you will learn about tips and techniques which could be used for selecting or choosing the right machine learning algorithms for your machine learning problem. These could be very useful for those data scientists or ML researcher starting to learn data science/machine learning topics. Based on the following, one could go for selecting different classes of machine learning algorithms for training the models. Availability of data Number of features This post deals with the following different scenarios while explaining machine learning algorithms which could be used to solve related problems: A large number of Features, Lesser Volume of Data A smaller number of Features, Large …
QA – How Reliable are your Machine Learning Systems?
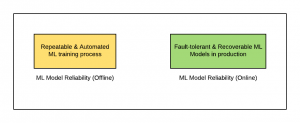
In this post, you will learn about different aspects of creating a machine learning system with high reliability. It should be noted that system reliability is one of the key software quality attributes as per ISO 25000 SQUARE specifications. Have you put measures in place to ensure high reliability of your machine learning systems? In this post, you will learn about some of the following: What is the reliability of machine learning systems? Why bother about machine learning models reliability? Who should take care of the ML systems reliability? What is the Reliability of Machine Learning Systems? As like software applications, the reliability of machine learning systems is primarily related to …
I found it very helpful. However the differences are not too understandable for me