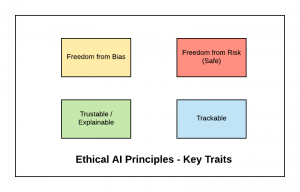
Do you know that organizations have started paying attention to whether AI/machine learning (ML) models are doing unbiased, safe and trustable predictions based on ethical principles? Have you thought through consequences if AI/machine learning (ML) models you created for your clients make predictions which are biased towards a class of customer, thus, hurting other customers? Have you imagined scenarios in which customers blame your organization of benefitting a section of customers (preferably their competitors), thus, filing a case against your organization and bring bad names and loss to your business? Have you imagined the scenarios when ML models start making incorrect predictions which could result in loss of business?
If above have not started haunting you, its time that you started thinking about some of the above. And, this is where you need to think about implementing the code of ethics in implementing artificial intelligence (AI) practices/principles. This post represents the definition of ethical AI, key traits and why businesses need to start paying attention to rolling out ethical AI practices in their organization. The following topics would be discussed:
- What is an ethical AI?
- What are the key traits of ethical AI implementation?
- Why is the need to implement ethical AI principles/practices?
- Who needs to be involved with ethical AI implementation?
What is an Ethical AI?
The meaning of the word, ethics, is moral principles that govern a person’s behavior or the conducting of an activity. In other words, ethics means a set of generalized rules for being a good person. When applied to an organization, an organization doing business based on ethics is governed by a set of principles based on which the organization conducts one or more business activities based on good principles such as honesty, integrity etc.
When ethics get applied to artificial intelligence (AI), it represents the fact that AI/machine learning models make predictions which are trustable/explainable (and hence transparent), unbiased/fair (towards all class of users or entities) and safe (not hurting businesses). Unethical AI would mean that models are biased towards a specific class of users (and, thus, unfair towards other users), or, intended to harm a specific class of user entity (and, thus, unsafe).
Key Traits of Ethical AI Implementation
The following are some of the key traits of implementing ethical AI in your organization:
- Freedom from Bias
- Freedom from Risk (Safe AI)
- Explainable / Trustable
- Trackable
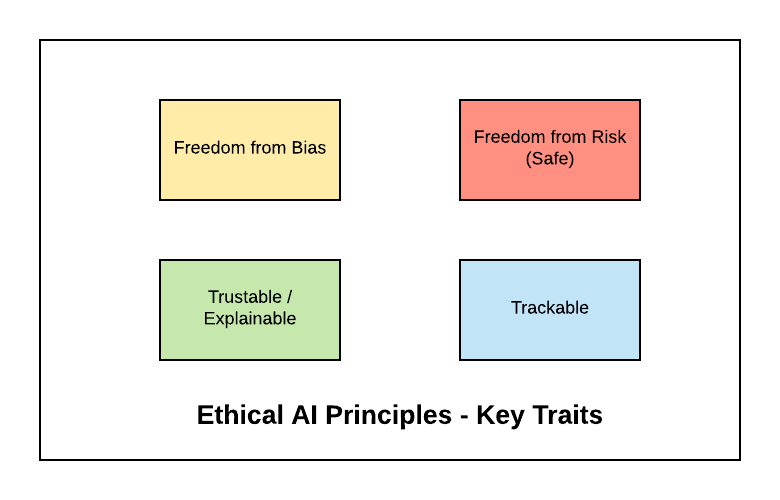
Fig: Key Traits of the Code of Ethics for Artificial Intelligence
Here are the details on above.
- Freedom from Bias: AI/Machine learning models should be trained appropriately while making sure the following is governed:
- The exclusion of one or more appropriate features: At times, some of the features may be left behind intentionally or unintentionally thereby resulting in biased models. There can be scenarios where data related to all the features may not be available. In such cases, one may adopt imputation techniques such as opting different models based on data availability or using the mean/mode of the missing data. This could result in bias.
- The exclusion of the specific classes of the dataset used for training the model, thereby resulting in the biased models. For example, let’s say a model is trained with millions of images to classify whether the image consists of one or more men or women. One of the features becomes the color of the skin. Imagine an instance where the model is trained with images consisting of men or women with white-colored skin. As a result, if the model would be fed with images comprising of men and women with different color skin other than white, the model may not be able to classify the images correctly. This would result in the biased model with the bias towards a specific color of skin.
One may argue the need to intentionally create biased models. Or, one may ask whether any form of bias is bad. Here are some thoughts in relation to the different form of bias or need to create biased models:
- Different forms of Bias: The following are different forms of bias which could be induced in the model:
- Good Bias: If it becomes difficult to create a generalized model due to various different reasons, one could go for creating biased models fulfilling the prediction requirements of a specific group of users. Different models will be trained with the data pertaining to different customers. As a result, the models created will be found to have the bias for the respective customers. This kind of bias found in the model could be called as good bias.
- Bad Bias: Let’s say a model is built to classify a person as happy or otherwise. And, one of the features of the model is monthly income. Let’s say the data used for training the model only consisted of those who have high income labeled as happy. Thus, when such a model is tested with those making lower income, he is predicted to be unhappy. Such a model can be said to be biased toward richer people. And, this bias may be termed as bad bias as it would end up doing incorrect classification of a person being happy or otherwise based on his monthly income.
- When to create biased models: One should go for creating biased models in an informed manner when it becomes difficult to create a generalized model covering different features and different data sets all as part of one model. For example, biased models could be created based on customers, or a particular geography etc.
- Freedom from Risk (Safe AI): The models should be trained appropriately to avoid false positives/false negatives (as appropriate) which could result in loss of business. More importantly, the ML models should be avoided from getting trained with adversary data sets. This is also termed as data poisoning attack. Failing to do so would result in model presenting incorrect results which could lead to impact in business outcomes related to models. In order to achieve the training of model using adversary datasets, one may be required to put appropriate governance/security controls to perform regular checks on the datasets used for training. Product managers/data scientists could be part of the team performing security/governance checks on datasets. Let’s try and understand this using some of the following examples:
- Hospitals may end up losing name/business due to incorrect AI/ML predictions: Let’s say you plan to build an AI/ML solution which aims to predict whether a person is suffering from heart disease. And, you are aiming to have doctors use your solution. It must be ensured that your model does not predict an unhealthy person (one who is suffering from heart disease) as a healthy person (not suffering from heart disease). Your model should have very very high recall rate. Failing to avoid predicting unhealthy person as a healthy person could lead to patients go home with peace and later find his disease taking the toll on his life. This could, in turn, result in the bad name for doctors and related hospital and hence, loss of business. Technically speaking, the model should have no or very very minimal cases of false negatives. In terms of precision/recall, the model is expected to have near 100% recall rate.
- Banks could lose money in loans: Let’s say you built a model helping your banking clients predict whether to give the loans to their customers. Incorrect predictions could result in banks losing money. In this scenario, ideally speaking, the expectation is that the model should have the high precision rate. Or else, the model prediction could result in loss of money. It is not okay to have high false positive which means that those who have lesser likelihood estimate to pay back the money should not be given the loans. In other words, it is expected to have low false positive which means high precision. High precision would mean that of all the predictions made for the eligible candidates, maximum should turn out to be correct.
- Explainable/Trustable Models: Your clients may ask to explain every prediction made by the model. And, it would be a fair ask. Explainability of the model would mean that the model should be able to explain the predictions in the sense which feature contributed to what extent in predictions. Higher the explainability of the model more is the trustability of the model. This would result in the greater satisfaction of your customers using your models. You may choose one of the following techniques to increase the trustability of the model:
- Avoid using black-box models. For instance, in place of using the random forest, use the decision tree. In place of using non-linear classification models, use models built using logistic regression.
- Use a framework which shows the features’ contribution in the predictions.
- Trackable Models: At any point in time, one should be able to track (using logs etc) different aspects of building ML models including some of the following. When asked, the appropriate data could as well be shared with customers and partners to restore their confidence at any given point in time:
- Information (meta-data) on data used for training the models
- Information on features used for training the models
- Information on models’ metrics, model freshness/staleness
- Information on the security of ML pipeline
Why implement Ethical AI Principles/Practices?
The following are some of the reasons why your organization should/must consider implementing ethical AI practices/principles:
- Beneficial for Customers/Partners Business: Ethical AI practices would make sure that your customers and partners businesses are not impacted by your model predictions.
- Beneficial for Society, in general: The aspects related to making a positive impact on customers/partners business would, in turn, result in helping the customers make a positive impact on society at large.
- Stakeholders’ Confidence: Stakeholders including executive management, customers, partners and internal team (product management, data scientist etc) would have a high level of confidence if they come to know that the models created as free from bias, free from risk and trustable.
Stakeholders of Ethical AI Implementation
Who all needs to be involved with ethical AI implementation is the key question? The following could be some of the stakeholders in ethical AI implementation:
- Product Managers / Business Analysts: They need to play an important role during feature and data gathering phase. They would need to make sure all important features are included and data covering different aspects are used for training the models. They also need to play important role in the regular/ongoing feature and data governance during model retraining phase.
- Data Scientists: Data scientists could as well perform the checks given they are provided with enough information on data quality checks to be performed on datasets used for training ML model.
- QA/Governance Team: An additional quality assurance/ML model governance team could be constituted to perform such checks at regular intervals as part of quality control checks.
References
- Ethics of artificial intelligence
- Google AI principles
- Ethical AI – Lessons learned from Google AI Principles
Summary
In this post, you learned about implementing the code of ethics for artificial intelligence (AI) / machine learning models. It is important to set ethical AI principles to ensure AI/ML models are making safe, unbiased and trustable predictions impacting the end customers and partners. Failing to do so would result in loss of business, health, and impact society in a negative manner.
- Mathematics Topics for Machine Learning Beginners - July 6, 2025
- Questions to Ask When Thinking Like a Product Leader - July 3, 2025
- Three Approaches to Creating AI Agents: Code Examples - June 27, 2025
I found it very helpful. However the differences are not too understandable for me