Data Analysis Types: Concepts & Examples
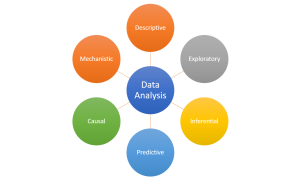
Data analysis plays an important role in understanding the world, discovering trends, and making decisions. Having a good understanding of the different types of data analysis available is essential for anyone looking to make sense of their data. In this blog post, we’ll discuss the six different forms of data analysis and provide examples of each type so you can get a better idea of how they work. The following is a representation of six forms of data analysis. Before getting ahead and understand different form of analysis, lets understand what is Data Analysis? The word “analysis” comes from the Ancient Greek ἀνάλυσις (analysis, “a breaking-up” or “an untying;” from …
Data Quality Characteristics & Examples
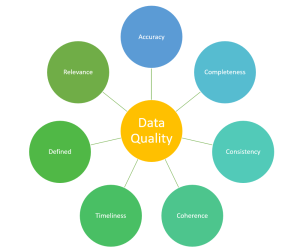
It is no secret that data is an essential component in the day-to-day operations of businesses—as well as the decision making processes. To ensure trust and reliability on the data, organizations must pay close attention to the quality of their data. In this blog post, we will discuss some of the key characteristics that make up quality data, diving into each characteristic and providing examples along the way. Good data governance strategies are also essential for maintaining high quality datasets across an organization’s entire IT infrastructure. These strategies include quality control processes for entering new data into the system; establishing internal documents with procedures for validating all incoming information; assigning …
Ensemble Methods in Machine Learning: Examples
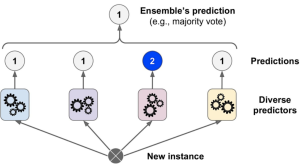
Machine learning models are often trained with a variety of different methods in order to create a more accurate prediction. Ensemble methods are one way to do this, and involve combining the predictions of several different models in order to get a more accurate result. When different models make predictions together, it can help create a more accurate result. Data scientists should care about this because it can help them create models that are more accurate. In this article, we will look at some of the common ensemble methods used in machine learning. Data scientists should care about this because it can help them create models that are more accurate. …
CART Decision Tree Python Example
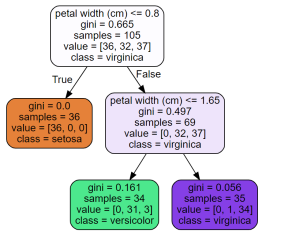
The Classification and Regression Tree (CART) is a supervised machine learning algorithm used for classification, regression. In this blog, we will discuss what CART decision tree is, how it works, and provide a detailed example of its implementation using Python. What is CART & How does it work? CART stands for Classification And Regression Tree. It is a type of decision tree which can be used for both classification and regression tasks based on non-parametric supervised learning method. The following represents the algorithm steps. First and foremost, the data is split into training and test set. Take a feature K and split the training data set into two subsets based on …
Decision Tree Concepts, Examples, Interview Questions
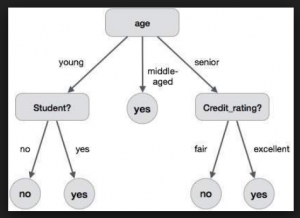
Decision tree is one of the most commonly used machine learning algorithms which can be used for solving both classification and regression problems. It is very simple to understand and use. Here is a lighter one representing how decision trees and related algorithms (random forest etc) are agile enough for usage. In this post, you will learn about some of the following in relation to machine learning algorithm – decision trees vis-a-vis one of the popular C5.0 algorithm used to build a decision tree for classification. In another post, we shall also be looking at CART methodology for building a decision tree model for classification. The post also presents a …
Two-way ANOVA Test: Concepts, Formula & Examples
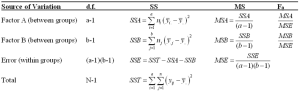
The two-way analysis of variance (ANOVA) test is a powerful tool for analyzing data and uncovering relationships between a dependent variable and two different independent variables. It’s used in fields like psychology, medicine, engineering, business, and other areas that require a deep understanding of how two separate variables interact and impact dependent variable. With the right knowledge, you can use this test to gain valuable insights into your data. Through a two-way ANOVA, data scientists are able to assess complex relationships between multiple variables and draw meaningful conclusions from the data. This helps them make informed decisions and identify patterns in the data that may have gone unnoticed otherwise. Let’s …
Population & Samples in Statistics: Examples
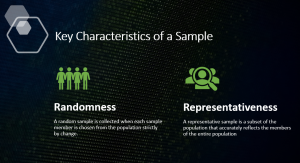
In statistics, population and sample are two fundamental concepts that help us to better understand data. A population is a complete set of objects from which we can obtain data. A population can include all people, animals, plants, or things in a given area. On the other hand, a sample is a subset of the population that is used for observation and analysis. In this blog, we will further explore the concepts of population and samples and provide examples to illustrate the differences between them in statistics. What is a population in statistics? In statistics, population refers to the entire set of objects or individuals about which we want to …
Account Receivables Use Cases for Machine Learning / AI
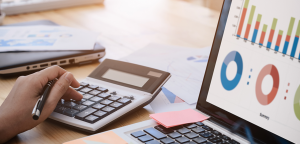
Account receivables (AR) account for a significant portion of total assets and revenue. However, the account receivable process is typically handled manually by accountants or finance staff. This can lead to inefficiencies when it comes to identifying account issues and resolving them quickly. In addition, there are opportunities of leveraging data-driven decision making in different areas related to account receivables. In this blog post, you will learn about account receivables analytics use cases and how AI/machine learning and deep learning techniques can be used to streamline account receivable processes. For product managers and data scientists, this post will prove to be useful to understand different machine learning use cases related …
Bayesian thinking & Real-life Examples
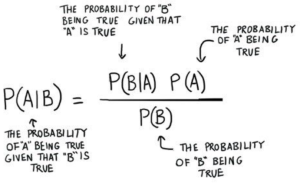
Bayesian thinking is a powerful way of looking at the world, and it can be useful in many real-life situations. Bayesian thinking involves using prior knowledge to make more accurate predictions about future events or outcomes. It is based on the Bayes theorem, which states that the probability of an event occurring is determined by its prior probability combined with new information as it becomes available. It is important for data scientists to learn about Bayesian thinking because it can help them make accurate predictions and draw more meaningful insights from data. In this blog post, we will discuss Bayesian thinking and provide some examples from everyday life to illustrate …
True Error vs Sample Error: Difference
Understanding the differences between true error and sample error is an important aspect of data science. In this blog post, we will be exploring the difference between these two common features of statistical inference. We’ll discuss what they are and how they differ from each other, as well as provide some examples of real-world scenarios where an understanding of both is important. By the end, you should have a better grasp of the differences between true error and sample error. In case you are a data scientist, you will want to understand the concept behind the true error and sample error. These concepts are key to understand for evaluating a …
Confidence Intervals Formula, Examples
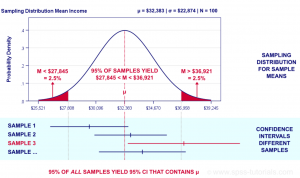
In this post, you will learn about the statistics concepts of confidence intervals in relation to machine learning models with the help of an example and Python code examples. You will learn about how to interpret confidence intervals, what are formulas for confidence intervals with the help of examples. When you get a hypothesis function by training a machine learning classification model, you evaluate the hypothesis/model by calculating the classification error. The classification error is calculated on the sample of the data used for training the model. However, does this classification error for the sample (sample error) also represent (same as) the classification error of the hypothesis/model for the entire …
Paired Sample T-Tests: Formula, Examples
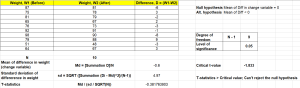
Paired sample t-tests are a commonly used statistical procedure used to compare two populations that are related in some way. They are often used for comparing dependent groups, such as the before and after results of an experiment. Data scientists must have a thorough understanding of the concept of paired sample t-test in order to craft accurate and reliable results when analyzing data. In this blog post, we will explore the formula, assumptions, and examples of paired sample t-tests. What is Paired Sample T-Test and Why is it needed? Paired sample t-tests are used to test whether means of same or similar group different from each other under separate conditions …
Hidden Markov Models: Concepts, Examples
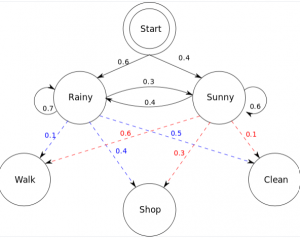
Hidden Markov models (HMMs) are a type of statistical modeling that has been used for several years. They have been applied in different fields such as medicine, computer science, and data science. The Hidden Markov model (HMM) is the foundation of many modern-day data science algorithms. It has been used in data science to make efficient use of observations for successful predictions or decision-making processes. This blog post will cover hidden Markov models with real-world examples and important concepts related to hidden Markov models. What are Markov Models? Markov models are named after Andrey Markov, who first developed them in the early 1900s. Markov models are a type of probabilistic …
Levene Test & Statistics: Concepts & Examples
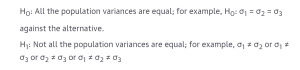
The Levene test is used to test for equality of variance in a dataset. It is used in statistical analysis to determine if two or more samples have similar variances. If the results of the test indicate that the samples do not have similar variances, then it means that one sample has a higher variance than the other and should be treated as an outlier. In this blog post, we’ll take a look at what exactly the Levene test is, how it works, and provide some examples of how it can be applied. As data scientists, it will be important for us to understand the Levene test in order to …
Questions to Ask Before Starting Data Analysis
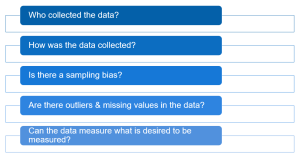
Data analysis is a crucial part of any business or organization. It helps make decisions and assists in strategy development. But before you can dive into the data, there are several questions that need to be answered first. These questions will help you understand whether you have right kind of data for analysis purpose in addition to defining your goals for data analysis. As data scientists or data analysts, it is your job to ask the right questions. Let’s take a look at some important questions to ask before starting data analysis. Who collected the data? When it comes to data analysis, it is essential to know who collected the …
Categorical Data Visualization: Concepts, Examples
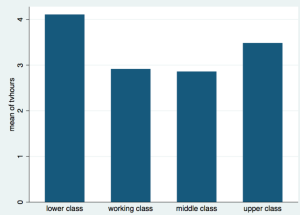
Everyone knows that data visualization is one of the most important tools for any data scientist or statistician. It helps us to better understand the relationships between variables and identify patterns in our data. There are specific types of visualization used to represent categorical data. This type of data visualization can be incredibly helpful when it comes to analyzing our data and making predictions about future trends. In this blog, we will dive into what categorical data visualization is, why it’s useful, and some examples of how it can be used. Types of Data Visualizations for Categorical Dataset When it comes to visualizing categorical data sets, there are primarily four …
I found it very helpful. However the differences are not too understandable for me