Author Archives: Ajitesh Kumar
Reinforcement Learning Real-world examples
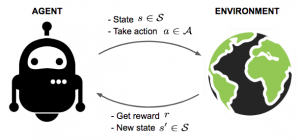
In this blog post, we’ll learn about some real-world / real-life examples of Reinforcement learning, one of the different approaches to machine learning where other approaches are supervised and unsupervised learning. Reinforcement learning is a type of machine learning that enables a computer system to learn how to make choices by being rewarded for its successes. This can be an extremely powerful tool for optimization and decision-making. It’s one of the most popular machine learning methods used today. Before looking into the real-world examples of Reinforcement learning, let’s quickly understand what is reinforcement learning. Introduction to Reinforcement Learning (RL) Reinforcement learning is an approach to machine learning in which the agents …
Passive Aggressive Classifier: Concepts & Examples
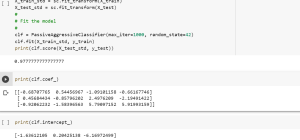
The passive aggressive classifier is a machine learning algorithm that is used for classification tasks. This algorithm is a modification of the standard Perceptron algorithm. The passive aggressive classifier was first proposed in 2006 by Crammer et al. as a way to improve the performance of the Perceptron algorithm on linearly separable data sets. In this blog, we will learn about the basic concepts and principles behind the passive aggressive classifier, as well as some examples of its use in real-world applications. What is the passive aggressive classifier and how does it work? The passive aggressive classifier algorithm falls under the category of online learning algorithms, can handle large datasets, …
Generalized Linear Models Explained with Examples
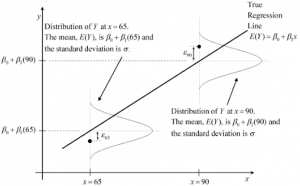
Generalized linear models (GLMs) are a powerful tool for data scientists, providing a flexible way to model data. In this post, you will learn about the concepts of generalized linear models (GLM) with the help of Python examples. It is very important for data scientists to understand the concepts of generalized linear models and how are they different from general linear models such as regression or ANOVA models. What are Generalized Linear Models? Generalized linear models (GLM) are a type of statistical models that can be used to model data that is not normally distributed. It is a flexible general framework that can be used to build many types of regression models, including …
Generate Random Numbers & Normal Distribution Plots
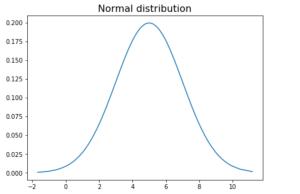
In this blog post, we’ll be discussing how to generate random numbers samples from normal distribution and create normal distribution plots in Python. We’ll go over the different techniques for random number generation from normal distribution available in the Python standard library such as SciPy, Numpy and Matplotlib. We’ll also create normal distribution plots from these numbers generated. Generate random numbers using Numpy random.randn Numpy is a Python library that contains built-in functions for generating random numbers. The numpy.random.randn function generates random numbers from a normal distribution. This function takes size N as in number of numbers to be generated as an input and returns an array of N random …
Pandas: Creating Multiindex Dataframe from Product or Tuples
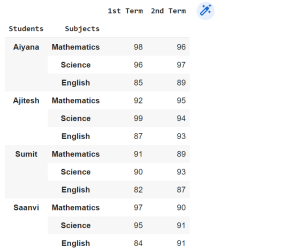
MultiIndex is a powerful tool that enables us to work with higher dimensional data, but it can be tricky to create MultiIndex Dataframes using the from_tuples and from_product function in Pandas. In this blog post, we will be discussing how to create a MultiIndex dataframe using MultiIndex from_tuples and from_product function in Pandas. What is a MultiIndex? MultiIndex is an advanced Pandas function that allows users to create MultiIndexed DataFrames – i.e., dataframes with multiple levels of indexing. MultiIndex can be useful when you have data that can be naturally grouped by more than one category. For example, you might have data on individual employees that can be grouped by …
Top Python Statistical Analysis Packages
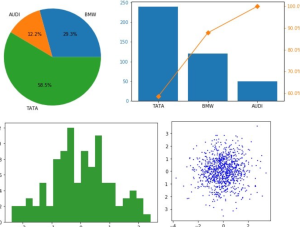
As a data scientist, you know that one of the most important aspects of your job is statistical analysis. After all, without accurate data, it would be impossible to make sound decisions about your company’s direction. Thankfully, there are a number of excellent Python statistical analysis packages available that can make your job much easier. In this blog post, we’ll take a look at some of the most popular ones. SciPy SciPy is a Python-based ecosystem of open-source software for mathematics, science, and engineering. SciPy contains modules for statistics, optimization, linear algebra, integration, interpolation, special functions, Fourier transforms (FFT), signal and image processing, and other tasks common in science and …
Covariance vs. Correlation vs. Variance: Python Examples
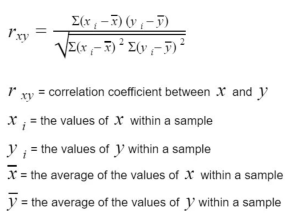
In the field of data science, it’s important to have a strong understanding of statistics and know the difference between related concepts. This is especially true when it comes to the concepts of covariance, correlation, and variance. Whether you’re a data scientist, statistician, or simply someone who wants to better understand the relationships between different variables, it’s important to know the difference between covariance, correlation, and variance. While these concepts may seem similar at first glance, they each have unique applications and serve different purposes. In this blog post, we’ll explore each of these concepts in more detail and provide concrete examples of how to calculate them using Python. What …
Import or Upload Local File to Google Colab
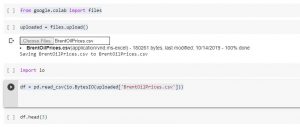
Google Colab is a powerful tool that allows you to run Python code in the cloud. This can be useful for a variety of tasks, including data analysis and machine learning. One of the lesser known features of Google Colab is that you can also import or upload files stored on your local drive. In this article, we will show you how to read a file from your local drive in Google Colab using a quick code sample. There are a few reasons why you as a data scientist might need to learn how to read files from your local drive in Google Colab. One reason is that you may …
Ridge Classification Concepts & Python Examples
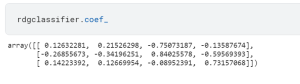
In machine learning, ridge classification is a technique used to analyze linear discriminant models. It is a form of regularization that penalizes model coefficients to prevent overfitting. Overfitting is a common issue in machine learning that occurs when a model is too complex and captures noise in the data instead of the underlying signal. This can lead to poor generalization performance on new data. Ridge classification addresses this problem by adding a penalty term to the cost function that discourage complexity. This results in a model that is better able to generalize to new data. In this post, you will learn about Ridge classifier in detail with the help of …
Pandas Dataframe loc, iloc & brackets examples
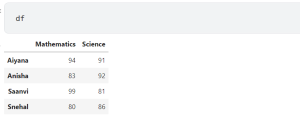
Pandas is a powerful data analysis tool in Python that can be used for tasks such as data cleaning, exploratory data analysis, feature engineering, and predictive modeling. In this article, we will focus on how to use Pandas’ loc and iloc functions on Dataframe, as well as brackets with Dataframe, with examples. As a data scientist or data analyst, it is very important to understand how these functions work and when to use them. In this post, we will work with the following Pandas data frame. Use loc and iloc functions to get Rows of Dataframe The loc function is used to get a particular row in a Dataframe by …
Pandas: How to Create a Dataframe – Examples
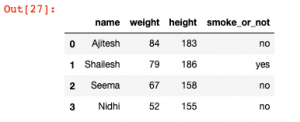
One of the most popular modules for working with data in Python is the Pandas library. Pandas provides data structures and operations for working with structured data. A key concept in Pandas is the Dataframe. Learning how to create and use dataframes is an important skill for anyone including data analysts and data scientists working with data in Python. In this post, you will learn about how to create a Pandas dataframe with some sample data. What is Pandas Dataframe? A Pandas dataframe is a two-dimensional data structure, like a table in a spreadsheet, with columns of data and rows of data. Dataframe is analogous to a table in SQL …
Central Limit Theorem: Concepts & Examples
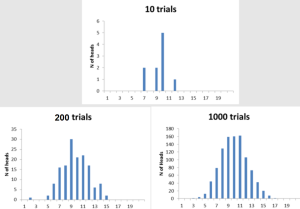
The central limit theorem is one of the most important concepts in statistics. This theorem states that, given a large enough sample size, the distribution of sample averages will be approximately normal. This is a huge deal because it means that we can use the normal distribution to make predictions about populations based on samples. In this article, we’ll explore the central limit theorem in more detail and look at some examples of how it works. As data scientists, it is important to understand the central limit theorem so that we can apply it to real-world situations. What is the central limit theorem and why is it important? The central …
Probability concepts, formulas & real-world examples
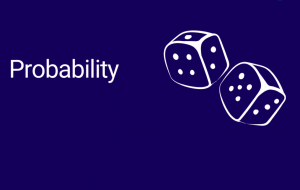
Probability is a branch of mathematics that deals with the likelihood of an event occurring. It is important to understand probability concepts if you want to get good at data science and machine learning. In this blog post, we will discuss the basic concepts of probability and provide examples to help you understand it better. We will also introduce some common formulas associated with probability. So, let’s get started! What is probability and what are the different types? Probability is a concept in mathematics that measures the likelihood of an event occurring. It is typically expressed as a number between 0 and 1, with 0 indicating that an event is …
Statistics – Random Variables, Types & Python Examples
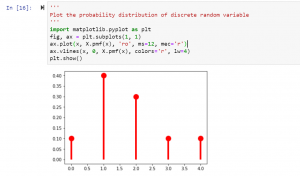
Random variables are one of the most important concepts in statistics. In this blog post, we will discuss what they are, their different types, and how they are related to the probability distribution. We will also provide examples so that you can better understand this concept. As a data scientist, it is of utmost importance that you have a strong understanding of random variables and how to work with them. What is a random variable and what are some examples? A random variable is a variable that can take on random values. The key difference between a variable and a random variable is that the value of the random variable …
How to Create Pandas Dataframe from Numpy Array
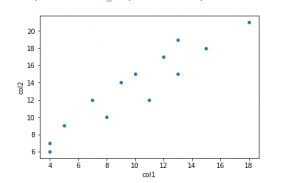
Pandas is a library for data analysis in Python. It offers a wide range of features, including working with missing data, handling time series data, and reading and writing data in different formats. Pandas also provides an efficient way to manipulate and calculate data. One of its key features is the Pandas DataFrame, which is a two-dimensional array with labeled rows and columns. A DataFrame is a table-like structure that contains columns and rows of data. Creating a Pandas DataFrame from a NumPy array is simple. In this post, you will get a code sample for creating a Pandas Dataframe using a Numpy array with Python programming. Step 1: Load …
Machine Learning Sklearn Pipeline – Python Example
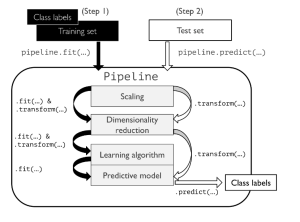
In this post, you will learning about concepts about machine learning (ML) pipeline and how to build ML pipeline using Python Sklearn Pipeline (sklearn.pipeline) package. Getting to know how to use Sklearn.pipeline effectively for training/testing machine learning models will help automate various different activities such as feature scaling, feature selection / extraction and training/testing the models. It is recommended for data scientists (Python) to get a good understanding of Sklearn.pipeline. Introduction to Machine Learning Pipeline & Sklearn.pipeline Machine Learning (ML) pipeline, theoretically, represents different steps including data transformation and prediction through which data passes. The outcome of the pipeline is the trained model which can be used for making the predictions. …
I found it very helpful. However the differences are not too understandable for me