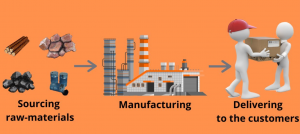
As supply chains become more complex, businesses are looking for new ways to optimize and automate their supply chain operations. One area that is seeing a lot of growth is the use of artificial intelligence (AI) and machine learning in supply chain management. There are many different applications for these technologies in supply chain management, from forecasting demand to optimizing inventory levels. In this blog post, we will explore some of the most interesting use cases for AI and machine learning in supply chain management.
What is supply chain management and what are its key components?
Supply chain management is the process of coordinating and controlling the flow of goods, information, and money between different parties in a supply chain. According to a report by Gartner, supply chain management includes all activities related to the flow of goods and services from supplier to customer. This means that supply chain management involves everything from procurement and production, to warehousing and logistics, to final delivery. In a supply chain, there are typically four different types of organizations:
- Suppliers
- Manufacturers
- Distributors
- Retailers.
Supply chain management involves coordinating and managing these different types of organizations in order to optimize the efficiency of the supply chain.
Supply chain management is a critical component of any business that wants to be successful. In today’s global economy, businesses must be able to move goods quickly and efficiently from suppliers to customers. Supply chain management helps businesses do this by coordinating the different parties in the supply chain and ensuring that everyone is working together to optimize the flow of goods, information, and money. By doing this, businesses can improve their overall performance and competitiveness.
There are four key components of supply chain management: supply planning, supply execution, supply control, and supply performance measurement.
- Supply planning: Supply planning is a critical function for any organization that relies on raw materials or finished goods to produce its products or services. An effective supply plan takes into account a number of factors, including demand forecasts, supplier lead times, and inventory levels. The goal of supply planning is to ensure that the right materials are available when they are needed, in the right quantities.
- Supply execution includes all activities necessary to fill customer orders, such as manufacturing, order processing, warehousing, and transportation.
- Supply control involves monitoring supply levels and ensuring that they remain adequate to meet customer demand. This may involve Inventory management and other techniques such as just-in-time production.
- Supply performance measurement involves tracking supply chain key performance indicators (KPIs) such as delivery time, fill rates, inventory levels, and production costs. This information can be used to identify problems and areas for improvement. supplying goods or services to customers in a timely and cost-effective manner is essential for businesses. supply chain management helps businesses to achieve this by coordinating.
Challenges for Supply Chain Management
Supply chain management is a complex process, and it can be difficult to manage effectively. There are challenges associated with each of the key components listed in the previous section.
- Supply planning: Supply planning can be a complex and challenging process. There are a number of challenges associated with supply planning. First, it can be difficult to accurately forecast demand, especially for new products or in rapidly-changing markets. In addition, supply planners must also balance the need for inventory with the cost of holding too much stock. As a result, supply planning is often a delicate balancing act. Second, even if demand can be accurately predicted, supplier lead times and delivery times can vary, making it hard to ensure that supplies will arrive on time. Third, unexpected events such as natural disasters can disrupt supply chains and cause widespread shortages. Lastly, managing stock levels is a delicate balancing act – too much stock can tie up valuable resources, while too little stock can leave businesses vulnerable to disruptions.
- Supply execution: Supply execution is vital for businesses, as it ensures that goods and services are delivered on time and in the correct quantities. However, supply execution can be a complex and challenging endeavor, with a variety of potential issues that can arise. For example, if demand increases unexpectedly, businesses may find themselves unable to meet customer needs. Similarly, if there are problems with the supply chain, such as delays or disruptions, this can also lead to issues with supply execution. There are a number of potential issues that can arise during supply execution, such as delays, quality issues, and shortages.
- Supply control: One of the most important aspects of supply chain management is supply control. Ensuring that there is a steady supply of raw materials, while at the same time avoiding waste and overproduction, can be a challenge. There are a number of issues that can affect supply control, including fluctuations in demand, changes in production costs, and disruptions to the supply chain. In addition, other factors include weather conditions, geopolitical instability, etc. As a result, supply control issues can quickly escalate into production shutdowns or delivery delays. In order to effectively manage supply control, businesses need to have a clear understanding of their supply and demand, as well as their production capabilities. They also need to be able to respond quickly to changes in the marketplace. By carefully managing supply control, businesses can avoid many of the challenges that can lead to missed sales targets and decreased profits.
- Supply performance management: Ensuring an adequate supply of goods and services is essential to the smooth functioning of any economy. To that end, businesses must continuously monitor supply performance and identify any challenges that may arise. Otherwise, disruptions can quickly lead to supply chain issues, putting a strain on both businesses and consumers. This supply performance can be good, bad or indifferent. Most supply chains have some degree of supply risk. The supply of goods and services may be disrupted due to a number of factors such as supplier failure, natural disasters, geopolitical risks and so on. A supply chain that is not performing optimally will result in increased costs, reduced customer satisfaction and ultimately may lead to the collapse of the supply chain. It is therefore essential that supply Chain Managers constantly monitor supply performance and identify and mitigate any risks to the supply chain. Some of the key challenges faced by Supply Chain Managers include:
- Supplier failure: This is when a supplier is unable to meet its contractual obligations due to financial distress, technological obsolescence or other reasons. This can disrupt the supply of goods and leads to increased costs.
- Natural disasters: Natural disasters such as floods, earthquakes and hurricanes can damage infrastructure and disrupt the supply of goods.
- Geopolitical risks: Tensions between countries can lead to trade disruptions and make it difficult to source goods from certain countries.
- Job losses: When there is a downturn in the economy, companies may lay off workers which can lead to a shortage of labor and skills.
AI / Machine learning use cases for supply chain management
Given the challenges listed, one can leverage AI / machine learning based solutions to tackle some of these challenges. The following is a list of some of the AI / machine learning use cases in relation to supply chain management.
- Demand forecasting: Demand forecasting is a critical part of effective supply chain management. By understanding future demand, businesses can ensure that they have the materials and resources they need to meet customer demand. There are a variety of demand forecasting methods, ranging from simple trend analysis to complex machine learning models. The right method depends on the data available and the specific needs of the business. For example, demand forecasting can be used to predict demand for a specific product, understand the impact of a new marketing campaign, or forecast sales for a particular store. In each case, demand forecasting can help businesses make more informed decisions about their operations. Read about demand forecasting in this post – Demand forecasting & machine learning techniques. The following are some important questions which can drive different use cases or assess the current demand forecasting methods adopted by your organization:
- All all important factors considered when forecasting demand?
- Has their been anomaly related to changes in demand that was not predicted by demand forecasting methods / models? If so, what happened? Have they been tracked?
- Have different data sets including internal and external dataset been considered for forecasting demand?
- Are you keeping a watch on new business levers and related sources of data to enhance the forecasting models?
- Have you tested / evaluated different demand forecasting models? Are you aware of limitations of different models?
- Optimal inventory stocking: Inventory stocking and machine learning go hand-in-hand. inventory stocking is the process of keeping track of inventory levels and reordering inventory when necessary. Machine learning can be used to predict inventory levels and optimize stocking decisions. There are a number of different use cases for inventory stocking and machine learning. For example, inventory stocking can be used to predict demand for products and optimize inventory levels accordingly. Machine learning can also be used to forecast Sales and understand which products are selling well and which are not. Additionally, machine learning can be used to monitor inventory levels in real-time and alert decision-makers when inventory is low. The following are some important questions to consider when building models related to inventory stocking:
- Has their been considerations for appropriate suppliers for uncommon inventory items?
- How has the models been enhanced from time-to-time for the stocking levels that were inaccurate?
- Has all kind of data (internal and external) been used for training the machine learning model to stock inventory?
- What factors or features were considered when building models that drove decisions related optimal inventory stocking?
- Delivery lead time prediction: Delivery lead time is the time it takes between a customer placing an order and the delivery of the product. It is a key metric in managing supply chain operations and customer satisfaction. In a supply chain, delivery lead times can have a major impact on customer satisfaction, inventory levels, and overall costs. However, predicting delivery lead times is often difficult due to the many variables involved. In recent years, there has been an increasing interest in using machine learning to predict delivery lead time. This is because delivery lead times can be highly variable and depend on many factors, such as the manufacturing process, supplier performance, transportation conditions, and so on. Machine learning can be used to build models that consider all of these factors and make more accurate predictions. There are many potential use cases for delivery lead time prediction, such as optimizing production schedules, forecasting demand, and improving customer service. In any case, machine learning can be a powerful tool for managing delivery lead times more effectively. The following are some important questions to consider when building models related to predicting delivery time:
- What types of data have been used to predict delivery lead times in your supply chain?
- How has delivery time prediction accuracy been measured and reported?
- Has different machine learning models been tried for reducing delivery lead times?
- What features have been considered when building models for predicting delivery lead times?
- Supplier financial health prediction: Supplier financial health is a key metric for any company that relies on suppliers in its supply chain. The health of a supplier can have a major impact on the timely delivery of goods and services, and on the quality of those products. As a result, many companies are turning to machine learning to predict the financial health of suppliers. Machine learning algorithms can analyze a variety of data sources, including financial statements, news articles, and social media posts, to identify patterns that may indicate financial distress. In addition, machine learning can be used to monitor changes in supplier financial health over time, allowing companies to take proactive measures to avoid disruptions in their supply chains. Supplier financial health prediction is just one of many potential use cases for machine learning in supply chain management.
Machine learning is a powerful tool that can be used in a variety of ways to improve supply chain operations. In this post, we have explored some of the key use cases for machine learning in supply chain management: demand forecasting, inventory stocking, and supplier financial health prediction. Each of these use cases has the potential to improve business efficiency and bottom line. As businesses continue to adopt machine learning into their supply chains, we can expect even more innovative applications of this technology. We will continue to add the use cases with time. Please feel free to suggest.
- Completion Model vs Chat Model: Python Examples - June 30, 2024
- LLM Hosting Strategy, Options & Cost: Examples - June 30, 2024
- Application Architecture for LLM Applications: Examples - June 25, 2024
Leave a Reply