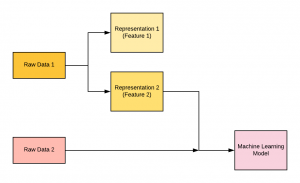
Machine learning is a field of machine intelligence concerned with the design and development of algorithms and models that allow computers to learn without being explicitly programmed. Machine learning has many applications including those related to regression, classification, clustering, natural language processing, audio and video related, computer vision, etc. Machine learning requires training one or more models using different algorithms. Check out this detailed post in relation to learning machine learning concepts – What is Machine Learning? Concepts & Examples.
One of the most important aspects of the machine learning model is identifying the features which will help create a great model, the model that performs well on unseen data. In this blog post, we will learn about features and related aspects. Let’s quickly understand what is machine learning.
What is Machine Learning?
Machine learning is about learning one or more mathematical functions/models using data to solve a particular task. Any machine learning problem can be represented as a function of three parameters.
Machine Learning Problem = < T, P, E >
In the above expression, T stands for the task, P stands for performance and E stands for experience (past data). A machine learning model learns to perform a task using past data and is measured in terms of performance (error). Here is my detailed post on machine learning concepts and examples.
Machine learning models are trained using data that can be represented as raw features (same as data) or derived features (derived from data). Let’s look into the next section on what are features.
What are the features in machine learning?
Features are nothing but the independent variables in machine learning models. What is required to be learned in any specific machine learning problem is a set of these features (independent variables), coefficients of these features, and parameters for coming up with appropriate functions or models (also termed hyperparameters). The following represents a few examples of what can be termed as features of machine learning models:
- A model for predicting the risk of cardiac disease may have features such as the following:
- Age
- Gender
- Weight
- Whether the person smokes
- Whether the person is suffering from diabetic disease, etc.
- A model for predicting whether the person is suitable for a job may have features such as the educational qualification, number of years of experience, experience working in the field etc
- A model for predicting the size of a shirt for a person may have features such as age, gender, height, weight, etc.
Features are of two types. They are following:
- Continuous features: Continuous features are numerical values that can take on any value within a certain range. This type of data is often used to represent things such as time, weight, income, temperature, etc. Continuous features are often used in machine learning applications, since they can provide a more detailed representation of data than discrete or categorical features. For example, imagine that you are trying to predict the weight of an animal based on its height. If you only had discrete data for height (e.g., “short,” “medium,” and “tall”), then your predictions would be less accurate than if you had continuous data (e.g., the animal’s actual height in inches or centimeters). Continuous features can also be more useful than discrete features when it comes to optimizing models.
- Categorical or discrete features: Categorical features are an important part of machine learning. Categorical data is data that can be divided into categories, such as “male” and “female” or “red” and “blue.” Categorical features can be used to help predict what category something belongs to, based on other features. Categorical data can be thought of as a set of categories, and each category can be represented by a number. For example, if we are predicting the type of animal based on a series of features, the animal’s species would be a categorical feature. Categorical features are of two types – nominal and ordinal.
The following represents above two types of features.
Features can be in the form of raw data that is very straightforward and can be derived from real-life as it is. However, not all problems can be solved using raw data or data in its original form. Many times, they need to be represented or encoded in different forms. For example, a color can be represented in RGB format or HSV format. Thus, a color can have two different representations or encodings. And, both of these representations or encodings can be used to solve different kinds of problems. Some tasks that may be difficult with one representation can become easy with another. For example, the task “select all red pixels in the image” is simpler in the RGB format, whereas “make the image less saturated” is simpler in the HSV format.
Machine-learning models are all about finding appropriate representations / features for their input data—transformations of the data that make it more amenable to the task at hand, such as a classification task.
In the case of machine learning, it is the responsibility of data scientists to hand-craft some useful representations/features from the given data set. In the case of deep learning, the feature representations are learned automatically based on the underlying algorithm. One of the most important reasons why deep learning took off instantly is that it completely automates what used to be the most crucial step in a machine-learning workflow: feature engineering
One way to understand the features is the representation of data in the form that can be used to map input data to the output. For many machine learning tasks or problems, these representations are hand-crafted. For example, if the problem is to classify whether a person is suffering from a specific disease or otherwise, the representations or features can be his/her blood report, gender, age, weight, etc. You may note that the representations can easily be hand-crafted. However, if the problem is to classify whether an object is a dog or cat, the representations or features that can map to the output (dog or cat) can be very difficult to identify. In such ML problems or tasks, it can be very cumbersome to hand-craft the features or representations. In such scenarios, there are representation algorithms that are used to identify correct representations. One such algorithm is Autoencoder. The figure given below represents the usage of hand-crafted representations/features and raw data in building machine learning models.
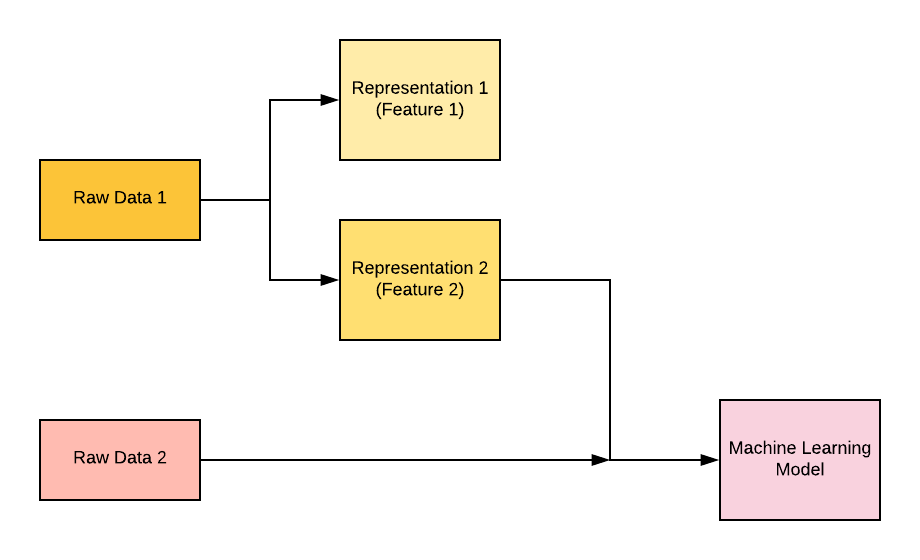
Fig 1. Features – Key to Machine Learning
The process of coming up with new representations or features including raw and derived features is called feature engineering.
Hand-crafted features can also be called as derived features.
The subsequent step is to select the most appropriate features out of these features. This is called feature selection. Here are my related posts in relation to feature engineering:
What are the characteristics of good features?
Here are some characteristics of good features:
- Features must be found in most of the data samples: Great features represent unique characteristics that can be applied across different types of data samples and are not limited to just one data sample. For example, can the “red” color of the apple act as a feature? Not really. Because apples can be found in different colors. It might have happened that the sample of apples that was taken for evaluation contained apples of just a “red” color. If not found, we may end up creating models having high bias.
- Features must be unique and may not be found prevalent with other (different) forms: Great features are the ones that are unique to apple and should not be applicable to other fruits. The toughness characteristic of apples such as “hard to teeth” may not be a good feature. This is because guava can also be explained using this feature.
- Features in reality: There can be features that can be accidental in nature and is not a feature at all when considering the population. For example, in a particular sample of data, a particular kind of feature can be found to be prevalent. However, when multiple data samples are taken, the feature goes missing.
A great feature must satisfy all of the above criteria. From that perspective, one can design derived features appropriately if the features represented using raw data do not satisfy the above criteria. Creating/deriving good features from raw data is also called feature engineering. The following are the two most important aspects of feature engineering:
- Feature extraction
- Feature selection: Here are some related posts in relation to different features selection technique:
How do we determine good features?
Reasoning by first principles can be of great help when analyzing the features of your model. In first principles thinking, we break down the problem into its constituents parts and work to arrive at the most basic causes or first causes. In relation to the machine learning model representing the real-world problem, these first causes can become the features of the model. For example, if there is a need to model a real world situation of a student scoring good or bad marks in the examination, we can apply first principles thinking and try and arrive at the most basic causes such as the following in relation to the student getting marks in the examination:
- What are the books referred to? Are they the ones recommended by the school or teachers, or, they are extra books as well?
- Does the student take extra coaching?
- Does the student make the notes diligently in the class?
- Does the student refer to the class notes as preparatory material?
- Does the student get help from his/her parents?
- Does the student get help from his/her siblings?
- Is the student introvert or extrovert?
References
- Questions to Ask When Thinking Like a Product Leader - July 3, 2025
- Three Approaches to Creating AI Agents: Code Examples - June 27, 2025
- What is Embodied AI? Explained with Examples - May 11, 2025
I found it very helpful. However the differences are not too understandable for me