Category Archives: Data Science
Sklearn SVM Classifier using LibSVM – Code Example
In this post, you learn about Sklearn LibSVM implementation used for training an SVM classifier, with code example. Here is a great guide for learning SVM classification, especially, for beginners in the field of data science/machine learning. LIBSVM is a library for Support Vector Machines (SVM) which provides an implementation for the following: C-SVC (Support Vector Classification) nu-SVC epsilon-SVR (Support Vector Regression) nu-SVR Distribution estimation (one-class SVM) In this post, you will see code examples in relation to C-SVC, and nu-SVC LIBSVM implementations. I will follow up with code examples for SVR and distribution estimation in future posts. Here are the links to their SKLearn pages for C-SVC and nu-SVC …
SVM – Understanding C Value with Code Examples
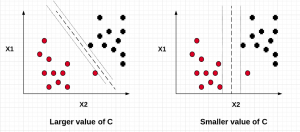
In this post, we will understand the importance of C value on the SVM soft margin classifier overall accuracy using code samples. In the previous post titled as SVM as Soft Margin Classifier and C Value, the concepts around SVM soft margin classifier and the importance of C value was explained. If you are not sure about the concepts, I would recommend reading earlier article. Lets take a look at the code used for building SVM soft margin classifier with C value. The code example uses the SKLearn IRIS dataset In the above code example, take a note of the value of C = 0.01. The model accuracy came out to …
SVM as Soft Margin Classifier and C Value
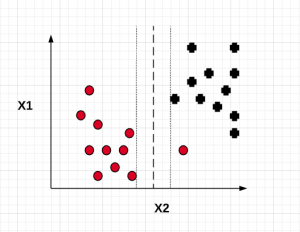
In this post, you will learn about SVM (Support Vector Machine) as Soft Margin Classifier and the importance of Value of C. In the previous post, we learned about SVM as maximum margin classifier. What & Why of SVM as Soft Margin Classifier? Before getting into understanding what is Soft Margin Classifier version of SVM algorithm, lets understand why we need it when we had a maximum margin classifier. Maximum margin classifier works well with linearly separable data such as the following: When maximum margin classifier is trained on the above data set with maximum distance (margin) between the closest points (support vectors), we can get a hyperplane which can separate the data in a clear …
SVM Algorithm as Maximum Margin Classifier
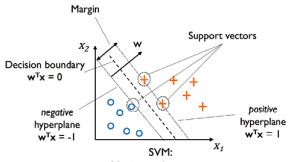
In this post, we will understand the concepts related to SVM (Support Vector Machine) algorithm which is one of the popular machine learning algorithm. SVM algorithm is used for solving classification problems in machine learning. Lets take a 2-dimensional problem space where a point can be classified as one or the other class based on the value of the two dimensions (independent variables, say) X1 and X2. The objective is to find the most optimal line (hyperplane in case of 3 or more dimensions) which could correctly classify the points with most accuracy. In the diagram below, you could find multiple such lines possible. In the above diagram, the objective is to find the …
Top 5 Data Analytics Methodologies
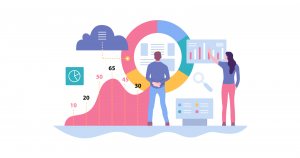
Here is a list of top 5 data analytics methodologies which can be used to solve different business problems and in a way create business value for any organization: Optimization: Simply speaking, an optimization problem consists of maximizing or minimizing a real function by systematically choosing input values (also termed as decision variables) from within an allowed set and computing the value of the function. An optimization problem consists of three things: A. Objective function B. Decision variables C. Constraint functions (this is optional) Linear / Non-linear programming with constrained / unconstrained optimization Linear programming with constrained optimization Objective function and one or more constraint functions are linear with decision variables as continuous variables Linear programming with unconstrained optimization Objective function …
Contract Management Use Cases for Machine Learning
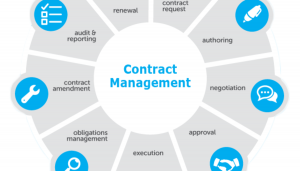
This post briefly represent the contract management use cases which could be solved using machine learning / data science. These use cases can also be termed as predictive analytics use cases. This can be useful for procurement business functions in any manufacturing companies which require to procure raw materials from different suppliers across different geographic locations. The following are some of the examples of industry where these use cases and related machine learning techniques can be useful. Pharmaceutical Airlines Food Transport Key Analytics Questions One must understand the business value which could be created using predictive analytics use cases listed later in this post. One must remember that one must start with questions …
Why Deep Learning is called Deep Learning?
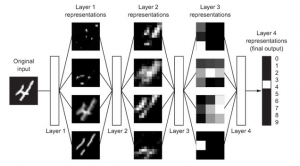
In this post, you will learn why deep learning is called as deep learning. You may recall that deep learning is a subfield of machine learning. One of the key difference between deep learning and machine learning is in the manner the representations / features of data is learnt. In machine learning, the representations of data need to be hand-crafted by the data scientists. In deep learning, the representations of data is learnt automatically as part of learning process. As a matter of fact, in deep learning, layered representations of data is learnt. The layered representations of data are learnt via models called as neural networks. The diagram below represents …
Difference – Artificial Intelligence & Machine Learning
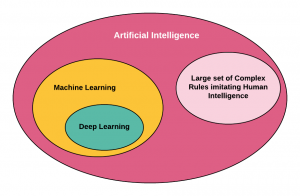
In this post, you learn the difference between artificial intelligence & machine learning. Artificial intelligence represents a set of computer programs that imitate human intelligence. The diagram below represents the key difference between AI and Machine Learning. Basically, machine learning is a part of AI landscape. One can do AI without doing machine learning or deep learning. Thus, an organization can claim that they have AI-based systems without having machine learning or deep learning based systems. All machine learning or deep learning based systems can be termed as AI systems. But, all AI systems may not be termed as machine learning systems. The following are key building blocks of an …
Machine Learning Models Evaluation Infographics
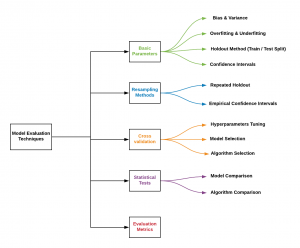
In this post, you will get an access to a self-explanatory infographics / diagram representing different aspects / techniques which need to be considered while doing machine learning model evaluation. Here is the infographics: In the above diagram, you will notice that the following needs to be considered once the model is trained. This is required to be done to select one model out of many models which get trained. Basic parameters: The following need to be considered for evaluating the model: Bias & variance Overfitting & underfitting Holdout method Confidence intervals Resampling methods: The following techniques need to be adopted for evaluating models: Repeated holdout Empirical confidence intervals Cross-validation: Cross …
Python – How to Plot Learning Curves of Classifier
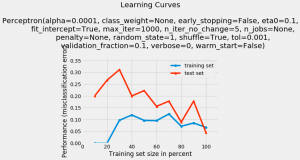
In this post, you will learn a technique using which you could plot the learning curve of a machine learning classification model. As a data scientist, you will find the Python code example very handy. In this post, the plot_learning_curves class of mlxtend.plotting module from mlxtend package is used. This package is created by Dr. Sebastian Raschka. Lets train a Perceptron model using iris data from sklearn.datasets. The accuracy of the model comes out to be 0.956 or 95.6%. Next, we will want to see how did the learning go. In order to do that, we will use plot_learning_curves class of mlxtend.plotting module. Here is a post on how to install mlxtend with Anaconda. The following …
Infographics for Model & Algorithm Selection & Evaluation
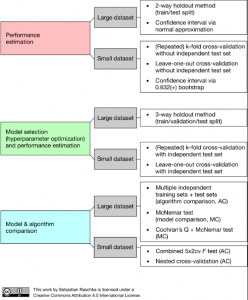
This is a short post created for quick reference on techniques which could be used for model evaluation & selection and model and algorithm comparision. This would be very helpful for those aspiring data scientists beginning to learn machine learning or those with advanced data science skills as well. The image has been taken from this blog, Comparing the performance of machine learning models and algorithms using statistical tests and nested cross-validation authored by Dr. Sebastian Raschka The above diagram provides prescription for what needs to be done in each of the following areas with small and large dataset. Very helpful, indeed. Model evaluation Model selection Model and algorithm comparison …
Feature Scaling & Stratification for Model Performance (Python)
In this post, you will learn about how to improve machine learning models performance using techniques such as feature scaling and stratification. The following topics are covered in this post. The concepts have been explained using Python code samples. What is feature scaling and why one needs to do it? What is stratification? Training Perceptron model without feature scaling and stratification Training Perceptron model with feature scaling Training Perceptron model with feature scaling and stratification What is Feature Scaling and Why is it needed? Feature scaling is a technique of standardizing the features present in the data in a fixed range. This is done when data consists of features of varying …
How to use Sklearn Datasets For Machine Learning
In this post, you wil learn about how to use Sklearn datasets for training machine learning models. Here is a list of different types of datasets which are available as part of sklearn.datasets Iris (Iris plant datasets used – Classification) Boston (Boston house prices – Regression) Wine (Wine recognition set – Classification) Breast Cancer (Breast cancer wisconsin diagnostic – Classification) Digits (Optical recognition of handwritten digits dataset – Classification) Linnerud (Linnerrud dataset – Classification) Diabetes (Diabetes – Regression) The following command could help you load any of the datasets: All of the datasets come with the following and are intended for use with supervised learning: Data (to be used for …
Python – How to install mlxtend in Anaconda
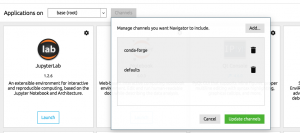
In this post, you will quickly learn about how to install mlxtend python package while you are working with Anaconda Jupyter Notebook. Mlxtend (machine learning extensions) is a Python library of useful tools for the day-to-day data science tasks. This library is created by Dr. Sebastian Raschka, an Assistant Professor of Statistics at the University of Wisconsin-Madison focusing on deep learning and machine learning research. Here is the instruction for installing within your Anaconda. Add a channel namely conda-forge by clicking on Channels button and then Add button. Open a command prompt and execute the following command: conda install mlxtend –channel Conda-forge Once installed, launch a Jupyter Notebook and try importing the following. This should work …
Python DataFrame – Assign New Labels to Columns
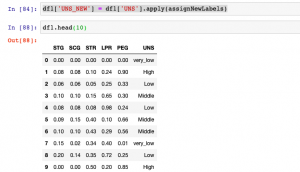
In this post, you will get a code sample related to how to assign new labels to columns in python programming while training machine learning models. This is going to be very helpful when working with classification machine learning problem. Many a time the labels for response or dependent variable are in text format and all one wants is to assign a number such as 0, 1, 2 etc instead of text labels. Beginner-level data scientists will find this code very handy. We will look at the code for the dataset as represented in the diagram below: In the above code, you will see that class labels are named as very_low, Low, High, Middle …
Java Implementation for Rosenblatt Perceptron
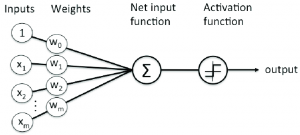
In this post, you will learn about Java implementation for Rosenblatt Perceptron. Rosenblatt Perceptron is the most simplistic implementation of neural network. It is also called as single-layer neural network. The following diagram represents the Rosenblatt Perceptron: The following represents key aspect of the implementation which is described in this post: Method for calculating “Net Input“ Activation function as unit step function Prediction method Fitting the model Calculating the training & test error Method for calculating “Net Input” Net input is weighted sum of input features. The following represents the mathematical formula: $$Z = {w_0}{x_0} + {w_1}{x_1} + {w_2}{x_2} + … + {w_n}{x_n}$$ In the above equation, w0, w1, w2, …
I found it very helpful. However the differences are not too understandable for me