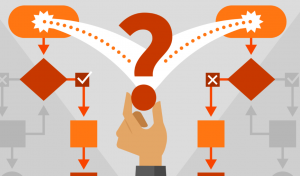
In this post, you will learn about some popular and most common real-life examples of machine learning (ML) classification problems. For beginner data scientists, these examples of classification problems will prove to be helpful to gain perspectives on real-world problems which can be solved using classification algorithms in machine learning. This post will be updated from time-to-time to include interesting examples which can be solved by training classification models.
Before going ahead and looking into examples, let’s understand a little about what is an ML classification problem. You may as well skip this section if you are familiar with the definition of machine learning classification problems & solutions.
You may want to check out my post on what is machine learning to get a better understanding of machine learning concepts with the help of examples.
What’s Classification Problem in Machine Learning?
Machine learning (ML) classification problems are those which require the given data set to be classified in two or more categories. For example, whether a person is suffering from a disease X (answer in Yes or No) can be termed as a classification problem. Another common example is whether to buy a thing from the online portal now or wait for couple of months in order to get maximum discount. Or, if you are planning to buy a car, which car out of available options is a best buy given your budget.
Classification problems are different than regression problems primarily in their outputs. Classification problems involve categorizing data into discrete classes or labels, such as “spam” or “not spam” in email filtering. In contrast, regression problems predict continuous, numerical outputs, like forecasting sales or temperatures. The algorithms and evaluation metrics used also differ; classification employs metrics like precision, recall, accuracy or F1 score, while regression uses mean squared error or R-squared. This fundamental distinction in the nature of the output dictates the approach and techniques used in solving these problems.
Take a quick test: Which of the following is an example of a classification problem?
- A) Predicting the future stock price of a company based on historical data
- B) Categorizing emails as ‘spam’ or ‘not spam’
- C) Grouping customers based on purchasing behavior
- D) Diagnosing whether a patient has a certain disease or not based on symptoms
The right answers are option B and D. Option A represent regression problem and option C represents clustering problem.
Different Types of Classification Problems
Classification problems can be of the following different types:
- Binary classification – Classifies data into two classes such as Yes / No, good/bad, high/low, suffers from a particular disease or not, etc. The picture below represents classification model representing the lines separating two different classes. Depending upon the type of problems and linear / non-linear data, the boundary separating the classes would be of different nature. Check out this post on linear vs non-linear data.
- Multinomial classification: Classifies data into three or more classes; Document classification, product categorization, malware classification
In both types of problems mentioned above, one of the following approaches is following in terms of classifying the data:
- The data is classified as hard assignments in one of the categories or classes.
- The data is classified as soft assignments, e.g., the probability that each category or class applies to the data. Thus, if there are three 10 classes for a digit recognition from 0 to 9, the classification output will be the probability that each class applies for a particular digit. Thus, 0 can be 0.01, and 1 can be 0.3, 3 as 0.05, 4 as 0.02, 5 as 0.0.8, 6 as 0.1, 7 as 0.5, etc. Thus, the digit can be classified as belonging to the 7 class based on the probability value.
Machine learning algorithms for solving Classification Problems
Classification problems are supervised learning problems wherein the training data set consists of data related to independent and response variables (label). The classification models are trained using some of the following algorithms:
- Logistic regression
- Decision trees
- Random forest
- XGBoost
- Light GBM
- Voting classifiers
- Artificial neural networks
When training a model for a classification problem based on supervised learning, there are different strategies that are followed to assign label the response or output variable:
- Assigning integer to the output: If there are three classes such as dog, cat, and horse, one can follow {1, 2, 3} for {dog, cat, and horse} respectively.
- Assigning a one-hot encoding vector: One can assign a one-hot encoding vector to label the output. One-hot encoding represents a vector that has as many components as classes or categories. The component corresponding to a particular instance’s class or category is set to 1 and all other components are set to 0. Thus, the one-hot encoding vector for the dog class will be (1, 0, 0). For cat class, it will be (0, 1, 0). For horse class, it is (0, 0, 1). Thus, the output labels can be represented as {(1, 0, 0), (0, 1, 0), (0, 0, 1)}
Classification Problems & Models Examples
Here is the list of real-life examples of classification problems and the classification models which can be used to solve these problems. Each of these can also be understood as applications of classification models.
- Customer behavior prediction: Customers can be classified into different categories based on their buying patterns, web store browsing patterns etc. For example, classification models can be used to determine whether a customer is likely to purchase more items or not. If the classification model predicts a greater likelihood that they are about to make more purchases, then you might want to send them promotional offers and discounts accordingly. Or if it has been determined that they will probably fall off of their purchasing habits soon, maybe save them for later by making their information readily available.
- Document classification: A multinomial classification model can be trained to classify documents in different categories. In this case, the classification model can be thought of as a function that maps from a document to a category label. Different algorithms can be used for document classification such as Naive Bayes classifier, Support Vector Machines (SVM), or Neural Networks models. Deep learning algorithms such as Deep Boltzmann Machines (DBMs), Deep Belief Networks (DBNs), and Stacked Autoencoders (SAEs) give state-of-the-art classification results on different document classification datasets.
- Spam filtering: A classification algorithm is trained to recognize spam email by learning the characteristics of what constitutes spam vs non-spam email. The classification model could be a function that maps from an email text to a spam classification (or non-spam classification). Algorithms such as Naive Bayes and Support Vector Machines can be used for classification. Once the classification model is trained, it can then be used to filter new incoming emails as spam or non-spam. The picture below represents the Spam classification model depicted as Spam classifier.
- Image classification: One of the most popular classification problems is image classification: determining what type of object (or scene) is in a digital image. Images can be thought of as a high-dimensional vectors which we would like to classify into different classes such as cat, car, human, and airplane. A multinomial classification model can be trained to classify images into different categories. For example, in order to classify images of dogs and cats for use within machine vision systems, machine learning techniques can help automate this process based on pre-classified images of dogs and cats.rent categories. Deep learning algorithms such as Convolutional Neural Networks (CNN)-based classification models are state-of-the-art in different image classification tasks. Another use case is image segmentation, where the pixels of an image are assigned a label based on what object they belong to. Image segmentation is defined as “the process of distinguishing semantically meaningful image regions on the basis of visual features”. The picture below represents how the CNN algorithm can be used to build a classification model that classifies images such as Cat and dog.
- Web text classification: Classifying web pages/documents into different topics is another classification problem. This classification task can be carried out by mapping a text document to its corresponding topic category, which can further be used for other downstream classification tasks such as automatic tagging of web pages. The naive Bayes classification model is usually used for this classification task, but deep learning models have been shown to give better classification accuracy than naive Bayes models. For example, classification models can be used to automatically classify web text into one of the following categories: Sports, Entertainment, or Technology. Google news is a classical example of this classification problem: it automatically classifies articles into different topic categories. Here is the diagram representing the same:
- Ad click-through rate prediction: Binary classification models can be used to predict whether one or more ads on the website will be clicked or not. Such models are used to optimize the ad inventory on websites by selecting which ads will have a better chance of being clicked. A machine learning classification model can be built using historical data about what types of users do or don’t click on certain ads, along with information like demographics and content within each web page where an ad appears; then it is used to predict the chances that a user will click on an ad.
- Product categorization: A multinomial classification can be used to categorize the products sold by different retailers in the same categories irrespective of categories assigned to the product by the respective retailers. This use case is relevant for eCommerce aggregators. Product classification is used in catalog-based shopping websites such as Amazon, where products are automatically classified into different categories based on their features or usage. Read this page on product categorization for greater details.
- Malware classification: A multinomial classification can be used to classify the new/emerging-malware on the basis of comparable features of similar malware. Malware classification is very useful for security experts to take appropriate actions for combating/preventing malware. Machine learning classification algorithms such as Naïve Bayes, k-NN, and tree-based models can be used for malware classification.
- Image sentiment analysis: Machine learning binary classification models can be built based on machine learning algorithms to classify whether the image contains a positive or negative emotion/sentiment or not. This use case is relevant in the field of social media analytics where machine learning techniques are applied to understand users’ opinions and sentiments on different topics.
- Customer churn prediction: A binary classification model can be used to classify whether a customer will churn or not in the near future. The application of the customer churn classification model can be found in different business scenarios like up-selling/cross-selling to existing customers, identifying at-risk accounts in the customer base, etc. More commonly, telecommunications companies have been found to use machine learning classification models for churn prediction.
- Customer behavior assessment for promotional offers: A binary classification model can be used to classify whether an account is customer-friendly or not in the context of a specific business scenario like upselling, cross-selling, etc. For example, based on past data about how customers respond to certain types of offers; machine learning techniques can be used to predict whether a given customer will respond positively or negatively to the offer.
- Anomaly detection problems such as fraud detection: Anomaly detection models can be built using machine learning classification algorithms like Naïve Bayes, k-NN, etc. The application of these machine learning anomaly detection models is very wide and includes use cases such as finding unusual patterns in financial transactions that may indicate fraud, finding machine problems by detecting unusual machine readings, and monitoring machine parameters to detect abnormalities. In the case of an anomaly detection problem, the machine learning model is trained to predict a particular class (e.g., normal or anomalous) and the classification model is then used to classify new data as either belonging to the normal set of data points or anomalous set of data points. The classification algorithm should be able to detect rare cases that lie outside the training distribution and can be used to detect possible fraudulent credit card transactions, for example.
- Credit card fraud detection: A binary classification model can be used for credit card fraud detection where the historical transaction data of a customer is analyzed using machine learning algorithms like Naïve Bayes, k-NN, etc. Based on past fraudulent or non-fraudulent transaction data and machine learning classification models, it can be predicted whether the given credit card will result in fraudulent transactions or not.
- Deduction validation classification: A binary classification model can be used to classify whether a deduction claimed by the buyer on a given invoice is a valid or invalid deduction. This would be useful in account receivables to classify whether the given invoice will be paid in full or partial based on deduction validation classification.
- Credit-worthiness assessment: A machine learning classification model can be trained to predict the probability of default for a customer based on past transaction data and historical information about customers who have defaulted/not defaulted in their payments. Credit card companies, financial institutions like banks, etc
- Blocked order release recommendation: A binary classification model can be built to classify whether an order placed by the customer should be blocked or not based on the buyer credit exposure. This use case is very prevalent in account receivables where machine learning classification models are used to predict whether a given order should be blocked or not. This would help the business save costs by identifying high-risk customers.
- Sentiment analysis: A machine learning binary classification model can be trained to identify the sentiment (positive/negative) of a given text document based on classification algorithms like Naïve Bayes, SVM etc. This would help determine whether the sentiment expressed in a document such as an email is positive or negative for business purposes like identifying whether a customer is satisfied or dissatisfied with the service provided.
Machine learning classification models can be used to solve a wide variety of business problems. There are many ML algorithms that can be applied in order to solve classification problems. For example, classification models could detect fraud by looking at unusual patterns in financial transactions which may indicate fraudulent activity. Classification models are also widely implemented within the telecommunications industry where machine learning classification models have been found to predict churn rates with more accuracy than traditional methods based on historical data from past customers.
If you are a business analyst, a product manager, or someone associated with building products, you may want to check out my latest book on reasoning by first principles titled – First principles thinking: Building winning products using first principles thinking. You may as well check out the related blog – First principles thinking explained with examples.
- Mathematics Topics for Machine Learning Beginners - July 6, 2025
- Questions to Ask When Thinking Like a Product Leader - July 3, 2025
- Three Approaches to Creating AI Agents: Code Examples - June 27, 2025
Hi Ajitesh,
I came across this article while doing research for my university project where I am meant Define a business problem that can be solved using Machine Learning – Classification in the chosen company (binary or multi-class) (not a regression, forecasting).
and Find a sample dataset suitable to solve the same.I you can give guidance on the same and data,i have checked in kaggle but i couldnt an applicable dataset i am seeking to use Orange software for my analysis.Regards