Tag Archives: Data Science
QA – Blackbox Testing for Machine Learning Models
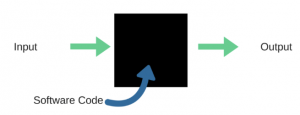
Data science/Machine learning career has primarily been associated with building models which could do numerical or class-related predictions. This is unlike conventional software development which is associated with both development and “testing” the software. And, the related career profiles are software developer/engineers and test engineers/QA professional. However, in the case of machine learning, the career profile is a data scientist. The usage of the word “testing” in relation to machine learning models is primarily used for testing the model performance in terms of accuracy/precision of the model. It can be noted that the word, “testing”, means different for conventional software development and machine learning models development. Machine learning models would …
Assessing Quality of AI Models from QA Standpoint
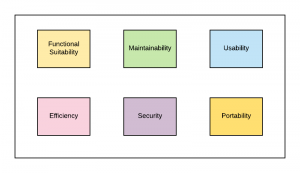
In this post, you will learn about the definition of quality of AI / machine learning (ML) models. Getting a good understanding of what is the high and low quality of AI models would help you design quality control checks for testing machine learning models and related quality assurance (QA) practices. This post would be a good read for QA professionals in general. However, it would also help set perspectives for data scientists and machine learning experts. The following are some of the key quality traits which are described in detail for assessing the quality of AI models: Functional suitability Maintainability Usability Efficiency Security Portability When designing QA practice and related quality control checks, all of the above would need to be considered for testing …
QA – Metamorphic Testing for Machine Learning Models
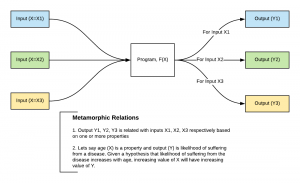
In this post, you will learn about how metamorphic testing could be used for performing quality control checks/testing on machine learning models. The post is primarily meant for data science (QA) specialists to plan the test cases to test the machine learning (ML) model implementation from QA perspective. Testing machine learning models from a quality assurance perspective is different from testing machine learning models for accuracy/performance. The word “testing” is one of the conflicting technical nomenclatures given its usage by machine learning experts and software engineering community in general. In this post, the following topics are discussed: Introduction to metamorphic testing Why metamorphic testing for machine learning models? Automated metamorphic testing of ML models Introduction …
QA – Why Machine Learning Systems are Non-testable
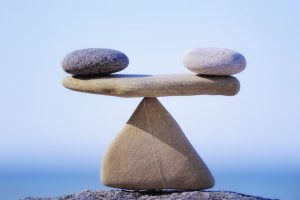
This post represents views on why machine learning systems or models are termed as non-testable from quality control/quality assurance perspectives. Before I proceed ahead, let me humbly state that data scientists/machine learning community has been saying that ML models are testable as they are first trained and then tested using techniques such as cross-validation etc., based on different techniques to increase the model performance, optimize the model. However, “testing” the model is referred with the scenario during the development (model building) phase when data scientists test the model performance by comparing the model outputs (predicted values) with the actual values. This is not the same as testing the model for any given input for which the …
QA – Testing Features of Machine Learning Models
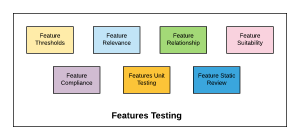
In this post, you will learn about different types of test cases which you could come up for testing features of the data science/machine learning models. Testing features are one of the key set of QA tasks which needed to be performed for ensuring the high performance of machine learning models in a consistent and sustained manner. Features make the most important part of a machine learning model. Features are nothing but the predictor variable which is used to predict the outcome or response variable. Simply speaking, the following function represents y as the outcome variable and x1, x2 and x1x2 as predictor variables. y = a1x1 + a2x2 + a3x1x2 + e In the above function, …
QA of Machine Learning Models with PDCA Cycle
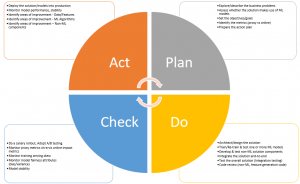
The primary goal of establishing and implementing Quality Assurance (QA) practices for machine learning/data science projects or, projects using machine learning models is to achieve consistent and sustained improvements in business processes making use of underlying ML predictions. This is where the idea of PDCA cycle (Plan-Do-Check-Act) is applied to establish a repeatable process ensuring that high-quality machine learning (ML) based solutions are served to the clients in a consistent and sustained manner. The following diagram represents the details. The following represents the details listed in the above diagram. Plan Explore/describe the business problems: In this stage, product managers/business analyst sit with data scientist and discuss the business problem at hand. The outcome of this …
QA & Data Science – How to Test Features Relevance
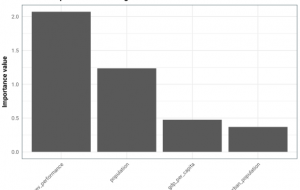
In this post, I intend to present a perspective on the need for QA / testing team to test the feature relevance when testing the machine learning models as part of data science QA initiatives, and, different techniques which could be used to test or perform QA on feature relevance. Feature relevance can also be termed as feature importance. Simply speaking, a feature is said to be relevant or important if it adds real predictive value to the underlying model. The relevant features must display a stable statistical relationship or association with the outcome variable. Well, an association does not imply a causation. However, a relevant feature or a feature …
Quality Assurance / Testing the Machine Learning Model
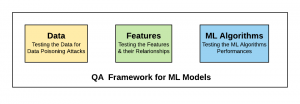
This is the first post in the series of posts related to Quality Assurance & Testing Practices and Data Science / Machine Learning Models which I would release in next few months. The goal of this and upcoming posts would be to create a tool and framework which could help you design your testing/QA practices around data science/machine learning models. Why QA Practices for testing Machine Learning Models? Are you a test engineer and want to know about how you could make difference in AI initiative being undertaken by your current company? Are you a QA manager and looking for or researching tools and frameworks which could help your team perform QA with …
AI – Three Different types of Machine Learning Algorithms
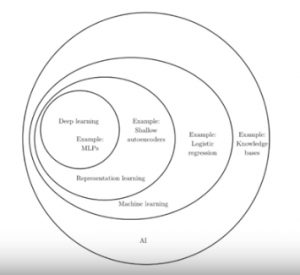
This post is aimed to help you learn different types of machine learning algorithms which forms the key to artificial intelligence (AI). Machine learning algorithms Representation or Feature learning algorithms Deep learning algorithms The following represents different types of learning algorithms in form of a Venn diagram. What are Machine Learning (ML) Algorithms? Machine learning algorithms are the most simplistic class of algorithms when talking about AI. ML algorithms are based on the idea that external entities such as business analysts and data scientists need to work together to identify the features set for building the model. The ML algorithms are, then, trained to come up with coefficients for each of the features and how are they …
8 Machine Learning Javascript Frameworks to Explore
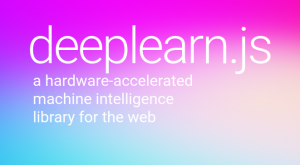
Javascript developers tend to look out for Javascript frameworks which can be used to train machine learning models based on different machine learning algorithms. The following are some of the machine learning algorithms using which models can be trained using different javascript frameworks listed in this article: Simple linear regression Multi-variate linrear regression Logistic regression Naive-bayesian K-nearest neighbour (KNN) K-means Support vector machine (SVM) Random forest Decision tree Feedforward neural network Deep learning network In this post, you will learn about different Javascsript framework for machine learning. They are some of the following: Deeplearn.js Propel ConvNetJS ML-JS KerasJS STDLib Limdu.js Brain.js DeepLearn.js Deeplearn.js is an open-source machine learning Javascript library …
Data Science – What are Machine Learning (ML) Models?
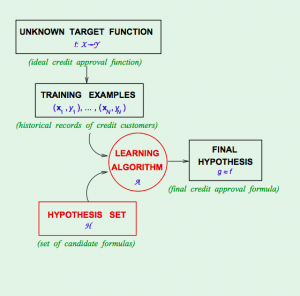
Machine learning (ML) models is the most commonly used in a data science project. In this post, you will learn about different definitions of a machine learning model to get a better understanding of what are machine learning models? A model is the relationship between features and the label. (Tensorflow – Getting Started for ML Beginners) An ML model is a mathematical model that generates predictions by finding patterns in your data. (AWS ML Models) ML Models generate predictions using the patterns extracted from the input data (Amazon Machine learning – Key concepts) Learning in the supervised model entails creating a function that can be trained by using a training …
10+ Key Stages of Data Science Project Life cycle
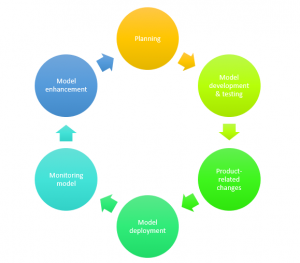
Data science projects need to go through different project lifecycle stages in order to become successful. In each of the stages, different stakeholders get involved as like in a traditional software development lifecycle. In this post, you will learn some of the key stages/milestones of data science project lifecycle. This article is aimed to help some of the following project stakeholders who play key roles in data science project implementation: Product managers Project managers ML architects The following represents 6 high-level stages of data science project lifecycle: Planning Model development & testing Product-level changes Model deployment Monitoring the model Model Enhancement Data Science Project Lifecycle – Planning ML Problem identification: …
Tutorials – Building Machine Learning Models for Predicting Cancer
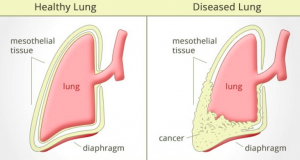
In this article, I would introduce different aspects of the building machine learning models to predict whether a person is suffering from malignant or benign cancer while emphasizing on how machine learning can be used (predictive analysis) to predict cancer disease, say, Mesothelioma Cancer. The approach such as below can as well be applied to any other diseases including different types of cancers. Predicting Mesothelioma Cancer – Supervised Learning Problem Machine learning problems are classified into different kinds of learning problem. Most important of them are following: Supervised learning Unsupervised learning Supervised Learning In supervised learning, you have a history of data with each record being labeled. Thus, in case of predictive analysis of Mesothelioma cancer, there is …
Neural Networks Interview Questions – Set 2
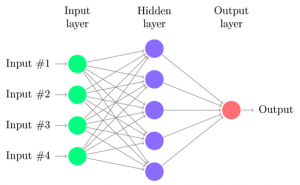
This quiz represents practice test on artificial neural networks. These questions and answers can be as well used for your upcoming interviews for the position of machine learning engineer or data scientist. These questions can prove to be very useful for testing your neural networks knowledge from time-to-time. Also, these will be useful for interns / freshers / beginners of machine learning / data science. The topics covered in this practice test are following: Introduction to different types of neural networks such as Radial Basis Network, Recurrent neural network etc. Difference between multilayer perceptron (MLP) and Radial basis function network Practice Test on Neural Networks
70 Regression Analysis Interview Questions & Practice Tests
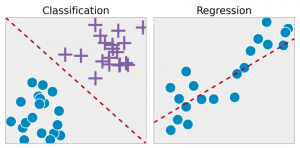
This page lists down practice tests (questions and answers), links to PDF files (consisting of interview questions) on Linear / Logistic Regression for machine learning / data scientist enthusiasts. These questions can prove to be useful, especially for machine learning / data science interns / freshers / beginners to check their knowledge from time-to-time or for upcoming interviews. Practice Tests on Linear / Multilinear Regression These are a set of four practice tests (consisting of 40 questions) covering linear (univariate) and multilinear (multivariate) regression in detail. Linear, Multiple regression interview questions and answers – Set 1 Linear, Multiple regression interview questions and answers – Set 2 Linear, Multiple regression interview …
Logistic Regression Interview Questions & Practice Tests
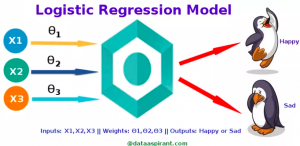
This page lists down a set of 30 interview questions on Logistic Regression (machine learning/data science) in form of objective questions and also provides links to a set of three practice tests that would help you test / check your knowledge on an ongoing basis. These questions and practice tests are intended to primarily help interns/freshers/beginners to help them brush up their knowledge in logistic regression from time to time. The following is a list of topics covered on this page. Introduction to logistic regression Logistic regression examples Evaluating performance of logistic regression and related techniques including AIC, deviance, ROC etc. Difference between linear and logistic regression Here is another post on …
I found it very helpful. However the differences are not too understandable for me