Author Archives: Ajitesh Kumar
Great Mind Maps for Learning Machine Learning
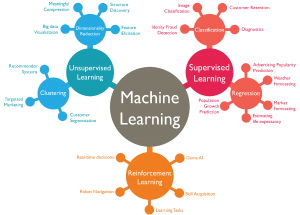
In this post, you will get to look at some of the great mind-maps for learning different machine learning topics. I have gathered these mind maps from different web pages on the Internet. The idea is to reinforce our understanding of different machine learning topics using pictures. You may have heard the proverb – A picture is worth a thousand words. Keeping this in mind, I thought to pull some of the great mind maps posted on different web pages. I would be updating this blog post from time-to-time. If you are a beginner data scientist or an experienced one, you may want to bookmark this page for refreshing your …
Different Types of Distance Measures in Machine Learning
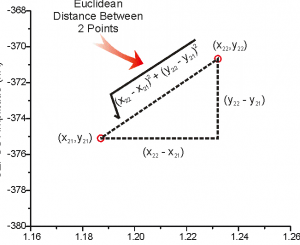
In this post, you will learn different types of distance measures used in different machine learning algorithms such as K-nearest neighbours, K-means etc. Distance measures are used to measure the similarity between two or more vectors in multi-dimensional space. The following represents different forms of distance metrics / measures: Geometric distances Computational distances Statistical distances Geometric Distance Measures Geometric distance metrics, primarily, tends to measure the similarity between two or more vectors solely based on the distance between two points in multi-dimensional space. The examples of such type of geometric distance measures are Minkowski distance, Euclidean distance and Manhattan distance. One other different form of geometric distance is cosine similarity which will discuss …
Introduction to Algorithms & Related Computational Tasks
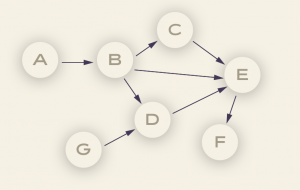
In this post, you will be introduced to some of the important class of algorithms and related computational tasks which could be taken care using these algorithms. Here are some important classes of algorithms which will be briefly discussed in this post: Divide and conquer algorithms Graphs based algorithms Greedy algorithms Dynamic programming Linear programming NP-complete algorithms Quantum algorithms Divide-and-Conquer Algorithms Divide and conquer algorithms are the algorithms which can be used to solve problems using divide and conquer strategy. The following represents the steps of divide-and-conquer algorithms: Breaking it into subproblems that are themselves smaller instances of the same type of problem Recursively solving these subproblems Appropriately combining their …
Machine Learning Terminologies for Beginners
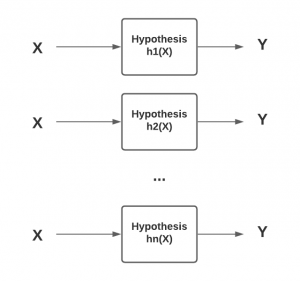
When starting on the journey of learning machine learning and data science, we come across several different terminologies when going through different articles/posts, books & video lectures. Getting a good understanding of these terminologies and related concepts will help us understand these concepts in a nice manner. At a senior level, it gets tricky at times when the team of data scientists / ML engineers explain their projects and related outcomes. With this in context, this post lists down a set of commonly used machine learning terminologies that will help us get a good understanding of ML concepts and also engage with the DS / AI / ML team in …
Machine Learning Free Course at Univ Wisconsin Madison
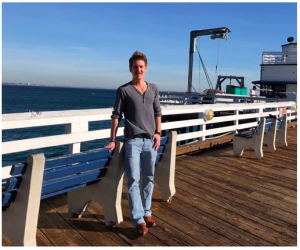
In this post, you will learn about the free course on machine learning (STAT 451) recently taught at University of Wisconsin-Madison by Dr. Sebastian Raschka. Dr. Sebastian Raschka in currently working as an assistant Professor of Statistics at the University of Wisconsin-Madison while focusing on deep learning and machine learning research. The course is titled as “Introduction to Machine Learning”. The recording of the course lectures can be found on the page – Introduction to machine learning. The course covers some of the following topics: What is machine learning? Nearest neighbour methods Computational foundation Python Programming (concepts) Machine learning in Scikit-learn Tree-based methods Decision trees Ensemble methods Model evaluation techniques Concepts of …
Starting on Analytics Journey – Things to Keep in Mind
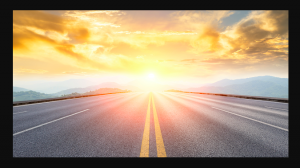
This post highlights some of the key points to keep in mind when you are starting on data analytics journey. You may want to check a related post to assess where does your organization stand in terms of maturity of analytics practice – Analytics maturity model for assessing analytics practice. In the post sighted above, the analytics maturity model defines three different levels of maturity which are as following: Challenged Practitioners Innovators At whichever level you are in terms of maturity of your analytics practice, it may be good idea to understand the following points to come up with data analytics projects. Believe that a lot of prior work is required …
MIT Free Course on Machine Learning (New)
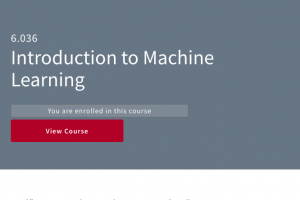
In this post, the information regarding new free course on machine learning launched by MIT OpenCourseware. In case, you are a beginner data scientist or ML Engineer, you will find this course to be very useful. Here is the URL to the free course on machine learning: https://bit.ly/37iNNAA. This course, titled as Introduction to Machine Learning, introduces principles, algorithms, and applications of machine learning from the point of view of modeling and prediction. It includes formulation of learning problems and concepts of representation, over-fitting, and generalization. These concepts are exercised in supervised learning and reinforcement learning, with applications to images and to temporal sequences. Here are some of the key topics for which lectures can be found: …
Gradient Boosting Regression Python Examples
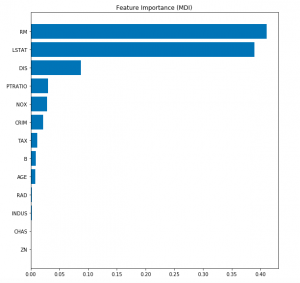
In this post, you will learn about the concepts of Gradient Boosting Regression with the help of Python Sklearn code example. Gradient Boosting algorithm is one of the key boosting machine learning algorithms apart from AdaBoost and XGBoost. What is Gradient Boosting Regression? Gradient Boosting algorithm is used to generate an ensemble model by combining the weak learners or weak predictive models. Gradient boosting algorithm can be used to train models for both regression and classification problem. Gradient Boosting Regression algorithm is used to fit the model which predicts the continuous value. Gradient boosting builds an additive mode by using multiple decision trees of fixed size as weak learners or …
Data Quality Challenges for Analytics Projects
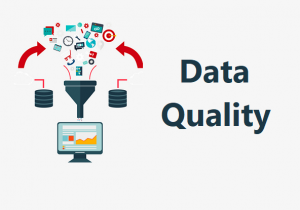
In this post, you will learn about some of the key data quality challenges which you may need to tackle with, if you are working on data analytics projects or planning to get started on data analytics initiatives. If you represent key stakeholders in analytics team, you may find this post to be useful in understanding the data quality challenges. Here are the key challenges in relation to data quality which when taken care would result in great outcomes from analytics projects related to descriptive, predictive and prescriptive analytics: Data accuracy / validation Data consistency Data availability Data discovery Data usability Data SLA Cos-effective data Data Accuracy One of the most important …
Data Science vs Data Engineering Team – Have Both?
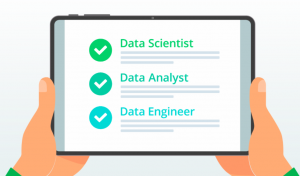
In this post, you will learn about different aspects of data science and data engineering team and also understand the key differences between them. As data science / engineering stakeholders, it is very important to understand whether we need to have one or both the teams to achieve high quality dataset & data pipelines as well as high-performant machine learning models. Background When an organization starts on the journey of building data analytics products, primarily based on predictive analytics, it goes on to set up a centralized (mostly) data science team consisting of data scientists. The data science team works with the product team or multiple product teams to gather the …
500+ Machine Learning Interview Questions
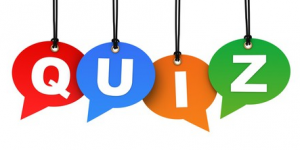
This post consists of all the posts on this website in relation to interview questions / quizzes related to data science / machine learning topics. These questions can prove to be helpful for the following: Product managers Data scientists Product Managers Interview Questions Find the questions for product managers on this page – Machine learning interview questions for product managers Data Scientists Interview Questions Here are posts representing 500+ interview questions which will be helpful for data scientists / machine learning engineers. You will find it useful as practise questions and answers while preparing for machine learning interview. Decision tree questions Machine learning validation techniques questions Neural networks questions – …
Spacy Tokenization Python Example
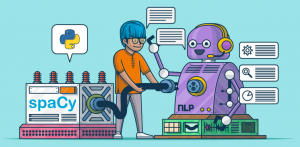
In this post, you will quickly learn about how to use Spacy for reading and tokenising a document read from text file or otherwise. As a data scientist starting on NLP, this is one of those first code which you will be writing to read the text using spaCy. First and foremost, make sure you have got set up with Spacy, and, loaded English tokenizer. The following commands help you set up in Jupyter notebook. Reading text using spaCy: Once you are set up with Spacy and loaded English tokenizer, the following code can be used to read the text from the text file and tokenize the text into words. Pay attention …
Top 10 Types of Analytics Projects – Examples
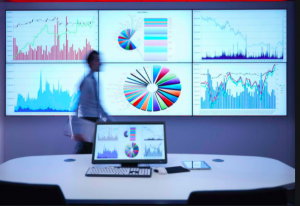
In this post, you will learn about some of the most common types of data analytics projects which can be executed by the organization to realise associated business value from analytics projects and, also, gain competitive advantage with respect to the related business functions. Note that analytics projects are different from AI / ML projects. AI / ML or predictive analytics is one part of analytics. Other types of analytics projects include those related with descriptive and prescriptive analytics. You may want to check out one of my related posts on difference between predictive and prescriptive analytics. Here are the key areas of focus for data analytics projects: Cost reduction: …
Predictive vs Prescriptive Analytics Difference
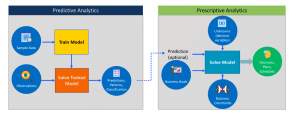
In this post, you will quickly learn about the difference between predictive analytics and prescriptive analytics. As data analytics stakeholders, one must get a good understanding of these concepts in order to decide when to apply predictive and when to make use of prescriptive analytics in analytics solutions / applications. Without further ado, let’s get straight to the diagram. In the above diagram, you could observe / learn the following: Predictive analytics: In predictive analytics, the model is trained using historical / past data based on supervised, unsupervised, reinforcement learning algorithms. Once trained, the new data / observation is input to the trained model. The output of the model is prediction in form …
Negative Binomial Distribution Python Examples
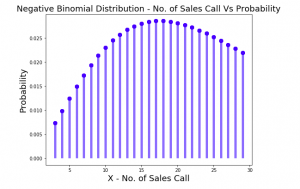
In this post, you will learn about the concepts of negative binomial distribution explained using real-world examples and Python code. We will go over some of the following topics to understand negative binomial distribution: What is negative binomial distribution? What is difference between binomial and negative binomial distribution? Negative binomial distribution real-world examples Negative binomial distribution Python example What is Negative Binomial Distribution? Negative binomial distribution is a discrete probability distribution representing the probability of random variable, X, which is number of Bernoulli trials required to have r number of successes. This random variable is called as negative binomial random variable. And, the experiment representing X number of Bernoulli trials required to product r successes is called …
NLTK – How to Read & Process Text File
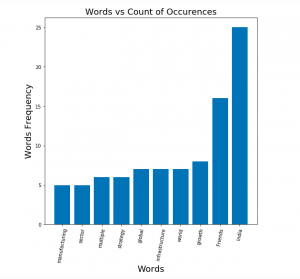
In this post, you will learn about the how to read one or more text files using NLTK and process words contained in the text file. As data scientists starting to work on NLP, the Python code sample for reading multiple text files from local storage will be very helpful. Python Code Sample for Reading Text File using NLTK Here is the Python code sample for reading one or more text files. Pay attention to some of the following aspects: Class nltk.corpus.PlaintextCorpusReader reader is used for reading the text file. The constructor takes input parameter such as corpus root and the regular expression representing the files. List of files that are read could be found using method such as fileids List …
I found it very helpful. However the differences are not too understandable for me