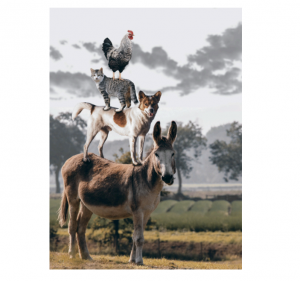
Last updated: 13th Sep, 2024
There are three main types of classification algorithms when dealing with machine learning classification problems: Binary, Multiclass, and Multilabel. In this blog post, we will discuss the differences between them and how they can be used to solve different classification problems. Binary classifiers can only classify data into two categories, while multiclass classifiers can classify data into more than two categories. Multilabel classifiers assign or tag the data to zero or more categories. Let’s take a closer look at each type!
Binary classification & examples
Binary classification is used to represent classification technique in supervised machine learning in which data is classified into two mutually exclusive groups or categories. The two groups can be labeled as 0 and 1, positive and negative, true and false, etc. Binary classification models are trained using a dataset that is labeled with two different classes mentioned earlier. The model then learns to predict the class of the new data points.
Binary classification can be used for a variety of applications, such as spam detection, fraud detection, and medical diagnosis. For example, a binary classification model could be trained to detect whether an email is a spam or not. The model would learn to identify certain keywords and patterns that are associated with spam emails. Once the model is trained, it can then be used to classify new emails as spam or not spam. Another example of a binary classifier is predicting an image as a cat or otherwise. The picture below represents a neural network classifier classifying the image as a cat or otherwise.
Machine learning algorithms that can be used for binary classification include logistic regression, support vector machines (SVM), decision trees, random forest, convolutional neural network (CNN), etc.
Multiclass classification & examples
Multiclass classification is used to represent a classification technique in supervised machine learning in which the data is classified into three or more groups/categories. Unlike binary classification, where the model is only trained to predict one of the two classes for an item, a multiclass classifier is trained to predict one from three or more classes for an item. For example, a multiclass classifier could be used to classify images of animals into different categories such as dogs, cats, and birds. The model would learn to identify certain features that are associated with each animal category. Once the model is trained, it can then be used to classify new images into the correct animal category.
Machine learning algorithms that can be used for multiclass classification include multinomial logistic regression, neural networks, etc. In multinomial logistic regression, softmax function is used to assign one probability per class.
In both binary and multi-class classification, each data sample is assigned one and only one label or class.
Multi-label classification & examples
Multilabel classification is a type of supervised machine learning algorithm that can be used to assign zero or more labels to each data sample. For example, a multilabel classifier could be used to classify an image to consist of both the animal such as a dog and a cat. In order to classify the diagram such as below, it will be a multilabel classifier that will be most suitable. It is an image of the Town Musicians of Bremen, a popular German fairy tale featuring four animals. The image represents a rooster, cat, a dog, and a donkey, with some trees in the background. Treating this as a binary classification problem might not be the most appropriate. Instead, it would be good to build a model that can tag the image with labels such as a cat, a dog, a donkey, and a rooster.
Auto-tagging is a classic example of a multilabel classification problem where a document can be about multiple topics and can be assigned multiple tags. Think of the tags that might be applied to a technical blog, e.g., “machine learning”, “data science”, “statistics”, “programming languages”, and “Python”. A typical article might have 5-6 tags applied because these concepts are correlated. Similarly, an image can have multiple objects and thus, can be assigned multiple labels.
For multilabel classification, algorithms like Decision Trees, Random Forests, k-Nearest Neighbors (k-NN), Neural Networks, and adapted versions of Support Vector Machines (SVMs) are commonly used. These can handle multiple labels simultaneously in a dataset.
Difference between binary, multiclass, and multi-label classification
The following is the difference between each of this classification problems / models:
- What’s the difference between binary and multiclass classification?
- Binary classification involves categorizing data into two distinct groups, like determining if an email is spam or not spam. It’s a straightforward decision between two outcomes. In contrast, multiclass classification involves categorizing data into more than two classes. An example is classifying a set of animals into categories like ‘dog’, ‘cat’, ‘bird’. It involves deciding among multiple outcomes, more complex than a simple binary choice.
- What’s the difference between multiclass and multilabel classification?
- Multiclass classification assigns a single class from multiple options to each instance, like identifying a fruit as either an apple, orange, or banana. Each instance belongs to one and only one class. Multilabel classification, however, allows for multiple classes to be assigned to each instance. For example, a movie could be labeled as both ‘comedy’ and ‘drama’. Here, instances can belong to multiple classes simultaneously, addressing more complex categorization scenarios.
- Multiclass classification assigns a single class from multiple options to each instance, like identifying a fruit as either an apple, orange, or banana. Each instance belongs to one and only one class. Multilabel classification, however, allows for multiple classes to be assigned to each instance. For example, a movie could be labeled as both ‘comedy’ and ‘drama’. Here, instances can belong to multiple classes simultaneously, addressing more complex categorization scenarios.
To summarize, binary classification is a supervised machine learning algorithm that is used to predict one of two classes for an item, while multiclass and multilabel classification is used to predict one or more classes for an item. While a multiclass classifier must assign one and only one class or label to each data sample, a multilabel classifier can assign zero or more classes or labels to the same data sample. Binary classification can be used for a variety of applications such as spam detection and fraud detection, while multiclass and multilabel classification is often used in image recognition and document classification tasks.
- Mathematics Topics for Machine Learning Beginners - July 6, 2025
- Questions to Ask When Thinking Like a Product Leader - July 3, 2025
- Three Approaches to Creating AI Agents: Code Examples - June 27, 2025
I found it very helpful. However the differences are not too understandable for me