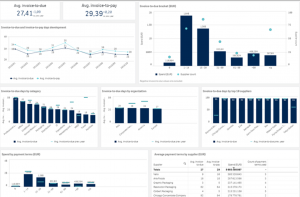
Last updated: 26th Nov, 2023
The procurement analytics applications is seeing tremendous growth in last few years. With so much data available, advancement in data analytics and related technology field, and the need for digital transformation across procurement organizations, it’s important to know how procurement analytics can help you make better business decisions. This blog will cover procurement analytics and key use cases examples from advanced analytics field such as machine learning, AI, generative AI that will be useful for business stakeholders such as category managers, sourcing managers, supplier relationship managers, business analysts/product managers, and data scientists to implement different use cases using machine learning. The use cases around data-driven decision making in different focus areas in procurement will be covered.
Procurement analytics use cases can leverage dashboards to track key performance indicators (KPIs), both leading and lagging, in order to measure the impact of data-driven decisions and related activities. Dashboards are effective tools for monitoring the outcomes and consequences of spend and savings use cases related decisions across various spend categories. These spends and savings can be classified as direct or indirect. The analytics use cases can, thus, be termed as direct procurement and indirect procurement use cases. Direct spending pertains to goods and services directly associated with the core business, such as raw materials for pharmaceutical companies or beverage ingredients for companies like Pepsi. Indirect spending encompasses all other expenses unrelated to the core business. Dashboards, including popular options like Qliksense, provide a foundation for establishing trust in data-driven decision-making within procurement organizations.
Once trust is established, organizations can progress along the analytics value chain and implement AI, Generative AI and machine learning use cases across different spends and savings categories. This advancement enables more advanced and sophisticated analytics to drive procurement strategies. In this blog post, you will gain insights into specific use cases applicable to procurement analytics. By harnessing the power of AI and ML, organizations can unlock newer opportunities for enhancing their procurement practices and achieving better results for the business.
Here is a list of some of the frequently asked questions related to procurement analytics use cases:
- How can the need to improve spend visibility leverage procurement analytics?
- How can procurement analytics help reduce maverick spending?
- How can the spend leakage be tracked using procurement analytics?
- How can procurement analytics help improve supplier performance?
- How can the RFP process be streamlined using procurement analytics?
- How can contract compliance be improved using procurement analytics?
- Can procurement analytics be used to track the catalogue leakage which can help realize cost savings?
- Can price deviations/price variance across different suppliers be tracked using analytics?
- Can procurement analytics help in demand management resulting in cost avoidance?
- How can generative AI (large language models – LLMs) be leveraged as part of procurement strategy to drive operational efficiency and cost savings?
- Can contract management benefit from LLMs based applications?
What are some unique use cases for procurement analytics?
Procurement analytics use cases are centered around the following core process areas in procurement:
- Demand management
- Spend analytics
- Category management
- Supplier relationship management
- Sourcing
- Contract management
- Compliance
- Third-party risk management (TPRM) / supplier risk management
Spend & pricing analytics use cases
Here is the list of use cases related to spend analysis and pricing analytics where advanced analytics (AI/machine learning) can be leveraged:
- Spend analytics: Spend analytics is a set of analytical methods for extracting actionable insights from spends & savings data for driving key procurement decisions and achieving desired outcomes. The insights can be related to spends in different categories, cost savings, cost avoidance, etc. It helps in understanding the maverick spending areas and enables to development a better procurement strategy in relation to managing spending. Some of the key impact areas where AI/machine learning can be leveraged are spend forecasting, savings opportunities, spend anomalies, price variance/deviations, negotiations etc. Tail spend analysis is one of the key aspects of spend analytics. The goal of tail spend analytics is the consolidation of suppliers into preferred suppliers. Check out a related post on spend analytics use cases.
- Purchasing trends analysis: Purchasing trend analysis helps in identifying what products are bought the most by analyzing purchasing data. It’s useful if you want to know how many of a particular product was purchased, and who all bought it including different business divisions across different countries/regions. It also helps in identifying the maverick spending trends. A dashboard can be built to track the trend analysis.
- Cost optimization: Cost optimization techniques can help with figuring out which supplier can provide the best price and why. It also provides a comparison between different suppliers to identify who’s offering better prices or services. Cost modeling including should cost modeling is one of the most used procurement analytics applications. Should cost modeling is a powerful tool that is based on an analysis of the costs of all the inputs / parts to a product or service, including labor, materials, and overhead. This information can then be used to develop a detailed model of the costs associated with producing the item in question. Should cost models can be developed for both individual products and entire manufacturing processes. In addition, should cost models can be used to assess the costs of proposed changes to a product or process before they are implemented. With the advent of generative AI, one can leverage large language models (LLMs) to generate / recommend appropriate inputs or parts of a product and services.
- Pricing benchmarking: Pricing analytics provides information on how much a supplier has raised prices over time, thus helping you identify whether or not it’s reasonable for them to demand an increase in price from their customers. External benchmarking data is very useful in performing such analysis. This information is useful for discussing the next contract with their suppliers. It helps them justify why a new price may be too high or low and come to an agreement on what’s best for both parties. A dashboard can be built to track pricing against a benchmark.
- Payment terms optimization: One of the important areas that can leverage advanced analytics is payment terms optimization. Payment terms optimization in procurement is the process of using AI / machine learning algorithms to optimize payment terms in order to improve organizational cash flow. Payment terms are the conditions under which a buyer agrees to pay for goods or services. Optimizing payment terms can help organizations improve their cash flow by reducing working capital costs and late payment penalties. It can also help to improve supplier relationships by ensuring that suppliers are paid on time. In order to optimize payment terms, organizations need to collect data on past payments, invoices, and supplier performance. This data can then be used to train a machine learning model to predict optimal payment terms for new invoices.
Supplier management use cases
The following are some unique use cases related with supplier relationship management:
- Supply risk management: Supplier risk is a huge concern for businesses, especially when it comes to the procurement of goods and services. There are so many suppliers out there, and it’s impossible to know which ones are reliable and which ones aren’t. The goal of supplier risk management is to identify and mitigate risks that may impact the business, such as cost overruns, quality issues, and delays. In the past, this was a largely manual process, but with the advent of machine learning and AI, businesses are now able to automate supplier risk assessments. By collecting and analyzing data on supplier performance, machine learning algorithms can provide valuable insights into which suppliers are at risk of causing problems.
- Supplier enablement: Supplier enablement is related with leveraging supplier data for effective negotiation with them. The procurement professionals such as category and sourcing managers are able to make the best decision by using insights on a supplier’s performance, financial stability, etc., as well as identifying the best suppliers for specific procurement requirements. It also allows learning about new vendors and untapped geographies which can help in widening their supplier base, thus providing a better customer experience.
- Supplier scorecards: You can train machine learning models to understand which supplier is performing well and what areas need improvement. This information can be used to develop suppliers by helping them improve their processes or products. It may also identify risks associated with certain suppliers, like the one that may not be able to meet the procurement requirements or deliver late. You can prioritize suppliers based on their risk assessment and contract terms, thus helping in effective procurement decisions.
- Supplier performance analysis: Advanced analytics techniques can be used to come up with standard measures / KPIs to compare different suppliers based on their financial health, risk management practices, etc. This information is useful for procurement professionals when they need to choose between multiple suppliers.
Category management use cases
The following are a few unique use cases related to category management:
- Category management: One of the key asks for category managers is to identify the procurement spends and savings trends in different categories. This is where analytics can come to the rescue. It will help category managers understand what products and services are selling well, how often they’re being purchased, etc. The following are a few use cases in category management that can be dealt with data analytics solutions:
- Spend analysis & visibility: Spend analysis and visibility in category management involve leveraging data analytics to gain detailed insights into procurement activities and spending patterns. By analyzing historical spend data, procurement professionals can identify cost-saving opportunities, such as areas of maverick spending, supplier consolidation possibilities, and potential inefficiencies.
- Demand management & market analysis: Demand forecasting and market analysis in category management involve utilizing data analytics to forecast future demand patterns and analyze market trends. By leveraging historical sales data, market data, and predictive analytics models, category managers can accurately anticipate demand fluctuations, optimize inventory levels, and minimize stockouts. Market analysis helps identify emerging suppliers, understand price fluctuations, and adjust sourcing strategies based on changing market dynamics. These data-driven insights enable category management teams to align their strategies with market demand, optimize sourcing decisions, and enhance overall supply chain performance.
- Scenario planning: Businesses need to look at future scenarios before making procurement decisions that will affect them over an extended period of time. Advanced analytics can help understand how various scenarios might affect different aspects of supply such as cost, quality, etc. This allows them to make the best decision based on all possible future outcomes and ensure procurement success in case of any eventuality.
Sourcing use cases
The following represents sourcing related use case:
- E-sourcing: Sourcing manager is required conduct e-sourcing surveys and identify what suppliers are worth targeting based on their performance, cost structures, and more. This is where advanced analytics techniques can be useful. This information is useful for procurement professionals when they’re looking to outsource a specific task or need reliable suppliers in the future.
- Supplier Performance Scorecards: Advanced analytics can be used to develop supplier performance scorecards by analyzing various supplier-related data points such as quality metrics, delivery performance, pricing, and customer satisfaction. This enables sourcing teams to objectively evaluate and compare supplier performance, identify areas for improvement, and make informed decisions on supplier selection, negotiation, and contract renewals.
Other use cases
The following is the list of other uses related to demand management, contract management, etc.
- Demand management analysis: Analysis of demand management is useful in understanding what products are bought when, how many of them were purchased, etc. This helps in identifying what products and services are in demand at that point in time. In turn, this can help in identifying savings opportunities by optimizing the demand for goods and services. The following are some of the key decisions that can leverage predictive analytics:
- Can the demand be delayed? This could result in cost avoidance and help allocate funds to core business.
- Can the demand be internalized? This could result in cost savings.
- Can the demand be forecasted? Demand forecasting is a critical component of demand management in procurement, as it helps businesses to identify future trends and plan accordingly. Machine learning can be used to improve demand forecasting by providing more accurate and timely data. For example, machine learning can be used to identify patterns in customer behavior, rental activity, and social media data. This information can then be used to make more informed decisions about stock levels, pricing, and promotions.
- Can the demand be fulfilled using existing assets within different organization divisions? In other words, can the demand be fulfilled using the reuse of existing assets?
- Contract analytics: Contract analytics is the process of using machine learning to analyze contracts and extract key information. This information can then be used to improve the contract drafting process, identify risk areas, and negotiate better terms. Contract analytics can be used for a variety of different use cases, including contract compliance, fraud detection, and optimal pricing. In each of these cases, contract analytics can help organizations save time and money by automating the analysis of large volumes of data.
- There are several generative AI use cases based on LLMs which can benefit contract management to a great extent. Contract analytics can significantly benefit from the use of Large Language Models (LLMs) like GPT-4 or BERT. These models excel in understanding the nuances of natural language, making them well-suited for dissecting the often complex and dense language found in contracts. They can automate the extraction and interpretation of clauses, identify non-standard terms or risky provisions, and even suggest alternative wording or clauses based on a vast corpus of legal language. Beyond mere keyword matching, LLMs can understand context and semantics, allowing for a deeper analysis that can uncover hidden risks or opportunities for cost savings. For example, an LLM can identify clauses that are unusually favorable or unfavorable to a party and flag them for review, thus aiding in risk mitigation. Additionally, LLMs can assist in comparing contracts across a large dataset to identify industry norms or trends, providing valuable insights for negotiation and strategic decision-making. LLMs can be used to generate different clauses in the contracts.
The following are some of the use cases for contract analytics:- Clause Analytics: Contract analytics can be used to identify clauses that may pose a risk to the company in the future. This information can then be used to negotiate different terms or plan for potential problems. Machine learning models can be trained on the dataset of contracts where the clauses have been labeled. You could use models like decision trees, random forests, or more advanced techniques like neural networks. NLP techniques to identify the semantic boundaries of clauses. Sentence segmentation techniques could be useful here. The semantic relationships between different clauses can be understood as well. You could measure how similar each clause is to a predefined set of templates or to clauses from other contracts. This could help in assessing risk or standard compliance. You can use statistical or machine learning methods to identify clauses that deviate significantly from a set of “normal” contracts. This could be useful for flagging potentially problematic clauses. Automated clause identification and analysis save man-hours that would otherwise be spent reading and interpreting contracts.
- Cost savings opportunities: Contract analytics can be used to identify opportunities for cost savings. For example, if a company usually pays a premium for rush delivery, but contract analytics shows that there is often extra capacity available, they may be able to negotiate a lower price. Automated analysis can flag clauses that might pose a risk, helping to avoid future liabilities or penalties. You can ensure that contracts are in line with industry regulations to avoid costly legal actions. Classification models can help identify non-standard clauses or terms, helping legal teams focus their negotiation efforts more effectively. Some contracts contain clauses that, if activated, could result in cost savings. Examples include volume discounts, early payment discounts, or performance-based incentives.
- Contract analytics can be used to understand the negotiating styles of different companies and individuals. This information can then be used to develop more effective negotiation strategies.
Procurement analytics provides a lot of useful information that procurement professionals can use to make better decisions and achieve their business goals. It helps them understand supplier performance, risk management practices, etc., which saves time and costs in the long run. This is why procurement analytics has become such an important part of modern procurement strategies.
- Retrieval Augmented Generation (RAG) & LLM: Examples - February 15, 2025
- How to Setup MEAN App with LangChain.js - February 9, 2025
- Build AI Chatbots for SAAS Using LLMs, RAG, Multi-Agent Frameworks - February 8, 2025
I found it very helpful. However the differences are not too understandable for me