First Principles Understanding based on Physics
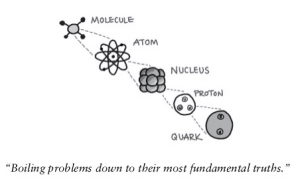
In this post, you will understand the concepts of first principles and first principles thinking based on physics concepts. Let’s jump in right away. In the meanwhile, you could also access one of my other posts on the first principles: First-principles thinking explained with examples. It will help you get started on what are first principles and what is first principle thinking. One of the most fundamental Physics concept to understand the first principle is this: Every physical quantity can be represented as the derived quantity or fundamental quantity. The fundamental quantities, also termed basic quantity, are most basic or fundamental and unique and there are no overlaps between them. …
Precision & Recall Explained using Covid-19 Example
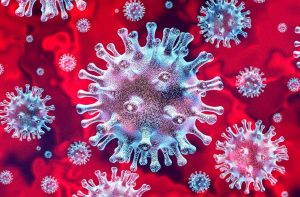
In this post, you will learn about the concepts of precision, recall, and accuracy when dealing with the machine learning classification model. Given that this is Covid-19 age, the idea is to explain these concepts in terms of a machine learning classification model predicting whether the patient is Corona positive or not based on the symptoms and other details. The following model performance concepts will be described with the help of examples. What is the model precision? What is the model recall? What is the model accuracy? What is the model confusion matrix? Which metrics to use – Precision or Recall? Before getting into learning the concepts, let’s look at the data (hypothetical) derived out …
Image Classification & Machine learning
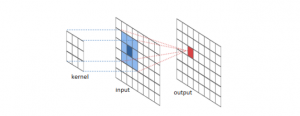
In this post, you will learn about how could image classification problems be solved using machine learning techniques. The following are some of the topics which will be covered: How does the computer learn about an image? How could machine learning be used to classify the images? How does the computer learn about an image? Unlike the human beings, the image has to be converted into numbers for computer to learn about the image. So, the question is how can an image be converted into numbers? The most fundamental element or the smallest building block of an image is a pixel. An image can be represented as a set of …
Actionable Insights Examples – Turning Data into Action
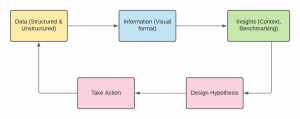
In this post, you will learn about how to turn data into information and then to actionable insights with the help of few examples. It will be helpful for data analysts, data scientists, and business analysts to get a good understanding of what is actionable insight? You will understand aspects related to data-driven decision making. Before getting into the details, let’s understand what is the problem at hand? The school authority is trying to assess and improve the health of students. Here is the question it is dealing with: How could we improve the overall health of the students in the school? We will look into the approach of finding the …
When to use Deep Learning vs Machine Learning Models?
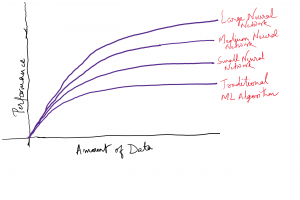
In this post, you will learn about when to go for training deep learning models from the perspective of model performance and volume of data. As a machine learning engineer or data scientist, it always bothers as to can we use deep learning models in place of traditional machine learning models trained using algorithms such as logistic regression, SVM, tree-based algorithms, etc. The objective of this post is to provide you with perspectives on when to go for traditional machine learning models vs deep learning models. The two key criteria based on which one can decide whether to go for deep learning vs traditional machine learning models are the following: …
Most Common Types of Machine Learning Problems
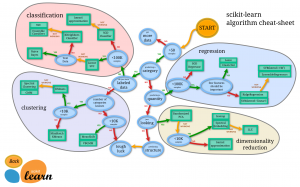
In this post, you will learn about the most common types of machine learning (ML) problems along with a few examples. Without further ado, let’s look at these problem types and understand the details. Regression Classification Clustering Time-series forecasting Anomaly detection Ranking Recommendation Data generation Optimization Problem types Details Algorithms Regression When the need is to predict numerical values, such kinds of problems are called regression problems. For example, house price prediction Linear regression, K-NN, random forest, neural networks Classification When there is a need to classify the data in different classes, it is called a classification problem. If there are two classes, it is called a binary classification problem. …
Historical Dates & Timeline for Deep Learning
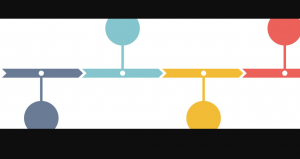
This post is a quick check on the timeline including historical dates in relation to the evolution of deep learning. Without further ado, let’s get to the important dates and what happened on those dates in relation to deep learning: Year Details/Paper Information Who’s who 1943 An artificial neuron was proposed as a computational model of the “nerve net” in the brain. Paper: “A logical calculus of the ideas immanent in nervous activity,” Bulletin of Mathematical Biophysics, volume 5, 1943 Warren McCulloch, Walter Pitts Late 1950s A neural network application by reducing noise in phone lines was developed Paper: Andrew Goldstein, “Bernard Widrow oral history,” IEEE Global History Network, 1997 Bernard …
Great Mind Maps for Learning Machine Learning
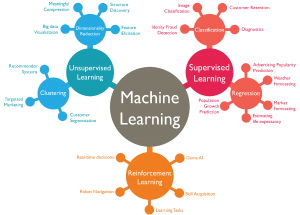
In this post, you will get to look at some of the great mind-maps for learning different machine learning topics. I have gathered these mind maps from different web pages on the Internet. The idea is to reinforce our understanding of different machine learning topics using pictures. You may have heard the proverb – A picture is worth a thousand words. Keeping this in mind, I thought to pull some of the great mind maps posted on different web pages. I would be updating this blog post from time-to-time. If you are a beginner data scientist or an experienced one, you may want to bookmark this page for refreshing your …
Different Types of Distance Measures in Machine Learning
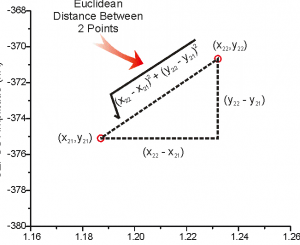
In this post, you will learn different types of distance measures used in different machine learning algorithms such as K-nearest neighbours, K-means etc. Distance measures are used to measure the similarity between two or more vectors in multi-dimensional space. The following represents different forms of distance metrics / measures: Geometric distances Computational distances Statistical distances Geometric Distance Measures Geometric distance metrics, primarily, tends to measure the similarity between two or more vectors solely based on the distance between two points in multi-dimensional space. The examples of such type of geometric distance measures are Minkowski distance, Euclidean distance and Manhattan distance. One other different form of geometric distance is cosine similarity which will discuss …
Introduction to Algorithms & Related Computational Tasks
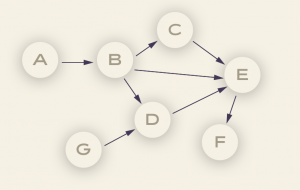
In this post, you will be introduced to some of the important class of algorithms and related computational tasks which could be taken care using these algorithms. Here are some important classes of algorithms which will be briefly discussed in this post: Divide and conquer algorithms Graphs based algorithms Greedy algorithms Dynamic programming Linear programming NP-complete algorithms Quantum algorithms Divide-and-Conquer Algorithms Divide and conquer algorithms are the algorithms which can be used to solve problems using divide and conquer strategy. The following represents the steps of divide-and-conquer algorithms: Breaking it into subproblems that are themselves smaller instances of the same type of problem Recursively solving these subproblems Appropriately combining their …
Machine Learning Terminologies for Beginners
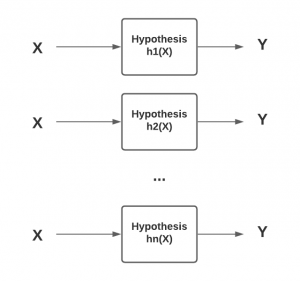
When starting on the journey of learning machine learning and data science, we come across several different terminologies when going through different articles/posts, books & video lectures. Getting a good understanding of these terminologies and related concepts will help us understand these concepts in a nice manner. At a senior level, it gets tricky at times when the team of data scientists / ML engineers explain their projects and related outcomes. With this in context, this post lists down a set of commonly used machine learning terminologies that will help us get a good understanding of ML concepts and also engage with the DS / AI / ML team in …
Machine Learning Free Course at Univ Wisconsin Madison
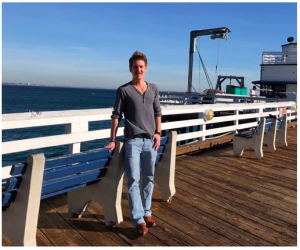
In this post, you will learn about the free course on machine learning (STAT 451) recently taught at University of Wisconsin-Madison by Dr. Sebastian Raschka. Dr. Sebastian Raschka in currently working as an assistant Professor of Statistics at the University of Wisconsin-Madison while focusing on deep learning and machine learning research. The course is titled as “Introduction to Machine Learning”. The recording of the course lectures can be found on the page – Introduction to machine learning. The course covers some of the following topics: What is machine learning? Nearest neighbour methods Computational foundation Python Programming (concepts) Machine learning in Scikit-learn Tree-based methods Decision trees Ensemble methods Model evaluation techniques Concepts of …
Starting on Analytics Journey – Things to Keep in Mind
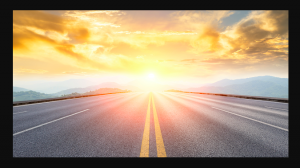
This post highlights some of the key points to keep in mind when you are starting on data analytics journey. You may want to check a related post to assess where does your organization stand in terms of maturity of analytics practice – Analytics maturity model for assessing analytics practice. In the post sighted above, the analytics maturity model defines three different levels of maturity which are as following: Challenged Practitioners Innovators At whichever level you are in terms of maturity of your analytics practice, it may be good idea to understand the following points to come up with data analytics projects. Believe that a lot of prior work is required …
MIT Free Course on Machine Learning (New)
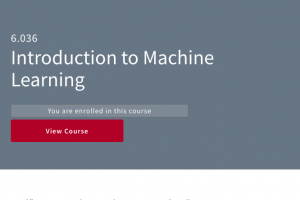
In this post, the information regarding new free course on machine learning launched by MIT OpenCourseware. In case, you are a beginner data scientist or ML Engineer, you will find this course to be very useful. Here is the URL to the free course on machine learning: https://bit.ly/37iNNAA. This course, titled as Introduction to Machine Learning, introduces principles, algorithms, and applications of machine learning from the point of view of modeling and prediction. It includes formulation of learning problems and concepts of representation, over-fitting, and generalization. These concepts are exercised in supervised learning and reinforcement learning, with applications to images and to temporal sequences. Here are some of the key topics for which lectures can be found: …
Gradient Boosting Regression Python Examples
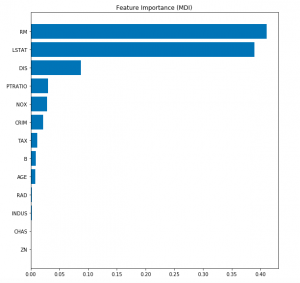
In this post, you will learn about the concepts of Gradient Boosting Regression with the help of Python Sklearn code example. Gradient Boosting algorithm is one of the key boosting machine learning algorithms apart from AdaBoost and XGBoost. What is Gradient Boosting Regression? Gradient Boosting algorithm is used to generate an ensemble model by combining the weak learners or weak predictive models. Gradient boosting algorithm can be used to train models for both regression and classification problem. Gradient Boosting Regression algorithm is used to fit the model which predicts the continuous value. Gradient boosting builds an additive mode by using multiple decision trees of fixed size as weak learners or …
Data Quality Challenges for Analytics Projects
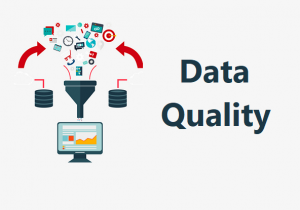
In this post, you will learn about some of the key data quality challenges which you may need to tackle with, if you are working on data analytics projects or planning to get started on data analytics initiatives. If you represent key stakeholders in analytics team, you may find this post to be useful in understanding the data quality challenges. Here are the key challenges in relation to data quality which when taken care would result in great outcomes from analytics projects related to descriptive, predictive and prescriptive analytics: Data accuracy / validation Data consistency Data availability Data discovery Data usability Data SLA Cos-effective data Data Accuracy One of the most important …
I found it very helpful. However the differences are not too understandable for me