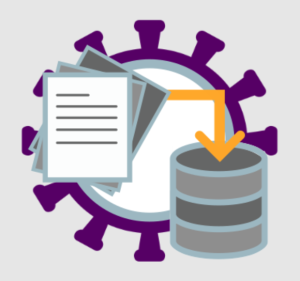
Linear regression is a fundamental machine learning algorithm that helps in understanding the relationship between independent and dependent variables. It is widely used in various fields for predicting numerical outcomes based on one or more input features. To practice and learn about linear regression, it is essential to have access to good quality datasets. In this blog, we have compiled a list of 17 datasets suitable for training linear regression models, available in CSV or easily convertible to CSV (Excel) format. I have also provided a sample Python code you can use to train using these datasets.
List of Dataset for Training Linear Regression Models
The following is a list of 15 dataset which you can use to train linear regression models for learning purpose:
- Boston Housing Dataset
- URL: https://raw.githubusercontent.com/selva86/datasets/master/BostonHousing.csv
- Description: Contains information about the housing values in the suburbs of Boston. Predicting house prices based on 13 features such as CRIM (crime rate), RM (average number of rooms), AGE (proportion of owner-occupied units built prior to 1940), etc.
- Target: Median value of owner-occupied homes.
- Source: Available in the sklearn.datasets module.
- California Housing Dataset
- URL: https://scikit-learn.org/stable/modules/generated/sklearn.datasets.fetch_california_housing.html
- Description: This dataset contains housing data from the 1990 California census. Consists of 8 features including median income, housing median age, average rooms, etc.
- Target: Median house value for California districts.
- Source: Available in the sklearn.datasets module
- Auto MPG Dataset
- URL: https://archive.ics.uci.edu/ml/machine-learning-databases/auto-mpg/auto-mpg.data-original
- Description: Predicting fuel efficiency (MPG) of cars based on 7 features
- Medical Cost Personal Dataset
- URL: https://raw.githubusercontent.com/stedy/Machine-Learning-with-R-datasets/master/insurance.csv
- Description: Predicting medical costs based on patient information
- Wine Quality Dataset
- URL: https://archive.ics.uci.edu/ml/machine-learning-databases/wine-quality/winequality-red.csv
- Description: Predicting wine quality based on physicochemical properties. Contains physicochemical (inputs) and sensory (the output) variables based on wine samples. Includes features like alcohol, sugar, pH level, etc.
- Target: Quality of wine on a scale of 0–10.
- Advertising Dataset
- URL: https://www.statlearning.com/s/Advertising.csv
- Description: Predicting sales based on advertising channels
- Real Estate Valuation Dataset
- URL: https://archive.ics.uci.edu/ml/machine-learning-databases/00477/Real%20estate%20valuation%20data%20set.xlsx
- Description: Predicting house prices based on real estate features
- Diabetes Dataset
- URL: https://raw.githubusercontent.com/plotly/datasets/master/diabetes.csv
- Description: Predicting disease progression based on diagnostic measurements. Contains ten baseline variables, age, sex, body mass index, average blood pressure, and six blood serum measurements.
- Target: A quantitative measure of disease progression one year after baseline.
- Iris Dataset
- URL: https://scikit-learn.org/stable/auto_examples/datasets/plot_iris_dataset.html
- Description: Though typically used for classification, it can be adapted for regression.
- Features: Sepal length, sepal width, petal length, petal width.
- Target: Typically species, but can be adapted to a continuous variable for regression.
- Concrete Compressive Strength
- URL: https://archive.ics.uci.edu/ml/machine-learning-databases/concrete/compressive/Concrete_Data.xls
- Description: Predicting concrete compressive strength based on its ingredients
- Appliances Energy Prediction
- URL: https://archive.ics.uci.edu/ml/machine-learning-databases/00374/energydata_complete.csv
- Description: Predicting energy consumption of appliances and lights based on various factors
- Video Game Sales
- URL: https://www.kaggle.com/gregorut/videogamesales
- Description: Predicting global video game sales based on platform, genre, etc.
- Abalone Dataset
- URL: https://archive.ics.uci.edu/ml/machine-learning-databases/abalone/abalone.data
- Description: Predicting abalone age based on physical measurements
- Student Performance Dataset
- URL: https://archive.ics.uci.edu/ml/machine-learning-databases/00320/student.zip
- Description: Predicting student performance based on demographic and school-related factors
- Bike Sharing Dataset
- URL: https://archive.ics.uci.edu/ml/machine-learning-databases/00275/Bike-Sharing-Dataset.zip
- Description: Predicting bike rental count based on time and weather factors
- Graduate Admissions Dataset
- URL: https://raw.githubusercontent.com/AdiPersonalWorks/Random/master/student_scores%20-%20student_scores.csv
- Description: Predicting graduate admission chance based on various factors
- Forest Fires Dataset
- URL: https://archive.ics.uci.edu/ml/machine-learning-databases/forest-fires/forestfires.csv
- Description: Predicting the burned area of forest fires based on meteorological data
Python Code for Training Linear Regression Models using CSV Dataset
The following is a sample Python code snippet demonstrating how to train a linear regression model using the Boston Housing Dataset. The following Python code snippet imports the necessary libraries, loads the Boston Housing Dataset, splits the data into training and testing sets, trains a linear regression model, makes predictions on the test set, and calculates the performance metrics (Mean Squared Error, Root Mean Squared Error, and R-squared) to evaluate the model’s performance.
The above code can be used as it is to read CSV files from URL and train the linear regression models. However, if the file is downloaded to your local drive, you may try with the following Python code:
import pandas as pd
import numpy as np
from sklearn.model_selection import train_test_split
from sklearn.linear_model import LinearRegression
from sklearn.metrics import mean_squared_error, r2_score
# Load the Boston Housing Dataset
url = "https://raw.githubusercontent.com/selva86/datasets/master/BostonHousing.csv"
df = pd.read_csv(url)
# Separate the features (X) and target (y)
X = df.drop('medv', axis=1)
y = df['medv']
# Split the dataset into training and testing sets
X_train, X_test, y_train, y_test = train_test_split(X, y, test_size=0.2, random_state=42)
# Train the linear regression model
lr = LinearRegression()
lr.fit(X_train, y_train)
# Make predictions on the test set
y_pred = lr.predict(X_test)
# Calculate the performance metrics
mse = mean_squared_error(y_test, y_pred)
rmse = np.sqrt(mse)
r2 = r2_score(y_test, y_pred)
# Print the performance metrics
print(f"Mean Squared Error: {mse:.2f}")
print(f"Root Mean Squared Error: {rmse:.2f}")
print(f"R-squared: {r2:.2f}")
# Load the dataset from local drive
file_path = "path/to/your/csv_file.csv"
df = pd.read_csv(file_path)
# Replace 'feature_columns' and 'target_column' with appropriate column names
feature_columns = ['feature1', 'feature2', 'feature3']
target_column = 'target'
# Separate the features (X) and target (y)
X = df[feature_columns]
y = df[target_column]
Conclusion
In this blog post, we compiled a diverse list of 17 datasets (CSV, Excel) suitable for training and practicing linear regression models. These datasets cover a broad range of topics, from predicting house prices to forecasting energy consumption. Using these datasets, you can not only gain hands-on experience with linear regression but also explore various aspects of the machine learning process, such as data preprocessing, feature selection, model evaluation, and improvement.
By working with these datasets, you’ll deepen your understanding of the assumptions and limitations of linear regression models and learn how to apply this fundamental algorithm effectively. Additionally, you’ll develop the skills necessary to tackle real-world problems using linear regression, paving the way for you to explore more complex machine learning techniques.
- Three Approaches to Creating AI Agents: Code Examples - June 27, 2025
- What is Embodied AI? Explained with Examples - May 11, 2025
- Retrieval Augmented Generation (RAG) & LLM: Examples - February 15, 2025
I found it very helpful. However the differences are not too understandable for me