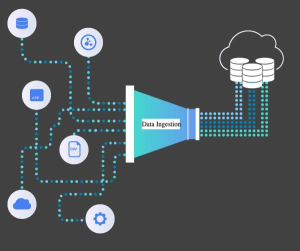
Last updated: 17th Nov, 2023
Data ingestion is the process of moving data from its original storage location to a data warehouse or other database for analysis. Data engineers are responsible for designing and managing data ingestion pipelines. Data can be ingested in different modes such as real-time, batch mode, etc. In this blog, we will learn the concepts about different types of data ingestion with the help of examples.
What is Data Ingestion?
Data ingestion is the foundational process of importing, transferring, loading, and processing data from various sources into a storage medium where it can be accessed, used, and analyzed by an organization. It’s akin to the first step in a complex journey of data transformation and utilization. The data ingested can range from small quantities to vast streams of real-time data and can originate from diverse sources like databases, SaaS platforms, IoT devices, and more.
In the era of big data, the ability to efficiently and accurately ingest data is critical. It lays the groundwork for data analysis, business intelligence, and decision-making processes. Proper data ingestion ensures that data is reliable, up-to-date, and readily available for various applications, such as machine learning models, reporting tools, and analytical frameworks.
The data source can be either structured or unstructured. Structured data sources are typically found in relational databases, while unstructured data sources include text files, images, and social media data. In order to ingest data from a data source, a data ingestion tool must be used. This tool typically uses some form of Extract, Transform, and Load (ETL) process to extract the data from the source, transform it into a format that can be loaded into the target data storage system, and then load it into that system. Once the data is ingested, it can then be accessed and analyzed by various data analytics tools.
The data can come from a variety of sources, including databases, NoSQL data stores, application logs, and social media feeds. In order to ingest data efficiently, it is important to have a clear understanding of the data’s structure and how it will be used. For example, data that will be used for analytics purposes may need to be cleansed and transformed before it can be loaded into the data warehouse. Once the data has been ingested, it can be stored in its raw form or processed further for analytics. Data ingestion is a critical part of any data management strategy.
Data ingestion in the data pipeline
The role of data ingestion is pivotal in the larger scheme of data processing. It forms the initial phase of the data pipeline, which typically includes stages like ingestion, storage, processing, analysis, and visualization. Ingestion is about getting the data into the system; what follows is a series of transformations and analyses that turn raw data into actionable insights.
Key Characteristics of Data Ingestion:
- Volume: It deals with different volumes of data, from few kilobytes in small businesses to petabytes in large-scale operations.
- Velocity: Data ingestion processes vary from handling batch data, which is collected over time and processed periodically, to real-time data that is processed almost immediately as it arrives.
- Variety: Data comes in all shapes and forms, from structured data like CSV files and SQL databases to unstructured data like emails, videos, and social media posts.
- Veracity: Ensuring the accuracy and consistency of ingested data is crucial, as it affects the quality of downstream analytics.
Key Challenges in Data Ingestion
Data ingestion might sound straightforward, but it poses several challenges:
- Integrating Varied Data Sources: Each data source can have different formats, structures, and interfaces.
- Scaling: As data volume grows, the ingestion process must scale accordingly without loss of performance.
- Data Quality: Ensuring that the ingested data maintains its quality and integrity is vital.
- Security and Compliance: Safeguarding sensitive data and complying with various data protection regulations is a critical aspect of the ingestion process.
What are some popular data ingestion tools?
The following are few examples of data ingestion tools:
- Apache Flume: Apache Flume is a distributed, reliable, and available system for efficiently collecting, aggregating, and moving large amounts of streaming data from various sources to a centralized data store. Flume is highly configurable and extensible, with a large number of built-in sources, channels, and sinks. It is also easy to use, with a simple yet powerful DSL for defining data flows.
- Apache Kafka: Apache Kafka is an open-source stream processing platform that can be used for a wide range of streaming data applications. Kafka is designed to handle high throughput and low latency streaming data, and provides built-in support for robust message delivery semantics such as exactly-once delivery and at-least-once delivery. Kafka also has strong support for message replay and fault tolerance, making it an ideal platform for building streaming data applications.
- Amazon Kinesis: Amazon Kinesis is a cloud-based stream processing service that can be used to process large amounts of streaming data in real time. Kinesis offers built-in support for both batch and real-time data processing, and provides developers with the ability to build custom applications using the Kinesis API. Kinesis is also highly scalable and fault tolerant, making it an ideal platform for building streaming data applications.
- Apache Storm: Apache Storm is a free and open source distributed real time computation system that can be used to process large amounts of streaming data in real time. Storm is designed to be highly scalable and fault tolerant, making it an ideal platform for building streaming data applications. Storm also offers a simple yet powerful API that makes it easy to develop custom applications.
There are primarily two types of data ingestion. They are as following:
- Real-time or streaming data ingestion
- Batch data ingestion
Real-time or Streaming Data Ingestion
Real-time data ingestion means that data is acquired and processed as soon as it becomes available, without any delay. In real-time data ingestion, data is transferred as it is generated. This is important for applications that need to take immediate action based on new data, such as monitoring or control systems. This type of ingestion is typically used for event-based data, such as log files, financial transactions, and sensor readings. There are many different techniques for real-time data ingestion, depending on the data source. Common data sources include streams (such as sensors or social media feeds), files (such as logs or transaction records), and databases (such as customer data). The most important thing for real-time data ingestion is timely availability of data, so the technique used must be able to handle high data volumes and meet latency requirements.
Batch Data Ingestion
Batch data ingestion is the process of taking data from a data source and importing it into a system in batches. This can be contrasted with real-time data ingestion, which involves taking data from a data source and importing it into a system as it is generated. Batch data ingestion is typically used when data sources are not able to provide data in real time, or when data needs to be processed before it can be ingested into a system. For example, if data needs to be cleansed or transformed before it can be used, batch data ingestion would be the appropriate approach. Batch data ingestion can also be used when there is a large volume of data that needs to be imported all at once, such as historical data. In general, batch data ingestion is less complex and easier to manage than real-time data ingestion, but it can take longer to import data using this approach.
Batch data ingestion is usually done on a schedule, such as once per day. Data sources can be internal or external. Internal data sources are usually databases, while external data sources can be anything from sensors to social media feeds.
Conclusion
Data ingestion is the process of bringing data into your system for storage or analysis. There are two main types of data ingestion: real-time or streaming, and batch. Real-time or streaming ingestion refers to data that is brought in as it is created, while batch ingestion involves gathering data all at once and loading it into the system. Both methods have their own benefits and drawbacks, so it’s important to understand which one will work best for your needs. If you would like to learn more about data ingestion or need help deciding which method is right for you, please let me know. I’d be happy to help!
- Completion Model vs Chat Model: Python Examples - June 30, 2024
- LLM Hosting Strategy, Options & Cost: Examples - June 30, 2024
- Application Architecture for LLM Applications: Examples - June 25, 2024
Leave a Reply