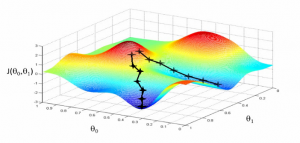
This article represents some of the most common machine learning tasks that one may come across while trying to solve machine learning problems. Also listed is a set of machine learning methods that could be used to resolve these tasks. Please feel free to comment/suggest if I missed mentioning one or more important points. Also, sorry for the typos.
You might want to check out the post on what is machine learning?. Different aspects of machine learning concepts have been explained with the help of examples. Here is an excerpt from the page:
Machine learning is about approximating mathematical functions (equations) representing real-world scenarios. These mathematical functions are also referred to as “mathematical models” or just models.
Following are the key machine learning tasks briefed later in this article:
- Regression
- Classification
- Clustering
- Transcription
- Machine translation
- Anomaly detection
- Synthesis & sampling
- Estimation of probability density and probability mass function
- Similarity matching
- Co-occurrence grouping
- Causal modeling
- Link profiling
Following are the key development phases that are used to solve the different tasks listed above. These form the key phases of the machine learning models’ (MLM) development lifecycle.
- Data gathering
- Data preprocessing
- Exploratory data analysis (EDA)
- Feature engineering including feature creation/extraction, feature selection, dimensionality reduction
- Training machine learning models
- Model / Algorithm selection
- Testing and matching
- Model monitoring
- Model retraining
Machine Learning Tasks
The following are some of the key tasks which can be performed using machine learning models:
- Regression: Regression tasks mainly deal with the estimation of numerical values (continuous variables). Regression task in machine learning is a supervised machine learning technique used to predict the values of a given target variable based on the input of one or more independent variables. It is a task of fitting a mathematical model to observed data points, where the objective is to minimize the sum of squared errors between the observed data and the predicted values. In regression tasks, we use linear and non-linear models to build our predictive models. Linear models have a basic assumption that there exists a linear relationship between the input and output variables, while non-linear models do not rely on any such assumptions. The goal of linear regression is to find the best fit line for our data whereas in non-linear regression, we try to identify complex relationships within our dataset. In addition to prediction problems, regression tasks can also be used for inference problems where we want to understand how variables interact with each other. For example, you might use a linear regression model in order to investigate how two or more independent variables influence a dependent variable such as salary or job satisfaction. This allows us to gain insights about how changes in one independent variable affects another independent variable or even the dependent variable itself without having to make any changes directly ourselves.
Some of the examples include estimation of housing price, product price, stock price etc. Some of the following ML methods could be used for solving regressions problems:-
- Kernel regression (Higher accuracy)
- Gaussian process regression (Higher accuracy)
- Regression trees
- Linear regression
- Support vector regression
- LASSO / Ridge
- Deep learning
- Random forests
-
- Classification: Classification tasks are simply related to predicting a category of data (discrete variables). Classification is a type of machine learning task that involves identifying which group or category an item belongs to, based on certain features or characteristics. It’s one of the most common types of supervised learning techniques and it’s used for everything from banking fraud detection to face recognition. At its core, classification is the process of sorting items into two or more mutually exclusive groups, often called classes. The goal is to correctly assign a given item to the right class based on its features. So in order to do this, the machine must learn how to differentiate between different classes using these features. This can be done by pre-labeling data so that the computer knows which class each item belongs to, then training it with different algorithms until it learns how to accurately identify each class. Simply speaking, a classification task results in the model which, given a new individual, determines which class that individual belongs to. A closely related task is scoring or class probability estimation. A scoring model applied to an individual produces, instead of a class prediction, a score representing the probability (or some other quantification of likelihood) that that individual belongs to each class. One of the most common examples is predicting whether or not an email if spam or ham. Some of the common use cases could be found in the area of healthcare such as whether a person is suffering from a particular disease or not. It also has its application in financial use cases such as determining whether a transaction is a fraud or not. You might want to check this page on real-world examples of classification models, machine learning classification models real-life examples. The ML methods such as the following could be applied to solve classification tasks:
- Kernel discriminant analysis (Higher accuracy)
- K-Nearest Neighbors (Higher accuracy)
- Artificial neural networks (ANN) (Higher accuracy)
- Support vector machine (SVM) (Higher accuracy)
- Random forests (Higher accuracy)
- Decision trees
- Boosted trees
- Logistic regression
- naive Bayes
- Deep learning
- Clustering: Clustering is a commonly used machine learning task in which data points are grouped into clusters, or groups of closely related data points. It is an unsupervised approach that does not require labeled data and can be used to identify patterns or similarities within a dataset. Clustering has many applications ranging from customer segmentation, market segmentation, image segmentation, document classification, and more. At its core, clustering is a process of partitioning a set of objects into distinct groups such that the elements in each group are similar to each other while those belonging to different groups are very dissimilar. The following are four different type of clustering algorithms:
- Prototype based clustering (K-means)
- Hierarchical clustering
- DBSCAN (Density based spatial clustering of applications with noise)
- Distribution based clustering
- Similarity matching: Similarity matching task in machine learning is a task in which machines are trained to match items based on their similarity. This type of task can be used for a wide range of applications, such as natural language processing, image recognition and recommendation systems. In order for a machine learning system to be able to perform well at this type of task, it needs to be able to learn how to distinguish between similar and dissimilar items. This can be done by creating feature vectors from examples of known data points, then using that information as training data so that the machine can make accurate predictions when presented with new data points. Once trained, similarity matching algorithms can be used in many ways such as providing recommendations or helping with search engine optimization. For example, if you were looking for a particular product online but couldn’t find it through traditional search methods, similarity matching could help by presenting other products that closely match your desired item based on their features and characteristics. Similarly, recommendation systems use such algorithms to suggest items that users may like based on their past preferences or those of other users with similar interests.
- Co-occurrence grouping: Co-occurrence grouping tasks are also called frequent itemset mining, association rule discovery, and market-basket analysis tasks. In this task, association between entities are found based on transactions involving them. for example, what items are commonly purchased together? The difference between clustering and co-occurrence grouping is that in clustering, the similarity between objects is found based on the objects’ attributes while in co-occurrence grouping, the similarity of objects is found based on them appearing together in transactions. For example, the purchase records from a supermarket may uncover the association that bread is purchased together with eggs much more frequently than expected.
- Multivariate querying: Multivariate querying is about querying or finding similar objects. Some of the following ML methods could be used for such problems:
- Nearest neighbors
- Range search
- Farthest neighbors
- Probability density and mass function estimation: Probability density function estimation problems are related to finding the likelihood or frequency of objects. In probability and statistics, density estimation is the construction of an estimate, based on observed data, of an unobservable underlying probability density function. Some of the following ML methods could be used for solving density estimation tasks:
- Kernel density estimation (Higher accuracy)
- Mixture of Gaussians
- Density estimation tree
- Machine translation: Machine translation is the process of translating text from one language to another using machine learning algorithms. There are many different machine translation tasks, such as machine translation of documents, machine translation of the speech, and machine translation of web pages. Deep learning models have achieved state-of-the-art results on many machine translation tasks. For example, deep learning models have been used to machine translate Web pages from English to Chinese with close to human-level accuracy. In addition, deep learning models have been used to machine translate speech from English to French with close to human-level accuracy. Machine translation is an important application of machine learning that has the potential to change the way people communicate with each other.
- Anomaly detection: Anomaly detection is the process of identifying unusual patterns in data that do not conform to expected behavior. It is often used in a wide range of applications, such as detecting fraudulent activity in financial data, detecting malicious behavior in network traffic data, and identifying equipment malfunctions in sensor data. Anomaly detection can be performed using a variety of machine learning models, such as density-based methods, cluster-based methods, and rule-based methods. Each of these methods has its own strengths and weaknesses, so it is important to select the right model for the particular application. Anomaly detection using machine learning is a complex process, but it can be extremely effective at identifying rare events that would otherwise be overlooked.
- Synthesis & sampling: Synthesis and sampling are essential tasks in deep learning and machine learning. They are used to generate new data from existing data or to select a representative subset of data for further analysis. Synthesis and sampling are often used together, in order to create a more diverse and representative dataset. Synthesis can be used to generate new data points, by extrapolating from existing data points. For example, if we have a dataset of images of animals, we can use synthesis to generate new images of animals that are similar to the ones in the dataset. Sampling can be used to select a subset of data that is representative of the entire dataset. For example, if we have a dataset of images of animals, we can use sampling to select a subset of images that represents all the different animal types in the dataset.
- Transcription: Transcription tasks are those that involve converting audio or video recordings or images having text into written text. They are commonly used in fields such as journalism, academia, and medicine. In recent years, transcription tasks have been automated to some degree using machine learning algorithms. Deep learning models can be trained to transcribe audio recordings with a high degree of accuracy. However, these models require a large amount of data to train on, and they often struggle with background noise and accents. As a result, transcription is still largely a manual task. Professional transcriptionists use their knowledge of the language and their attention to detail to produce accurate transcription services.
- Causal modeling: Causal modeling is a type of machine learning task that aims to infer the causes and effects of certain conditions or variables. It is an important tool for researchers in fields such as epidemiology, economics, psychology, marketing, and political science. In causal modeling, data is used to make inferences about the relationships between variables. The goal is to identify which variables are causing certain outcomes and how they are related. For example, a researcher may want to determine the causal relationship between smoking cigarettes and lung cancer. Does smoking cigarettes is related to lung cancer?
- Dimensionality reduction (feature extraction): As per the Wikipedia page on Dimension reduction, Dimension reduction is the process of reducing the number of random variables under consideration, and can be divided into feature selection and feature extraction. Following are some ML methods that could be used for dimension reduction:
- Manifold learning/KPCA (Higher accuracy)
- Principal component analysis
- Independent component analysis
- Gaussian graphical models
- Non-negative matrix factorization
- Compressed sensing
- Link prediction: Link prediction task in machine learning is a task that focuses on identifying potential connections between entities that are not yet connected. It is used to predict relationships between entities, such as customers, products, authors, and more. The goal of link prediction is to build a model that can accurately identify connections between entities in a dataset. In the past, link prediction has primarily been used for social network analysis where it’s often used to suggest friends or followers for users of a social network platform. However, it can also be applied to other types of data such as customer transactions data or scientific research papers. Link prediction models are also often used in recommendation systems to recommend items to customers based on their past behavior or preferences. Link prediction can also estimate the strength of a link. For example, for recommending movies to customers, link prediction can be used to create a graph between customers and the movies they’ve watched or rated. Within the graph, those potential links (strong link) are searched that should exist between customers and movies.
Development phases of MLM Development Lifecycle
Following are the most common machine learning models development phases that one could come across most frequently while solving different machine learning tasks:
- Data Gathering: Any machine learning problem requires a lot of data for training/testing purposes. Identifying the right data sources and gathering data from these data sources is the key. Data could be found from databases, external agencies, the internet, etc.
- Data Preprocessing: Before starting training the models, it is of utmost importance to prepare data appropriately. As part of data preprocessing, some of the following is done:
- Data cleaning: Data cleaning requires one to identify attributes having not enough data or attributes which are not have variance. These data (rows and columns) need to be removed from the training data set.
- Missing data imputation: Handling missing data using data imputation techniques such as replacing missing data with mean, median, or mode. Here is my post on this topic: Replace missing values with mean, median or mode
- Exploratory Data Analysis (EDA): Once data is preprocessed, the next step is to perform exploratory data analysis to understand data distribution and relationships between/within the data. Some of the following are performed as part of EDA:
- Correlation analysis
- Multicollinearity analysis
- Data distribution analysis
- Feature Engineering: Feature engineering is one of the critical tasks which would be used when building machine learning models. Feature engineering is important because selecting the right features would not only help build models of higher accuracy but also help achieve objectives related to building simpler models, reducing overfitting, etc. Feature engineering includes tasks such as deriving features from raw features, identifying important features, feature extraction, and feature selection. The following are some of the techniques which could be used for feature selection:
- Filter methods help in selecting features based on the outcomes of statistical tests. The following are some of the statistical tests which are used:
- Pearson’s correlation
- Linear discriminant analysis (LDA)
- Analysis of Variance (ANOVA)
- Chi-square tests
- Wrapper methods help in feature selection by using a subset of features and determining the model accuracy. The following are some of the algorithms used:
- Forward selection
- Backward elimination
- Recursive feature elimination
- Regularization techniques penalize one or more features appropriately to come up with most important features. The following are some of the algorithms used:
- LASSO (L1) regularization
- Ridge (L2) regularization
- Elastic net regularization
- Regularization with classification algorithms such as Logistic regression, SVM, etc.
- Filter methods help in selecting features based on the outcomes of statistical tests. The following are some of the statistical tests which are used:
- Training Models: Once some of the features are determined, then comes training models with data related to those features. One popular algorithm used for while training regression models is Gradient Descent which helps us to find optimal parameter values in order to minimize our cost function (also known as error rate). In this method, we start with random initial parameter values and then gradually update them by taking small steps until we reach an optimal solution. This iterative process helps us reduce error rates over time and ultimately provide better predictions for our target variable.
- Model selection / Algorithm selection: Many times, there are multiple models which are trained using different algorithms. One of the important tasks is to select the most optimal models for deploying them in production. Hyperparameter tuning is the most common task performed as part of model selection. Also, if there are two models trained using different algorithms which have similar performance, then one also needs to perform algorithm selection.
- Testing and matching: Testing and matching tasks relate to comparing data sets. Following are some of the methods that could be used for such kinds of problems:
- Minimum spanning tree
- Bipartite cross-matching
- N-point correlation
- Model monitoring: Once the models are trained and deployed, they require to be monitored at regular intervals. Monitoring models require the processing actual values and predicted values and measuring the model performance based on appropriate metrics.
- Model retraining: In case, the model performance degrades, the models are required to be retrained. The following gets done as part of model retraining:
- New features get determined
- New algorithms can be used
- Hyperparameters can get tuned
- Model ensembles may get deployed
Latest posts by Ajitesh Kumar (see all)
- Mathematics Topics for Machine Learning Beginners - July 6, 2025
- Questions to Ask When Thinking Like a Product Leader - July 3, 2025
- Three Approaches to Creating AI Agents: Code Examples - June 27, 2025
I found it very helpful. However the differences are not too understandable for me