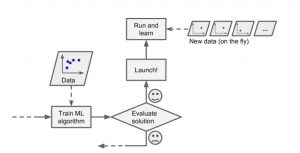
In this post, you will learn about the concepts and differences between online and batch or offline learning in relation to how machine learning models in production learn incrementally from the stream of incoming data or otherwise. It is one of the most important aspects of designing machine learning systems. Data science architects would require to get a good understanding of when to go for online learning and when to go for batch or offline learning.
Why online learning vs batch or offline learning?
Before we get into learning the concepts of batch and on-line or online learning, let’s understand why we need different types of models training or learning in the first place.
The key aspect to understand is the data. When the data is limited and comes at regular intervals, we can adopt both types of learning based on the business requirements. However, when we talk about big data, the aspects such as the following become the key considerations when deciding the model of learning:
- Volume: The data comes in large volumes. There are a lot of preprocessing steps that need to be done in order to make data available for both training and prediction. This would thus require IT infrastructures, software systems, and appropriate expertise and experience to do the data processing.
- Velocity: As like in the case of the high volume of data, the data coming at high speed (for example, tweets) can also become key criteria.
- Variety: Similar to volume and variety, the data can become of a different variety. For example, data for aggregator services such as Uber, and Airbnb.
In order to manage big data while delivering on the business requirements, an appropriate selection of learning methods such as batch learning or online learning is made.
What is Batch or Offline Learning?
Batch learning represents the training of machine learning models in a batch manner. In other words, batch learning represents the training of the models at regular intervals such as weekly, bi-weekly, monthly, quarterly, etc. In batch learning, the system is not capable of learning incrementally. The models must be trained using all the available data every single time. The data gets accumulated over a period of time. The models then get trained with the accumulated data from time to time at periodic intervals. This model training takes a lot of time and computing resources. Hence, it is typically done offline. After the models are trained, they are launched into production and they run without learning anymore. Batch learning is also called offline learning. The models trained using batch or offline learning are moved into production only at regular intervals based on the performance of models trained with new data.
Building offline models or models trained in a batch manner requires training the models with the entire training data set. Improving the model performance would require re-training all over again with the entire training data set. These models are static in nature which means that once they get trained, their performance will not improve until a new model gets re-trained. The model’s performance tends to decay slowly over time, simply because the world continues to evolve while the model remains unchanged. This phenomenon is often called model rot or data drift. The solution is to regularly retrain the model on up-to-date data. Offline models or models trained using batch learning are deployed in the production environment by replacing the old model with the newly trained model.
For the model to learn about the new data, the model would need to be trained with all the data from scratch. The old model would then need to be replaced with the new model. And, as part of batch learning, the whole process of training, evaluating, and launching a machine learning system gets automated. The following picture represents the automation of batch learning. Model training using the full set of data can take many hours. Thus, it is recommended to run the batch frequently rather than weekly as training on the full set of data would require a lot of computing resources (CPU, memory space, disk space, disk I/O, network I/O, etc.)
There can be various reasons why we can choose to adopt batch learning for training the models. Some of these reasons are the following:
- The business requirements do not require frequent learning of models.
- The data distribution is not expected to change frequently. Therefore, batch learning is suitable.
- The software systems (big data) required for real-time learning is not available due to various reasons including the cost.
- The expertise required for creating the system for incremental learning is not available.
If the models trained using batch learning needs to learn about new data, the models need to be retrained using the new data set and replaced appropriately with the model already in production based on different criteria such as model performance. The whole process of batch learning can be automated as well. The disadvantage of batch learning is it takes a lot of time and resources to re-training the model.
The criteria based on which the machine learning models can be decided to train in a batch manner depends on the model performance. Red-amber-green statuses can be used to determine the health of the model based on the prediction accuracy or error rates. Accordingly, the models can be chosen to be retrained or otherwise. The following stakeholders can be involved in reviewing the model performance and leveraging batch learning:
- Business/product owners
- Product managers
- Data science architects
- Data scientists
- ML engineers
What is Online Learning?
In online learning, the training happens in an incremental manner by continuously feeding data as it arrives or in a small group / mini batches. Each learning step is fast and cheap, so the system can learn about new data on the fly, as it arrives.
Online learning is great for machine learning systems that receive data as a continuous flow (e.g., stock prices) and need to adapt to change rapidly or autonomously. It is also a good option if you have limited computing resources: once an online learning system has learned about new data instances, it does not need them anymore, so you can discard them (unless you want to be able to roll back to a previous state and “replay” the data) or move the data to another form of storage (warm or cold storage) if you are using the data lake. This can save a huge amount of space and cost. The diagram given below represents online learning.
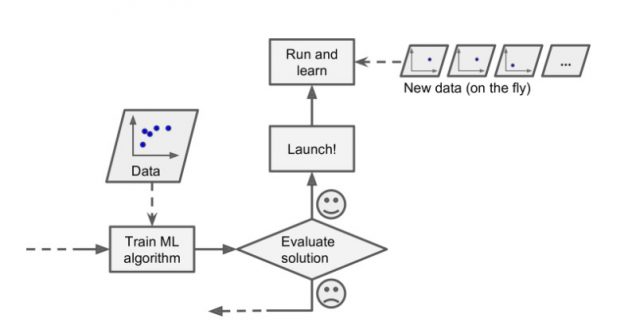
Fig 1. Online learning – Machine Learning System
Online learning algorithms can also be used to train systems on huge datasets that cannot fit in one machine’s main memory (this is also called out-of-core learning). The algorithm loads part of the data runs a training step on that data and repeats the process until it has run on all of the data.
One of the key aspects of online learning is the learning rate. The rate at which you want your machine learning to adapt to new data set is called the learning rate. A system with a high learning rate will tend to forget the learning quickly. A system with a low learning rate will be more like batch learning.
One of the big disadvantages of an online learning system is that if it is fed with bad data, the system will have bad performance and the user will see the impact instantly. Thus, it is very important to come up with appropriate data governance strategy to ensure that the data fed is of high quality. In addition, it is very important to monitor the performance of the machine learning system in a very close manner.
Data governance needs to be put in place across different levels such as the following when choosing to go with online learning:
- Data ingestion
- ETL pipelines
- Feature extraction
- Predictions
The following are some of the challenges for adopting an online learning method:
- Data governance
- Model governance includes appropriate algorithm and model selection on-the-fly
Online models require only a single deployment in the production setting and they evolve over a period of time. The disadvantage that the online models have is that they don’t have the entire dataset available for the training. The models are trained in an incremental manner based on the assumptions made using the available data and the assumptions at times can be sub-optimal.
- Logistic Regression in Machine Learning: Python Example - April 26, 2024
- MSE vs RMSE vs MAE vs MAPE vs R-Squared: When to Use? - April 25, 2024
- Gradient Descent in Machine Learning: Python Examples - April 22, 2024
This is great article for understanding Batch learning and Online learning. Easy explanation.
I’d like to point out that the third point in the reasons for choosing Batch learning is incorrect.
“The software systems (big data) required for batch learning is not available due to ….”
Thank you Rahul. That was a good catch. Changed it appropriately.