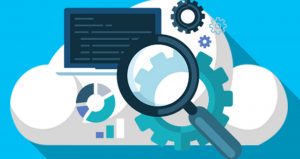
This post represents thoughts on what would it look like planning unit tests for machine learning models. The idea is to perform automated testing of ML models as part of regular builds to check for regression related errors in terms of whether the predictions made by certain set of input data vectors does not match with expected outcomes. This brings up some of the following topics for discussion:
- Why unit testing for machine learning models?
- What would unit tests for machine learning models mean?
- Data coverage or code coverage?
Why unit testing for Machine Learning models?
Once a model is built, the challenge is to monitor the performance metrics of the models and take appropriate action when the performance degrades below a certain threshold. There are different ways in which performance could be monitored. The primary of them is monitoring performance related metrics such as precision, recall, RMSE etc. However, there are scenarios where one would want to monitor the predictions accuracy in relation to some of the following:
- Performance (prediction accuracy) related with different class (slices) of input data vectors;
- Performance related to features importance vis-a-vis predictions; Are there changes in feature importance?
In order to test the ML models against some of the above criteria, the need for some kind of testing comes into picture. This is where one could consider some sort of traditional unit testing methods and how could they be applied to machine learning models.
What is Unit testing for Machine Learning models?
In order to understand unit testing for ML models, one would need to understand what might “Unit” stand for? And, what might “Unit testing” mean?
What might “Unit” stand for?
Units may be represented as the different sets of input data vectors which when fed into the ML models ends up making a specific class of predictions. As part of unit testing, this class of predictions would be asserted/matched against the expected outcomes. This would mean that data scientists would need to work with product managers / business analysts to understand multiple different sets of data which would produce different class of predictions and write tests for matching these predictions against expected outcomes.
What would “Unit testing” mean?
Once the different set of input data vectors and related predictions are defined, the next step might be to plan different tests for testing different units of data and related predictions against the expected outcomes. These unit tests could be automated using continuous integration tools (such as Jenkins) build jobs. Each time the tests are run, the predictions are matched against the expected outcomes. In case, the predictions made by a unit of data does not match with the expected outcome, the error flag would be raised leading to regression bug.
Data Coverage or Code Coverage?
In traditional software development, the quality of unit tests is measured using the code coverage (line, branch coverage) done using unit tests. In case of machine learning models development, the quality of unit tests could be measured using different types of input data vectors and related predictions which got covered. This would require lot of inputs from product managers / business analysts. And mismatch would result in regression bugs which would mean that for certain set of data, the expected outcomes have changed (no more same as the previously set outcomes).
References
- Testing the machine learning models
- Blackbox testing for machine learning models
- Testing features of machine learning models
- What is machine learning?
Summary
In this post, you were presented with thought process in relation to what would unit testing mean for machine learning models? This would mean that it would be good for ML engineers and data scientists to learn the aspect of testing in relation to machine learning models.
- Completion Model vs Chat Model: Python Examples - June 30, 2024
- LLM Hosting Strategy, Options & Cost: Examples - June 30, 2024
- Application Architecture for LLM Applications: Examples - June 25, 2024
Leave a Reply