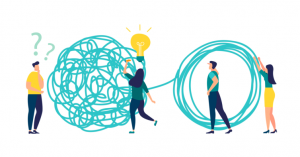
In this post, you will learn about some of the key challenges in relation to achieving successful AI / machine learning (ML) or Data science projects implementation in a consistent and sustained manner. As AI / ML project stakeholders including senior management stakeholders, data science architects, product managers, etc, you must get a good understanding of what would it take to successfully execute AI / ML projects and create value for the customers and the business. Whether you are building AI / ML products or enabling unique models for your clients in SaaS setup, you will come across most of these challenges.
Understanding the Business Problem
Many times, the nature of the problem becomes a challenge when you need multiple predictive models for different types of problems. If real-world problems, one regression model or one classification model may not be enough. What is needed in an ensemble of models that work together to provide the best solution. It requires someone having good analytical skills to break down the problems into smaller problems that can be tackled. It is recommended to have one or more product managers dedicated to data science teams to help them understand the problems in a better manner.
Whether a Machine Learning / AI solution is required & can be implemented?
The trickiest part of AI / ML projects is to identify whether a business problem can be solved using machine learning solutions. Many times, the solution to the problem can be based on implementing a complex set of rules. In order to determine whether a problem has a solution based on machine learning and can be solved using machine learning, one needs to ensure the following:
- Define the machine learning tasks
- Understand what kind of data is required and whether data is available
- Define performance metrics that can be used to evaluate models
Business Value Metrics Definition
Apart from model performance metrics, it is very / extremely important to identify the value metrics which can be used to evaluate the machine learning-based solution. Value metrics refer to the business metrics. Many a time, models get deployed in production but in absence of value metrics, the model utility fails to get ascertained, and hence this results in the failure of the project.
Lack of Representative Training Data
This is often the biggest challenge to data science / AI projects. In order to build models and algorithms, data is required. If data is not available or the available data is not representative of problem we are trying to solve, it can be difficult or impossible to complete the project. In order to generalize well with the predictions, it is very important to gather the training data which can be representative of the new cases that are desired to be generalized. This is true whether one selects to go for instance-based learning or model-based learning. If the size of the data is too small, it may not be enough to train the models properly. A problem may require data sets that are internal and readily available, and, the ones which are external and need to be collected/bought. If the training data sample is too small, this will result in sampling noise. The data can also turn out to be non-representative even if the data is too large depending upon the sampling method. This can result in sampling bias.
It is very important to determine the data sources (internal and external) which will be used to train the models. In a SaaS setup, this data comes from the customer database. Given the fact that customers can have data stored in different formats, it becomes a challenge to gather the right kind of data which can be used to train the models.
Given that a machine learning problem could cut across different product lines having different databases, DS team members working on a particular problem related to a particular product only get to access the data from the database related to that product. This limits the ability to come up with a great set of features that could span across different product databases. For example, the team working on product A problem does not get to study the data from product B or product C which could provide useful insights in analyzing the problem and solution approaches. Similarly, team members working on product C problems do not get to see the related data (business domain) in other databases such as product A or product B. Given the fact that business functions many times are interconnected, not having the data from different product databases would most likely result in sub-optimal models.
Data Quality
The following are some of the key aspects of data quality which poses challenges for building machine learning models having high performance:
Data accuracy
Building machine learning models requires reliable and accurate data. The model relies on labeled data to teach it the parameters of what constitutes a correct output — so if labels, or the data itself, is incorrect then the model will be trained incorrectly and be destined for failure when presented with new information. Data accuracy also provides more precise results from the model since differences between unseen examples can be accurately judged by the machine before making a predictive decision. To ensure one’s machine learning models are reliable and accurate, data should have correct data labels and variable types. This requires precise cleaning up of the dataset as well as deducing the underlying connections between inputs and outputs which will enable better accuracies in modeling applications related to forecasting or predicting customer behaviors. It is important to recognize that this effort in ensuring high-quality datasets often saves time in the long run versus training a flawed model that only results in erroneous conclusions down the line.
Data completeness
When we build machine learning models, the accuracy of our predictions depends heavily on the completeness and balance of the data. Machine learning algorithms are able to create incredibly accurate predictions based on data that is varied, complete, and correctly labeled. To ensure accuracy, developers must ensure they have a complete dataset with enough labels to cover all possible scenarios. For example, if we’re trying to detect objects in an image, then our dataset should be balanced evenly between all the objects that might appear in an image including cars, bikes and people. This will give us a more genuinely representational set which can better predict how these objects may look in any given scene. Similarly for sentiment analysis or other classification tasks, the training dataset must be washed properly and provided with varied labels for each situation before being used to develop a machine learning model. This will ensure that the algorithm can identify unique data points easily, leading to improved and less biased prediction accuracy than if it was trained on a muddled data set.
Data consistency
Building a machine learning model requires a significant amount of data consistency. Most models rely on data from multiple sources, such as multiple readings from sensors or customer-provided surveys. In order for the model to be effective, this data must be consistent and free from human bias. Any inconsistency in the initial sources can lead to errors in the final product and decreased accuracy of predictions. This is why it is important to maintain data consistency when building machine learning models. To achieve this, extra care must be taken to make sure that all data comes from reliable and consistent sources, with data validation procedures put in place to identify any potential discrepancies between inconsistent data sources. Without ensuring data consistency, human bias can easily distort results and give us false readings that could have severe consequences if used for decision making purposes within ML models. By taking the necessary steps towards addressing these issues, we can ensure that our machine learning models are robust and accurate enough to provide reliable predictions.
Data timeliness
When building machine learning models, it’s important for data to be up to date and accurate in order for the models to perform properly. This is referred to as timeliness, or the sensitivity of data to changes over time. Data latency challenges can arise when this requirement is not met; such challenges may include long wait times before changes are reflected in a model’s results, as well as stale data that prevents the best decision from being reached. To avoid these challenges, developers must work diligently on optimally configuring their machines and services to provide timely data updates with minimal latency. Having realistic expectations of what can be done within current technological constraints is an important step towards finding solutions that make the necessary adjustments quickly and accurately while avoiding errors.
Data complexity
Another common challenge is data complexity. This can include data that is unstructured, noisy or has a lot of missing values. Data complexity can make it difficult to build accurate models and algorithms. Data complexity can be further aided by inconsistency and inaccuracies in data. In addition, the non-stationarity of data can also make data complex and difficult to work with. It means that the data changes over time. This can make it difficult to build models that accurately predict future data points.
Inappropriate or Insufficient feature engineering
The model will have high performance if the training data contains enough relevant features and not too many irrelevant ones. A critical part of the success of a machine learning project is coming up with a good set of features to train on. The process of coming up with appropriate features is called as feature engineering.
There are several key aspects of feature engineering that can result in great features for building machine learning models. Some of the most important aspects include:
-
Domain knowledge: Having a deep understanding of the problem domain can be crucial for identifying useful features and designing effective feature engineering strategies. This knowledge can help to identify the most important characteristics of the data and guide the selection and creation of features that capture these characteristics.
-
Feature selection: Choosing the right features is crucial for building effective machine learning models. This involves selecting a subset of the available features that are most relevant to the problem at hand and discarding irrelevant or redundant features. Feature selection can help to improve the model’s performance by reducing overfitting and increasing the interpretability of the model.
-
Feature transformation: Feature transformation involves modifying the existing features in order to improve their usefulness for the machine learning model. This can involve techniques such as normalization, scaling, and encoding categorical data. Feature transformation can help to improve the model’s performance by making the features more amenable to the learning algorithm.
-
Feature extraction: Feature extraction involves creating new features from the existing data. This can be done using techniques such as principal component analysis, independent component analysis, and kernel-based methods. Feature extraction can help to improve the model’s performance by discovering hidden patterns and relationships in the data that were not apparent in the original features.
Reproducability
The ability for one to be able to reproduce the results of a machine learning model is essential for achieving trust and accuracy. However, despite the many advances made in machine learning, there is still considerable difficulty reproducing the output of such models. This is due to any number of challenges that arise when building and deploying machine learning applications. These range from data quality and formatting issues, to variations among different computing environments, as well as a lack of transparency as to how calculations were performed.
How do I ensure that my model is reproducible? One possible solution is to fix the random seed value and record all necessary parameters whenever performing any machine learning experiments. This would guarantee that you always have the ability to go back to ones initial setup and obtain the same outcomes over and over again. Additionally, checking across different platforms, such as CPU or GPU enabled machines, may help further increase your confidence in your model’s reproducibility. Other aspects include data splitting mechanisms resulting in training and test data, data preparation, learning rate, batch size, etc.
Data Labelling
In some cases, you may not have enough labeled data to train your machine learning models. This can be a challenge because labeling data is a time-consuming and expensive process. You may need to hire someone to label data or use a data labeling service. Problems related to document classification, image classification, object detection, etc may require data labeling.
Data Privacy related challenges
Given the constant need to manage and monitor data preparation/processing and data access from different teams working across different offices / geographic locations, from a security standpoint, the database (DB) and data science (DS) team along with the data security team will need to collaborate at regular intervals to make sure data scientists have access to right data set in a secured manner. In this relation, the DB team and DS team would need to collaborate at regular intervals for data gathering and preparation.
When working with data, it is important to consider data privacy. This includes ensuring that critical or sensitive data is not released without consent and that data is not used for illegal purposes. Data privacy can be a challenge when working with data sets that contain sensitive information. When working with data scientists having different levels of experience or those belonging to different organizations, data privacy concerns can increase. Data masking is one of the common data privacy techniques used in data science
Data scientists working from different geographic locations would need easy and quick access to data while meeting the data security requirements. It may so happen that in one of the locations, the data scientists are juniors or interns who may not be given full data access due to different security reasons. Rather, they will be provided with selected data sets consisting of selected columns from selected tables in order to meet data security requirements. This throws up the challenges such as a need for data security, database and data science team to work on data preparation, data masking, assessing data security, and making data available in the desired format such as CSV. And, this requires the regular intervention of this team due to the need to constantly assess data security requirements. From a business standpoint, this would not only impact data science team productivity but also delay the deployment of models in production leading to business impacts.
Computing intensive feature engineering/processing
Most of the time, data scientists use laptops for building models. And, the laptops are having computing resources constraints vis-a-vis data / big data processing requirements. Thus, the data scientists across different locations will be constrained to work on only a selected set of problems (building models) with only a limited set of features where data volume is not large enough. In case you have huge clients having big data, this is going to impact business sooner than later in terms of providing high-quality AI-powered solutions in a timely manner.
Scalable platform for serving predictions
Many times, the ML platform is unable to support prediction requirements within the desired time period given computing-intensive feature calculations which are required to be done during runtime. What is desired is the distributed calculations for feature processing for faster predictions. This is where the ML platform needs to scale in a manner that it could handle big data requirements including running Spark workload for feature/data processing and serving predictions.
Longer Lead Time for Machine Learning Model Deployments
There is always a need for processing data of large volume. The fact that the DS team generally uses their laptops for data processing and model building becomes a big constraint. This, in turn, leads to delays in relation to breaking data into chunks and then processing them on one’s laptop. Additionally, the need for training the model with a large volume of data becomes a constraint due to the lack of big data infrastructure. And, this results in delays related to building models and moving them into production.
AI/ML support & education (different teams)
Finally, AI / ML team can work in a silo and create value for the business. The predictions need to be consumed by the products and thus, engineering tea has an important role to play in the integration. The models need to be deployed in production by the IT team. The customer service team needs to interact with the client in relation to predictions. Consultants may need to understand how the model predictions work in order to customize and deploy the solution.
In order to achieve the above requirements in a seamless manner, it becomes very important to provide high-level education to different stakeholders including some of the following:
- Software engineering team including product managers
- IT team deploying AI / ML products
- Customer service team
- Consultants designing AI / ML solutions
- Sales & marketing team selling AI products/solutions
Machine Learning Model Performance Governance
Another challenge is the need to have models perform better in terms of accuracy and precision. This can be difficult to achieve if the data is not of high quality or if the data is not enough in size. It requires setting up model governance and data management processes. One of the key aspects is setting up tools & processes in place for regular model re-training if required. This can be due to changes in the data or the need to improve model performance. Retraining models can be time-consuming and expensive. It can also be difficult to retrain large models that have been trained on a lot of data.
Lack of Resources (Skill, Computing, Storage)
Another challenge is the lack of resources. Data science / AI projects can require a lot of resources in terms of data storage, computing power, and human capital. If these resources are not available, it can be difficult to complete the projects. One of the key reasons why data science / AI projects fail is due to the lack of expertise in terms of skilled data scientists and data engineers. Hiring expert data scientists can be a challenge because there are many different skills required for data science. In addition, data scientists are in high demand and may be expensive to hire. You may need to hire multiple data scientists with different skill sets.
Lack of leadership support
Finally, Data science / AI projects need leadership support in order to be successful. This includes executive buy-in, adequate funding, and the right team. Without leadership support, data science / AI projects can be difficult to implement.
These are some of the common challenges data scientists and AI practitioners face when working on data science / AI projects. While there are many challenges, data science / AI projects can be successful if these challenges are overcome. To increase the chances of success, it is important to have a clear understanding of the problem, use high-quality data, and select the right algorithms. In addition, it is important to have the resources required to complete the project. Finally, data scientists and AI practitioners need to be aware of the common challenges and how to overcome them. By doing so, they can increase the chances that their data science / AI projects will be successful.
- Completion Model vs Chat Model: Python Examples - June 30, 2024
- LLM Hosting Strategy, Options & Cost: Examples - June 30, 2024
- Application Architecture for LLM Applications: Examples - June 25, 2024
Leave a Reply