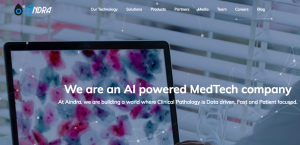
AIndra, a Bengaluru-based startup is into the business of building innovative products and technologies to aid computational pathology. Established in 2014, AIndra’s vision is to build state of the art medical devices for screening Cervical Carcinoma.
This article is created solely based on my analysis of information found on AIndra’s website. The objective is to make readers aware of some of the following technologies AIndra is using and, how they can be used to solve healthcare problems, in general. The goal is provide food for thought to the readers such that they can use some of these technologies in their future startups.
- Computation Pathology
- Telepathology
If you work in AIndra, please feel free to reach out if you think further information could be added.
AIndra’s Products
The following are key aspects of screening / analysis which are taken care by related AIndra products:
- Autostaining (Intellistain): Staining is one of the key aspect of data gathering phase. AIndra provides a product namely, Intellistain, which supports staining procedures such as PAP test etc and help achieve high quality staining.
- Data capture from slides (Visionex): Another key aspect of data gathering is digitizing the slides. In other words, capturing the slide image in clear manner using a device. This is where Visionex comes into picture. The primary goal of Visionex is to digitize the slides in most efficient manner.
- Predictive analysis: Once data (images) is captured through Visionex, this is used for building models. This is where AIndra AI algorithm, Astra comes into picture.
AIndra’s Technology
AIndra is making use of some of the following technologies to achieve the AI-powered cervical cancer screening:
- Telepathology to retrieve image-rich pathology data from different diagnostic centres. Technology such as computer vision, internet of things (IOT), cloud computing etc would play key role in accomplishing telepathology.
- Computational pathology to process the data retrieved from different diagnostic centres
- Artificial intelligence system (algorithm) can be used to analyse data and predict one or more outcomes from time-to-time. For example, one of the outcome can be likelihood of a person suffering from cervical cancer.
Telepathology
As per Wikipedia page on Telepathology, Telepathology is the practice of pathology at a distance. It uses telecommunications technology to facilitate the transfer of image-rich pathology data between distant locations for the purposes of diagnosis, education, and research.
The primary idea is to transfer the image-rich pathology data to distant location. This can be very effective if you are planning to gather slides details (images) from different diagnostic centres. From business perspective, AIndra might require to undergo partnership with multiple hospitals / diagnostic centres across different cities and gather the slides images from them using Telepathology techniques such as some of the following:
- Virtual slide system which can be used to create digital image file of the glass slide and store the file on a computer server (preferably locally). These files can, then, be sent to cloud or remote servers over internet. The disadvantage is the low internet bandwidth and large image sizes. Given that internet speed is improving day-by-day, this can be the most viable system. Visionex or related products might play important role into this.
- Static image system
- Real-time robotic microscopy
Technologies for Telepathology
The following is the list of some of the key technologies which could be used to implement telepathology:
- IOT device to capture the data and send the data over internet to remote location (cloud servers)
- Image scanning system (such as Visionex)
- High-speed internet
- Remote Image / File server (such as that present on Cloud)
- Application to send the file to remote server
Computational Pathology
Computational pathology, generally speaking, can said to be an approach to diagnosis that incorporates data from multiple sources such as some of the following to generate diagnostic inferences. These diagnostic inferences provide doctors / patients with actionable knowledge.
- Pathology
- Radiology
- Clinical, molecular and lab operations
The following are some of the benefits of using computational pathology as part of overall pathology ecosystem:
- Helps improve diagnostic accuracy: This is given the fact that with time, number of tests are ever increasing along with number of patients and a limited number of pathologists. This leads to higher chances of error. This is where computational pathology comes into picture.
- Patient care optimization: Given improvement of diagnostic accuracy, patient care is optimized to a great extent.
- Cost reduction: Given above, patients require to shell out lesser money to get effective treatment / care.
AIndra is using product such as Intellistain and Visionex for capturing data from data source such as slides.
Technologies for Computational Pathology
The following is the list of technologies which can be used (IMHO), in general, to implement computational pathology solutions:
- IOT Devices: IOT device can be used to retrieve data from remote / distant diagnostic centres. The data, however, can be manually sent from these distant diagnostic centres.
- Cloud-computing for scalable data storage and large-scale, on-demand data processing
- Big Data: This, surely, looks to be a big data problem given that the data may need to be gathered from different data sources at regular intervals (using scheduled jobs) in order to perform data analysis. Following are some of the key data processing steps which may be required to implement the solution:
- Data Gathering: Data gathering from different data sources related to pathology, radiology, clinical data etc is the most critical step; There can be multiple different approaches to this:
- API-based integration to pull the data using APIs exposed by different systems
- Scheduled jobs which push / pull data from different systems and put it on some FTP server; There can be another set of scheduled jobs to write this data in distributed file systems such as HDFS.
AIndra uses the image scan using Visionex to gather / capture data from slides. These images can then be put on or sent to a centralized document / image server for further processing.
- Data Processing: Once data is put on the document / image server, the next step could be to process the data; This is guided by the analytics (machine learning Engineer/ data scientists) needs to come up with derived data sets from the original data.
- Data Gathering: Data gathering from different data sources related to pathology, radiology, clinical data etc is the most critical step; There can be multiple different approaches to this:
- Predictive Analytics: Following are some of the key analytics steps which can be used for prediction:
- Data preparation: Products such as Intellistain and Visionex plays key role in creating data to be fed into the AI algorithm, Astra.
- Building Models (Train and test the models): If this is about building models using image as data, the neural networks such as convolutional neural network could be the way to go.
- Deploy models: Models can as well be deployed on one of the cloud-computing platform such as AWS, Google cloud.
- Three Approaches to Creating AI Agents: Code Examples - June 27, 2025
- What is Embodied AI? Explained with Examples - May 11, 2025
- Retrieval Augmented Generation (RAG) & LLM: Examples - February 15, 2025
I found it very helpful. However the differences are not too understandable for me