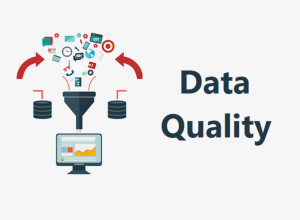
What is data quality? This is a question that many people ask, but it is not always easy to answer. Simply put, data quality refers to the accuracy and completeness of data. If data is not accurate, it can lead to all sorts of problems for businesses. That’s why data quality is so important – it ensures that your data is reliable and can be used for decision-making purposes. Data is at the heart of any enterprise. It is essential for making sound business decisions, understanding customers, and improving operations. However, not all data is created equal. In order to make the most out of your data, you need to good understanding of what is data quality and why is it important. In this blog post, we will discuss the importance of data quality and how you can ensure that your data is of the highest quality possible!
What is data quality?
In a digital world, data is everything. Businesses rely on data to make decisions, understand trends, and assess performance. And as data becomes more and more central to how businesses operate, the importance of data quality increases. Data quality refers to the accuracy, completeness, timeliness, and consistency of data. In other words, it’s about making sure that data is clean, accurate, and up-to-date. Why is this important? Because if data is unreliable, then any insights or decisions that are based on that data will also be unreliable. Poor data quality can lead to incorrect analyses and bad decision-making, which can have a negative impact on business performance. That’s why data quality is so important – it’s essential for making sound business decisions.
Data quality is one of the key components of data governance. Data governance is a framework for managing data throughout its life cycle. It includes processes and procedures for acquiring, storing, using, and destroying data.
The following are key attributes of data quality:
- Data accuracy: The data is correct and reflects reality. Data accuracy can be defined as the extent to which data meets user requirements. For example, if you are measuring the sales of a product, the data should accurately reflect how much of that product was sold. It is the responsibility of data owners to ensure data accuracy. The following are some of the challenges associated with data accuracy:
- Incorrect data: This is data that does not reflect reality. For example, if the spend data is incorrectly associated with a different spend category, it will lead to an inaccurate analysis.
- Duplicate data: This is data that appears more than once in the dataset. For example, if you have customer data with two different records for the same customer, that would be considered duplicate data.
- Missing data: This is data that should be present in the dataset but is not.
- Data completeness: All required data is included. Completeness can be defined as the degree to which all desired information is present in a set of data. Data completeness is often referred to as “the five Ws” – who, what, when, where, and why. All of this information should be included in order to get a comprehensive understanding of the data. For example, if you are measuring the number of products sold, you would want to include data on all products sold, not just a select few. The following are some of the challenges associated with data completeness:
- Incomplete data: This is data that is missing information. For example, if you only have partial customer data, that would be considered incomplete data.
- Irrelevant data: This is data that is not related to the question being asked. For example, if you are measuring the number of products sold, data on the number of employees in the company would be considered irrelevant data.
- Data timeliness: The data is up-to-date. Timeliness can be defined as the degree to which data reflects the current state of affairs. For example, if you are measuring the number of products sold, data from last year would not be considered timely data. The following are some of the challenges associated with timeliness:
- Outdated data: This is data that is no longer accurate because it reflects an earlier time period. For example, data from last month would be considered outdated data.
- Outdated data: This is data that is no longer accurate because it reflects an earlier time period. For example, data from last month would be considered outdated data.
- Data consistency: The data is reliable and consistent across different sources. Data consistency can be defined as the degree to which data is the same across different data sets. For example, if you have customer data from two different sources, and the data is inconsistent (e.g. one source has an email address while the other does not), then that would be considered inconsistent data. The following are some of the challenges associated with data consistency:
- Different data formats: This is data that is formatted in a different way than the desired format. For example, if you have customer data in a text file but you want it in a CSV file, that would be considered data in a different format.
- Duplicate data: This is data that appears more than once in the dataset. For example, if you have customer data with two different records for the same customer, that would be considered duplicate data.
- Structured vs. unstructured data: This is data that is organized in a specific way (e.g. in a table) or is not organized at all. For example, text data would be considered unstructured data, while data in a CSV file would be considered structured data.
- Data integrity: The data is accurate and has not been tampered with. Data integrity can be defined as the degree to which data has not been modified from its original state. For example, if you have customer data and someone has changed the data (e.g. changed the email address), that would be considered a data integrity issue.
Why is data quality important?
The following represents some of the reasons along with examples related to why data quality is important:
- Digital Transformation: As businesses undergo digital transformation, data quality becomes increasingly important. This is because data is at the heart of how businesses operate in the digital age. Many business processes are now automated and rely on data to function properly. For example, if you want to order a product online, the process will be automatically triggered when you enter your personal information and credit card details. The data you enter is used to make a decision on whether to approve the order or not. If the data is inaccurate, it could trigger an incorrect response – such as approving an order when the customer actually wants to cancel. This could lead to lost sales and damage to the company’s reputation.
- Decision-Making: Data is also used to make important decisions that can impact the business. For example, data is used to decide which products to sell, how much inventory to order, and where to allocate resources. If the data is inaccurate, it could lead to bad decisions that could hurt the business.
- Predictive modeling: In order to create predictive models having good accuracy, it is important to use high-quality data. If the data is inaccurate, it will lead to the creation of models which are not accurate. As a result, the business will not be able to rely on the predictions made by these models and could lose out on potential opportunities.
- Dashboard reporting: In order to create meaningful dashboards, it is important to use data that is of high quality. Data represents KPIs and KPIs are used to track the progress of the business. If the data is inaccurate, KPIs may not be reliable and this could result in the business taking the wrong actions. If the data is inaccurate, it will lead to the creation of dashboards that are not accurate. As a result, the business will not be able to rely on the information presented in these dashboards and could make wrong decisions.
- Business processes automation: As mentioned earlier, many business processes are now automated and rely on data to function properly. If the data is inaccurate, it could lead to these processes not working correctly or not working at all. This could cause disruptions in the business and could lead to losses.
What is the data quality improvement lifecycle?
The data quality improvement cycle is a process that can be used to improve the quality of data. The following represents some of the key steps of the data quality improvement lifecycle:
- Identification of data quality issues
- Analysis of data quality issues
- Correction of data quality issues
- Prevention of data quality issues
Who is responsible for data quality?
The following are some of the key stakeholders and teams which are responsible for data quality:
- Data owners: The data owner is responsible for the data and is responsible for ensuring that the data is of high quality. There can be enterprise data owners and data owners for specific data domains.
- Data architects: The data architect is responsible for the design of data systems and is responsible for ensuring that the data is of high quality. Data architects are responsible for data integration, data quality assessment, data profiling, and data cleansing.
- Data analysts: The data analyst is responsible for analyzing the data to identify issues and correcting
- Business users: Business users are responsible for using the data to make decisions. They are also responsible for providing feedback on data quality.
- Data stewards: The data steward is responsible for ensuring that the data meets the business’ requirements. They are also responsible for correcting data quality issues and preventing them from happening in the first place.
- Data analysts: The data analyst is responsible for data analysis and is responsible for ensuring that the data is accurate and of high quality.
What are data quality tools & frameworks?
There are different data quality tools & frameworks which primarily provide the following functionality:
- Data quality assessment: The tools or frameworks for data quality assessment help in assessing the quality of data. It allows you to identify data quality issues and it also provides a mechanism for correcting these issues.
- Data profiling: The tools or frameworks for data profiling help in profiling the data. It allows you to understand the data better and it also provides a mechanism for correcting data quality issues.
- Data cleansing: The tools or frameworks for data cleansing help in cleansing the data. It allows you to correct data quality issues and it also provides a mechanism for preventing data quality issues from happening in the first place.
Data quality is important for a variety of reasons, including decision-making, predictive modeling, dashboard reporting, and business processes automation. If the data is inaccurate, it could lead to bad decisions that could hurt the business. In order to create accurate predictions, it is important to use high-quality data. If the data is inaccurate, it will lead to the creation of dashboards that are not accurate. As a result, the business will not be able to rely on the information presented in these dashboards and could make wrong decisions. Lastly, if the data is inaccurate, it could lead to disruptions in the business and could lead to losses. By ensuring data quality is maintained, businesses can avoid these negative consequences.
- What are AI Agents? How do they work? - January 7, 2025
- Agentic AI Design Patterns Examples - January 6, 2025
- List of Agentic AI Resources, Papers, Courses - January 5, 2025
I found it very helpful. However the differences are not too understandable for me