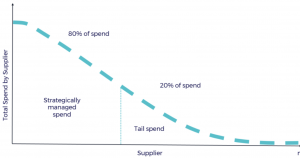
Do you know where your business is spending its money? And more importantly, do you know where your business SHOULD be spending its money? Many businesses don’t have a good handle on their tail spend – the money that’s spent on things that are not essential to the core operations of the company. Tail spend can be difficult to track and manage, but with the help of spend analytics tools and machine learning, it’s becoming easier than ever before. In this blog post, we’ll discuss what tail spend is, how to track it, and how to use analytics and machine learning to make better decisions about where to allocate your resources.
What is Tail spend and why is it important to track?
Tail spend is the percentage of total procurement spend that is incurred on low-value, ad-hoc purchases. It can as well be defined as the cumulative spend of an organization on all suppliers that fall outside of the core group of strategic suppliers (below a specific spend threshold). Different organizations have different spend thresholds below which any spend is termed as tail spend. For example, lets say the procurement threshold is $25K, than, any spend below $25K can be termed as tail spend. While tail spend only represents a small portion of total spend, it can have a significant impact on an organization’s bottom line. The picture below represents tail spend:
Tail spend often results in higher spend and longer lead times, as well as increased risk due to the lack of contracts in place. As a result, tracking tail spend and using tail spend analytics can help organizations to optimize their procurement processes and improve their bottom line. When it comes to managing tail spend, visibility is key. By tracking tail spend, organizations can gain insights into where and how they are incurring costs, as well as identify opportunities for optimization. Tail spend analytics can provide valuable insights into organizational spending patterns, helping to create a more efficient and effective procurement strategy.
Tail spend management challenges
Tail spend management can be a challenge for procurement professionals for a number of reasons.
- Tail spend can account for a significant percentage of total spend, but it is often spread across many different suppliers across different business divisions / units. This can make it difficult to get a clear picture of where tail spend is going and to negotiate better terms with suppliers.
- Tail spend is often made up of low-value items or one-time purchases, which are difficult to track and can make it challenging to justify the time and resources needed to manage it effectively. This can make it difficult to negotiate discounts with suppliers or to take advantage of volume discounts.
- Tail spend is often out of alignment with the rest of the organization’s spend, making it difficult to integrate into existing procurement systems and processes.
- Many organizations lack clear policies and procedures for tail spend management, leading to confusion and inefficiencies.
- Many tail spend management solutions are designed for larger enterprises, making them less accessible or affordable for small and medium-sized businesses.
Machine learning / AI & Tail spend management
The following are some tail spend management use cases which can be solved using machine learning / AI:
- Supplier bundling or clustering: One way to reduce tail spend is through supplier bundling or clustering. This approach uses machine learning to identify groups of similar suppliers. Machine learning algorithms such as k-means clustering can be used to group suppliers based on their spend patterns. This information can then be used to negotiate better terms with suppliers, or to consolidate spend with a smaller number of strategic suppliers. This can also help organizations improve communication, and streamline procurement processes. Additionally, machine learning can be used to create more accurate clusters, leading to even greater efficiencies. By consolidating spending with these suppliers, companies can realize significant savings.
- Tail items bundling or clustering: Another use case is products bundling or clustering. In this case, machine learning is used to group together tail items which are often purchased together. The idea is to consolidate and classify the tail items as different bundles. This approach can be used to create tail items bundles, which can then be offered at a discount to customers. This can also help organizations streamline their procurement processes, by reducing the number of individual purchase orders that need to be processed. In addition, the similar products are purchased across different business units / divisions. Machine learning / AI can be used to identify these similar products purchased across different BUs and accordingly, the products can be bundled and ordered appropriately.
- Tail spend classification: Tail spend can be classified into different categories such as spend fragmentation, price variance, off contract, spot buying, etc. Machine learning models can be trained to classify tail spend into above mentioned categories.
- Price variance: One of the major challenges with tail spend is that it can be very difficult to control. This is because it often consists of smaller, less essential items that are not purchased through the usual channels. As a result, there can be a lot of price variance from one purchase to the next. This variability can make it difficult to keep costs under control.
- Spend fragmentation: Spend fragmentation represents tail spend of similar tail items across different business units or departments with each business unit purchasing from a different supplier. As a result, tail spend can represent a significant opportunity for cost savings. One way to reduce tail spend is to consolidate purchasing through a centralized system. This can help to eliminate duplicate spending, reduce prices through volume discounts, and improve supplier performance through increased competition.
Tail spend management is a challenge for procurement professionals for a number of reasons. Tail spend can account for a significant percentage of total spend, but it is often spread across many different suppliers across different business divisions / units. This can make it difficult to get a clear picture of where tail spend is going and to negotiate better terms with suppliers. Tail spend is often made up of low-value items or one-time purchases, which are difficult to track and can make it challenging to justify the time and resources needed to manage it effectively. This can make it difficult to negotiate discounts with suppliers or to take advantage of volume discounts. In this blog, we looked at some of the key challenges associated with tail spend management and how machine learning can be used to overcome these challenges. We also looked at some specific use cases where machine learning can be used to improve tail spend management.
What are your thoughts on using analytics / machine learning for tail spend management? Have you tried it in your organization? Let me know in the comments below.
- Retrieval Augmented Generation (RAG) & LLM: Examples - February 15, 2025
- How to Setup MEAN App with LangChain.js - February 9, 2025
- Build AI Chatbots for SAAS Using LLMs, RAG, Multi-Agent Frameworks - February 8, 2025
Very informative and well-articulated post. Thank you