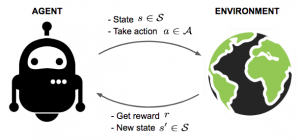
In this blog post, we’ll learn about some real-world / real-life examples of Reinforcement learning, one of the different approaches to machine learning where other approaches are supervised and unsupervised learning. Reinforcement learning is a type of machine learning that enables a computer system to learn how to make choices by being rewarded for its successes. This can be an extremely powerful tool for optimization and decision-making. It’s one of the most popular machine learning methods used today.
Before looking into the real-world examples of Reinforcement learning, let’s quickly understand what is reinforcement learning.
Introduction to Reinforcement Learning (RL)
Reinforcement learning is an approach to machine learning in which the agents are trained to make a sequence of decisions. It is defined as the learning process in which an agent learns action sequences that maximize some notion of reward. The agent, also called an AI agent gets trained in the following manner:
- The agent interacts with the environment and make decisions or choices. For training purpose, the agent is provided with the contextual information about the environment and choices.
- The agent is provided with the feedback or rewards based on how well the action taken by the agent or the decision made by the agent resulted in achieving the desired goal.
The diagram below represents the above. In the diagram below, the agent (software agent) takes an action in the given environment having state s. The environment sends a response to the agent in form of reward (r) and the new state information. The state changes as a result of action (a) taken by the agent.
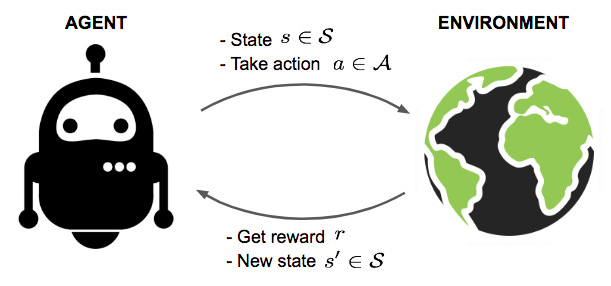
Here is another picture to illustrate the concept of reinforcement learning. The agent is initially in state St. It takes an action denoted by At in the environment. With action At taken with environment, the agent state changes to St+1. The environment sends reward signal if appropriate, denoted by Rt+1
The reinforcement learning models provide significant contributions in reinforcement-learning-based applications like robotics, web user interfaces, etc. Thus reinforcement learning is important to understand how such applications can carry out tasks in real life. Reinforcement learning also has various applications in video games and medical diagnosis systems.
What are different types of reinforcement learning algorithms?
There are 3 different types of reinforcement learning algorithms:
- Q-learning: The most important reinforcement learning algorithm is Q-learning and it computes the reinforcement for states and actions. The output of Q-learning depends on two factors, states, and actions. Q-learning is used in reinforcement learning problems where there are finite numbers of states and actions.
- Policy iteration: Policy iteration computes the reinforcement for states and actions by following two steps i.e., policy evaluation step followed by policy improvement step. In this reinforcement learning algorithm, there is an agent and a domain of states and actions. The task for the reinforcement learner is to find a policy that causes the reinforcement to increase for each initial state, without causing reinforcement from any other successor state to decrease. Policy iteration is used in reinforcement learning problems where there is an infinite number of states and actions.
- Value iteration: Value iteration computes reinforcement for states and actions by using reinforcement signal determined by reinforcement function. It is used when we know the environment transition equation and we need to find the action-value function which is a Q-function that gives us reinforcement for each state and action. Value iteration is used when the reinforcement learning algorithm is given complete information about the environment transition equation.
How & when to have RL models deployed in the production?
Here is one of the ways in which RL models can be deployed in production.
The user actions are recorded and stored in the database. The AI agent learns from this recorded data in batch mode. When the AI agent has learned enough from users’ actions to approximate the recommendation at high accuracy, the agent can be deployed in the production to let it learn by interacting with the end-users thereby supporting a positive user experience.
Real-life examples of Reinforcement Learning
Real-world examples where reinforcement learning can be used are usually found in the sciences, engineering, economics and finance. Here are some real-life examples of reinforcement learning. Reinforcement learning can be used in different fields such as healthcare, finance, recommendation systems, etc.
- Playing games like Go: Google has reinforcement learning agents that learn to solve problems by playing simple games like Go, which is a game of strategy. Playing this game requires reasoning and intelligence. Google’s reinforcement learning agent had no prior knowledge of the rules of the game or how to play it. It simply tried different moves randomly at first; then it “learned” which moves were the most likely to get the best results. It continuously learned until it was able to beat human players consistently. Using reinforcement learning, researchers at MIT created an algorithm called Deep Q-Network (DQN) that mimics the behavior of animals playing Atari games. As it moves through an environment, the reinforcement learning agent collects data. It uses this reinforcement learning data to evaluate possible actions and their consequences in order to determine which action will likely maximize its expected return of rewards .
- Self-driving cars: Reinforcement learning is used in self-driving cars for various purposes such as the following. Amazon cloud service such as DeepRacer can be used to test RL on physical tracks.
- Trajectory optimization: Reinforcement learning can be used to train an agent for optimizing trajectories. In reinforcement learning, the software agents could get reward from their environment after every time step by executing an action in the state. Reward is typically normalized to [0, 1].
- Motion planning including lane changing, parking etc
- Dynamic pathing: Reinforcement learning can be used for dynamically planning the most efficient path in a grid of potential paths.
- Controller optimisation
- Scenario-based learning policies for highways
- Data centre automated cooling using Deep RL: Use deep RL to automate the data center cooling. At regular time intervals, the snapshot of the data centre cooling system, being fetched from thousands of sensors, is fed into the deep neural networks. The deep NN predicts how different combinations of potential actions will impact the future energy consumption. The AI system, then, identifies the actions that will minimise the energy consumption. The most appropriate action is sent to the data centre. The recommended action is verified and implemented.
- Personalised product recommendation system: Personalise / customize what products need to be shown to individual users to realise maximum sale; This would be something ecommerce portals would love to implement to realise maximum click-through rates on any given product and related sales, on any given day
- Ad recommendation system: Customise / personalise what Ads need to be shown to the end user to have higher click-through rate. Reinforcement learning is used in large-scale ad recommendation system due to its dynamic adaptation of the Ad according to reinforcement signals and its success in real-life applications. For example, retargeting user who has already seen the product before, and show the product to user who has not yet seen it. User clicks on an ads and get directed to a landing page. The reinforcement signal is defined as the total click-through rate (CTR) of the ad. The reinforcement learning model calculates weights at each time step, and then updates them in real-time according to reinforcement signals. Thus, it learns how to best respond to reinforcement signals at each time step.
- Personalised video recommendations based on different factors related to every individual.
- Customised action in video games based on reinforcement learning; AI agents use reinforcement learning to coordinate actions and react appropriately to new situations through a series of rewards.
- Personalised chatbot response using reinforcement learning based on the behavior of the end user in order to achieve desired business outcome and great user experience
- AI-powered stock buying/selling: While supervised learning algorithms can be used to predict the stock prices, it s the reinforcement learning which can be used to decide whether to buy, sell or hold the stock at given predicted price.
- RL can be used for NLP use cases such as text summarization, question & answers, machine translation.
- RL in healthcare can be used to recommend different treatment options. While supervised learning models can be used to predict whether a person is suffering from a disease or not, RL can be used to predict treatment options given a person is suffering from a particular disease.
There are several cloud-based AI / ML services such as Azure Personalizer that can be used to train reinforcement learning models to deliver personalized solutions such as some of those mentioned above.
- Completion Model vs Chat Model: Python Examples - June 30, 2024
- LLM Hosting Strategy, Options & Cost: Examples - June 30, 2024
- Application Architecture for LLM Applications: Examples - June 25, 2024
Leave a Reply