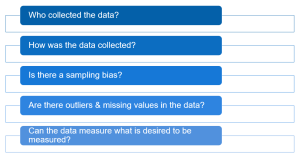
Data analysis is a crucial part of any business or organization. It helps make decisions and assists in strategy development. But before you can dive into the data, there are several questions that need to be answered first. These questions will help you understand whether you have right kind of data for analysis purpose in addition to defining your goals for data analysis. As data scientists or data analysts, it is your job to ask the right questions. Let’s take a look at some important questions to ask before starting data analysis.
Who collected the data?
When it comes to data analysis, it is essential to know who collected the data and how they did it. Questions that should be asked include: Was the data collected by an individual or a team? If so, who were they? Were they trained in data collection, and if so, what kind of training did they receive? How long did the data collection process take? Did the individuals collecting the data have any bias or preconceived notions about the subject material that could skew the results of the data collection process? It’s also important to consider whether there were any ethical considerations taken into account when collecting the data – for example, were confidentiality and anonymity respected to protect all involved parties? Questions like these are key when considering who collected the data and what effect this may have on its validity.
How was the data collected?
Before beginning any data analysis, it is essential to determine how the data was collected. Questions such as “What were the methods used for collection?”, and “When and where was the data gathered?” should be answered. Responses to these questions can provide insight into what types of bias or inaccuracies may exist in the data set due to sampling design or other factors.
Data collection can be done from a variety of sources including both internal and external data sources. Questions such as “Are the data from secondary sources or are they primary?”, and “What type of sampling techniques were used (e.g., random selection)?” can help to clarify whether the data set is reliable enough to be used for analysis. It is also important to consider factors such as the context in which the data was collected and any technical issues that may have impacted its accuracy.
Understanding how a data set was collected provides insight into what biases may exist in the results of any analysis conducted on that data. Asking these questions before beginning an analysis project can save time and resources by avoiding potential pitfalls or errors that could occur later.
Is there a sampling bias?
When it comes to data analysis, it is important to ask whether there is a sampling bias in the data. A sampling bias occurs when the data collected does not accurately represent the population being studied. This can occur if the sample size used is too small or if certain elements of the population are excluded due to their characteristics. For example, if a survey on consumer habits was conducted and people over a certain age were excluded because they did not fit the demographic of participants needed, then this would be considered a sampling bias.
Various types of sampling biases can occur in data collection, including selection bias, self-selection bias, and non-response bias. Selection bias occurs when certain elements of the population are more likely to be included in a sample than others. Self-selection bias occurs when respondents actively choose which questions to answer in a survey or questionnaire and do not answer all questions. Non-response bias occurs when respondents refuse to answer some questions or do not complete the survey altogether.
It is essential to account for potential sampling biases before beginning any analysis and ensure that steps are taken to prevent them from occurring during data collection. If these steps are not taken, the data analysis outcome might not be correct.
Are there outliers & missing values in the data?
Questions about outliers and missing values in particular can be especially helpful, as they can help pinpoint potential issues before beginning a deeper dive into the analysis.
Outliers are observations that are much higher or lower than the average value for a group. They can have a significant effect on results and should always be taken into consideration when performing an analysis. It is important to identify, analyze, and interpret outliers to determine if they represent meaningful information or if they simply represent errors in input data. Knowing which outliers are valid does not necessarily mean that they will be included in the final analysis; however, it provides greater insight into the dataset and its characteristics.
Missing values indicate areas within a dataset where data points have been left blank or incomplete. If these values cannot be identified or filled in through other means, then they should be taken into account when conducting an analysis. Ignoring missing values can lead to inaccurate results, as it will affect the data’s overall shape and distribution, skewing any conclusions that may be drawn from it.
Asking questions about outliers and missing values is an essential step of any data analysis process – it helps ensure the accuracy of your results by accounting for any potential issues with your input data before performing an investigation into its characteristics. Understanding these potential issues allows analysts to develop strategies for how to properly handle them during their workflows, leading to more effective outcomes over time.
Can the data measure what is desired to be measured?
Asking questions about whether the data can accurately measure what is desired to be measured is essential for successful data analysis.
Before beginning any data analysis project, it’s important to ask some key questions. Questions such as: What type of data do I need? Is this the correct type of data to answer my questions? Does this data contain all of the necessary information to make reliable conclusions? Is this dataset complete or incomplete? And if incomplete, how will that affect my results?
Asking such questions will help you determine whether or not your dataset is suitable for your needs and goals before you start analyzing it. Validating your dataset ensures that any conclusions drawn from it are reliable, trustworthy, and valid; otherwise, any decisions based on these results may be misinformed and inaccurate. Taking a few moments upfront can save time in the long run by providing insights into potential problems within the datasets and providing valuable direction on where further research should go.
Conclusion
Data analysis can provide valuable insight into any business or organization, but it needs to be done correctly in order for it to yield useful results. Asking yourself these important questions before beginning an analysis will help ensure success: What is my goal? Which data should I use? What tools do I need? Answering these questions will help focus your efforts on achieving meaningful results from your analyses, making them more useful and impactful overall!
- What are AI Agents? How do they work? - January 7, 2025
- Agentic AI Design Patterns Examples - January 6, 2025
- List of Agentic AI Resources, Papers, Courses - January 5, 2025
I found it very helpful. However the differences are not too understandable for me