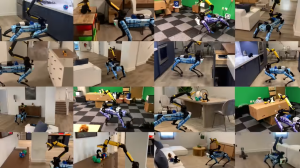
Meta AI has announced two major advancements in general-purpose embodied AI agents, focusing on challenging sensorimotor skills. These advancements include an artificial visual cortex called VC-1 and a new approach called Adaptive Skill Coordination (ASC). Both developments offer valuable benefits to data scientists and researchers in the field of AI. Embodied AI is field of AI focused on agents that can perceive, understand, and interact with their environment through sensorimotor experiences. It aims to create AI systems that can perform tasks in the physical world, bridging the gap between abstract thought and reasoning, and physical actions.
VC-1 is a single perception model that supports a diverse range of sensorimotor skills, environments, and embodiments. It is trained on videos of people performing everyday tasks from the groundbreaking Ego4D dataset, created by Meta AI and academic partners. VC-1 matches or outperforms the best-known results on 17 different sensorimotor tasks in virtual environments. Recall that visual cortex is a region of the brain that, in conjunction with the motor cortex, allows organisms to convert visual input into movement. Meta AI aims to create an artificial visual cortex for AI systems to enable artificial agents to translate camera input into actions. Sensorimotor tasks are the tasks that involve the integration of sensory perception and motor control to perform actions in the environment.
ASC, on the other hand, achieves near-perfect performance (98 percent success) on the challenging task of robotic mobile manipulation. It involves navigating to an object, picking it up, navigating to another location, placing the object, and repeating the process in physical environments. ASC comprises three components: a library of basic sensorimotor skills, a skill coordination policy to select appropriate skills, and a corrective policy that adapts pretrained skills when out-of-distribution states are perceived.
These breakthroughs are powered by data, as embodied AI requires data that captures interactions with the environment. The researchers have developed new ways for robots to learn using videos of human interactions with the real world and simulated interactions within photorealistic simulated worlds.
The artificial visual cortex, VC-1, is expected to benefit data scientists by providing a comprehensive pretrained model that can be used across diverse sensorimotor tasks. It has been trained on more than 4,000 hours of egocentric human video from seven diverse datasets and required over 10,000 GPU-hours of training and evaluation.
ASC, developed in collaboration with Georgia Tech, will help researchers build robots capable of performing long-horizon tasks and adapt to new and changing environments, overcoming hardware instabilities, picking failures, and adversarial disturbances.
These advancements open new avenues for sim2real research and the development of scalable, robust, and diverse robot assistants of the future. They can operate in new environments out of the box and do not require expensive real-world data collection.
- What is Embodied AI? Explained with Examples - May 11, 2025
- Retrieval Augmented Generation (RAG) & LLM: Examples - February 15, 2025
- How to Setup MEAN App with LangChain.js - February 9, 2025
I found it very helpful. However the differences are not too understandable for me