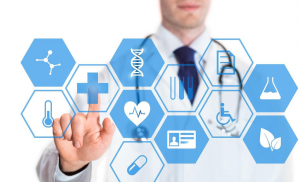
AI & Machine learning is being used more and more in the healthcare industry. This is because it has the potential to improve patient outcomes, make healthcare more cost-effective, and help with other important tasks. In this blog post, we will discuss some of the healthcare & AI / machine learning use cases that are currently being implemented. We will also talk about the benefits of using machine learning in healthcare settings. Stay tuned for an exciting look at the future of healthcare!
What are top healthcare challenges & related AI / machine learning use cases?
Before getting into understand how machine learning / AI can be of help in healthcare, let us first understand what are the top healthcare challenges:
- Lack of access to quality care: According to the World Health Organization, nearly half of the world’s population does not have access to essential health services. This problem is especially acute for low-income and minority populations. Access to essential health services is one of the most important factors in achieving quality care. However, challenges such as poverty, poor infrastructure, and inadequate staffing can prevent people from getting the care they need. In addition, even when services are available, they may not be of high quality. This can be due to a lack of trained personnel, outdated equipment, or a lack of supplies. When essential health services are not accessible or of poor quality, it can have a significant negative impact on people’s health. Lack of access to quality healthcare can lead to a number of serious health problems, including increased risk of chronic disease, higher mortality rates, and poorer overall health. Lack of access to quality healthcare is a serious problem that needs to be addressed. This is a huge problem that needs to be addressed. There can be different solutions approaches and AI / machine learning can be used in some of these solutions. For example, one of the solution approach is conducting regular or monthly free or subsidized health checkups camps. The following are some of the challenges which can be addressed using AI / machine learning: A. Which are the areas where govt or private institutions can contribute in conducting medical camps for free or subsidized checkups? B. How to design optimal health package keeping in mind disease prevalent in particular area or community? C. Which healthcare professionals or organization to be deputed or partnered with, to conduct this health-checkups? D. What incentives to be given to these HCPs or organizations who conduct these checkups?
- Rising healthcare costs: Rising healthcare costs are a major concern for families, businesses, and the government. Healthcare costs have been rising for years, and there is no end in sight. The rising cost of healthcare is a major driver of inflation, and it is one of the biggest factors driving up the cost of living. Rising healthcare costs are also a major burden on businesses, who must provide health insurance for their employees. The rising cost of healthcare is also a major concern for the government, which must provide healthcare for its citizens. In many countries such as India, although public hospitals provide free health care, they are not as accessible or well-equipped as private institutions and patients must pay for a majority of medical expenses. There can be different solutions to address these challenges. And, AI/machine learning can be leveraged for some of these solutions. The following are few examples where AI / machine learning can be helpful: A. Identifying areas where there is a mismatch between healthcare costs and standard of living B. Identifying need for affordable healthcare services including medicines area-wise C. Finding out which healthcare services and medicines costs can be customized with the help of subsidies, etc.
- Aging population: The aging population is one of the biggest healthcare challenges facing society today. As people live longer, they are more likely to develop age-related chronic diseases such as diabetes, heart disease, and Alzheimer’s. These conditions can be costly to treat and often require long-term care. In addition, the aging population is also more likely to need help with activities of daily living such as cooking, cleaning, and bathing. As a result, the demand for healthcare services is increasing at a rapid pace. Healthcare organizations must find ways to provide high-quality care for an aging population while also controlling costs. This is a daunting task, but it is one that must be addressed if we are to meet the challenges of an aging society. There can be different solutions approaches and AI / machine learning can be used in some of these solutions. The following are some of the challenges which can be addressed using AI / machine learning: A. Classifying the aging population which may need regular preventive health checkups B. Classifying the aging population which may be needing the free or subsidized checkups C. Recommending the health-checkups which may be needed by specific group of aging population
- Need for greater emphasis on preventive care: There is a need for greater emphasis on preventive care in the healthcare system. Preventive healthcare is an important aspect of overall healthcare. It refers to the measures taken to prevent disease or illness, rather than treating it after it has already developed. Preventive healthcare can take many forms, including vaccinations, regular check-ups, and lifestyle changes. By taking steps to prevent disease before it occurs, we can save lives and reduce the burden on our healthcare system. In addition, preventive healthcare is often less expensive than treatment, making it a wise investment for both individuals and society as a whole. Despite its many benefits, preventive healthcare is often underutilized. One reason for this is that people often do not see the need for preventive measures until they are faced with a health problem. Another reason is that preventive care can sometimes be inconvenient or uncomfortable. However, the rewards of preventive healthcare are well worth the effort. By taking steps to protect our health today, we can enjoy a healthier tomorrow.
- Need for trained and efficient manpower: There is a greater need for trained and efficient manpower in healthcare. The healthcare industry is facing a shortage of qualified personnel including doctors, nurses, paramedics, and basic healthcare workers. This shortage is expected to grow as the population ages and the demand for healthcare services increases. In order to meet the needs of an aging population, healthcare organizations must find ways to attract and retain qualified personnel.
- Lack of high quality data: One of the biggest healthcare challenges is the lack of high quality data. When going for digital transformation in healthcare for providing speedy care to patients, good quality data plays important role in building great AI / machine learning models. The data collected by healthcare organizations is often of poor quality. This is due to a number of factors, including the fact that healthcare data is often collected manually, which can lead to errors. In addition, healthcare data is often siloed, which makes it difficult to share and use. This makes it difficult for researchers to develop new treatments and for healthcare organizations to make evidence-based decisions. In addition, the healthcare industry has been slow to adopt new technologies, which makes it even more difficult to collect and analyze data. This is a major problem that needs to be addressed if we are going to use machine learning / AI effectively in healthcare settings.
- Data labelling for building AI / Machine learning systems: In healthcare, data is often unstructured and incomplete. This makes it difficult to train machine learning models. In addition, healthcare data is often sensitive and private. As a result, healthcare organizations must take care to protect patient privacy while also ensuring that data is properly labelled. Data labelling is a complex and time-consuming task, but it is essential for training machine learning models.
- Need for greater digital interoperability: Given that there ae large number of devices which are creating data in different formats, the challenge exists to make this data easily accessible to healthcare professionals. Interoperability challenges arise from a lack of standards, which makes it difficult to exchange data between different systems. In addition, healthcare organizations often have legacy systems that are not compatible with newer systems. As a result, healthcare organizations must find ways to improve digital interoperability in order to make the most of their data. This is a complex task, but it is one that must be addressed if we are going to use machine learning / AI effectively in healthcare settings. In order for machine learning to be used effectively in healthcare settings, there needs to be greater digital interoperability. This means that data must be able to flow freely between different healthcare organizations and different devices. Unfortunately, the healthcare industry has been slow to adopt standards for digital interoperability. This is a major problem that needs to be addressed if we are going to use machine learning effectively in healthcare settings.
- Explainability of AI solutions: In healthcare, it is often important to explain why a particular decision was made. For example, if a machine learning system recommends a course of treatment for a patient, it is important to be able to explain how the system arrived at that recommendation. This is known as explainability. Explainability is important for many reasons, including the fact that it can help to build trust in machine learning systems. In addition, explainability can help healthcare professionals to understand and use machine learning systems. However, explainability is often difficult to achieve, due to the complex nature of machine learning models. As a result, healthcare organizations must take care to ensure that their machine learning models are explainable.
Key Hypotheses for Data Scientists in relation to Healthcare Challenges
The following are some of key hypotheses which can result in improved healthcare:
- Greater number of educated healthcare personnel can result in improved healthcare. This would mean identifying courses which healthcare personnel can take to improve their skills. In addition, government can work with medical institutions to provide these courses. In addition, the smart and intelligent systems leveraging AI / machine learning can be used to predict demand for healthcare services. This would help to better allocate resources and staff.
- If we can develop better methods for data collection and analysis, this will result in creation of great quality & reliable applications & solutions thereby resulting in improved healthcare.
- If we can find ways to improve digital interoperability, this can help companies working in healthcare ecosystem including hospitals, diagnostic labs, etc to adopt and adapt to digital transformation thereby enabling them make fast and informed decisions and serve patients in a better manner.
- If we can improve explainability of machine learning models, this will help healthcare professionals to trust, understand and use machine learning systems appropriately in a reliable manner thereby leading to improved healthcare.
As time progresses, I will be updating these blogs to include more AI and machine learning use cases & projects for healthcare.
- Questions to Ask When Thinking Like a Product Leader - July 3, 2025
- Three Approaches to Creating AI Agents: Code Examples - June 27, 2025
- What is Embodied AI? Explained with Examples - May 11, 2025
I found it very helpful. However the differences are not too understandable for me