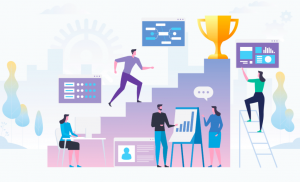
In this post, you will learn about some of the common success metrics that can be used for measuring the success of AI / ML (machine learning) / DS (data science) initiatives / projects / products. If you are one of the AI / ML stakeholders including product managers, you would want to get hold of these metrics in order to apply right metrics in right business use cases. Business leaders do want to know and maximise the return on investments (ROI) from AI / ML investments.
Here is the list of success metrics for AI / DS / ML initiatives:
- Business value metrics / key performance indicators (KPIs): Business value metrics related to top-line or bottom-line impacts can be used to measure AI / ML product / solution contribution to overall business success. Thesevalue metrics can also be termed as the lagging KPIs. Gartner recommends that value metrics / lagging KPIs should be defined before the AI project is deployed (as a baseline) and again few months of project or solutions deployment to determine the resulting benefit compared to the baseline. KPIs are defined as metrics that can be used to test and verify hypothesis by measuring performance against the baseline performance. AI solutions are mostly offered as part of product offerings. Thus, one can use product operational KPIs to measure the effectiveness of AI / ML solutions. Some of the product operational KPIs include cost per acquisition, number of new sessions, users’ retention rate, users’ conversion rate, number of sign-ups etc. Here is the hypothesis: The more pronounced the AI capabilities in the product / solutions are, the greater their influence on operational KPIs. In case the product is largely an AI-based product, then the value metrics of product can be directly associated with effectiveness of AI/ML solutions embedded in the product.
- AI / ML Technical metrics: Many a time, product operational KPIs or value metrics is not enough to measure the effectiveness of AI solutions. For example, enhanced usage of email can’t be enough to measure the effectiveness of spam filter until proper isolation is done in some manner. Thus, it is recommended to use AI / ML technical metrics in conjunction with business value metrics / KPIs to measure the effectiveness of AI / ML solutions. Here are different kinds of technical metrics which could be used with different kind of AI/ML solutions:
- Classification accuracy
- Root mean square error (RMSE)
- Mean absolute error (MAE)
- Sensitivity / specificity average
- Number of business questions / problems / requests addressed; Responsiveness to the business questions / problems / requests
- Number of departments / business users supported / adopted the solution
- Number of models deployed in production
- Availability of the models
- Infrastructure cost usage in relation to the models (during build and in production)
- Metrics measuring carbon footprint of models given deep learning models long training time
- Volume of data analyzed
- AI / ML organization strength
- Number of white papers published
References
- Mathematics Topics for Machine Learning Beginners - July 6, 2025
- Questions to Ask When Thinking Like a Product Leader - July 3, 2025
- Three Approaches to Creating AI Agents: Code Examples - June 27, 2025
I found it very helpful. However the differences are not too understandable for me