Category Archives: Large Language Models
Agentic AI Design Patterns Examples
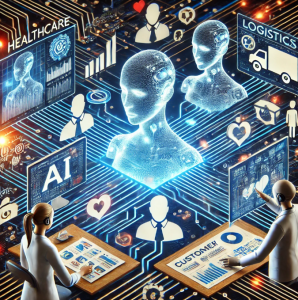
In the ever-evolving landscape of agentic AI workflows and applications, understanding and leveraging design patterns is crucial for building effective and innovative solutions. Agentic AI design patterns provide structured approaches to solving complex problems. They enhance the capabilities of AI agents by enabling reasoning, planning, collaboration, and tool integration. For instance, you can think of these patterns as a blueprint for constructing a well-oiled team of specialists in a workplace—each with unique roles and tools, working in harmony to tackle a project efficiently and innovatively. Imagine a team of engineers collaborating on designing a new car, where one member focuses on aerodynamics, another on engine performance, and a third on …
List of Agentic AI Resources, Papers, Courses
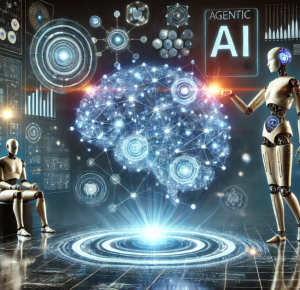
In this blog, I aim to provide a comprehensive list of valuable resources for learning Agentic AI, which refers to developing intelligent systems capable of perception, autonomous decision-making, reasoning, and interaction in dynamic environments. These resources include tutorials, research papers, online courses, and practical tools to help you deepen your understanding of this emerging field. This blog will continue to be updated with relevant and popular papers periodically, based on emerging trends and the significance of newly published works in the field. Additionally, feel free to suggest any papers that you would like to see included in this list. This curated list highlights some of the most impactful and insightful …
OpenAI GPT Models in 2024: What’s in it for Data Scientists
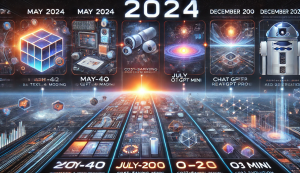
For data scientists and machine learning researchers, 2024 has been a landmark year in AI innovation. OpenAI’s latest advancements promise enhanced reasoning capabilities and multimodal processing, setting new industry benchmarks. Let’s dive into these milestones and their practical implications for data scientists. May 2024: Launch of GPT-4o OpenAI introduced GPT-4o (“o” for “omni”), a multimodal powerhouse designed for text, image, and audio processing. With faster response times and improved performance across multilingual and vision tasks, GPT-4o offers a great tool for developing advanced AI applications. Early adopters have reported up to 40% efficiency gains in tasks requiring multimodal analysis (Smith et al., 2024). GPT-4o’s ability to process and integrate multi-modal …
Collaborative Writing Use Cases with ChatGPT Canvas
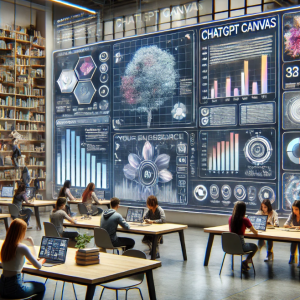
ChatGPT Canvas is a cutting-edge, user-friendly platform that simplifies content creation and elevates collaboration. Whether drafting detailed research papers, crafting visually engaging presentations, or writing professional emails, ChatGPT Canvas has the tools to make your work efficient and impactful. This guide explores leveraging Canvas effectively, tailored for college-level users and professionals alike. Developing Blogs, Articles or Research Essays Research essays, blogs & articless demand clarity, depth, and methodical organization. ChatGPT Canvas streamlines the entire process by offering tools to explore topics, verify facts, and refine your arguments. Its readability and visual integration features ensure that your essays are both compelling and accessible. Topic Exploration: Use web searches to dive into …
When to Use ChatGPT O1 Model
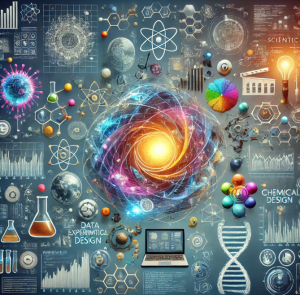
Knowing when to use the LLM such as the ChatGPT O1 model is key to unlocking its full potential. For example, the O1 model is particularly beneficial in scenarios such as analyzing large datasets for patterns in genomics, designing experiments to test novel chemical reactions, or creating algorithms to optimize workflows in computational biology. These applications highlight its ability to address diverse and intricate challenges. Designed to address complex, multifaceted challenges, the O1 model shines when diverse expertise—spanning data analysis, experimental design, coding, and beyond—is required. Let’s delve into these capabilities to understand when and how they can be effectively applied to drive groundbreaking advancements across various fields. Data Analysis …
Agentic Reasoning Design Patterns in AI: Examples
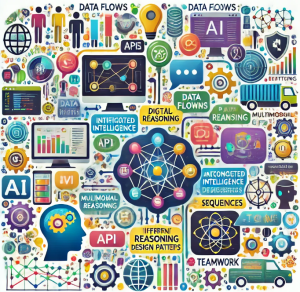
In recent years, artificial intelligence (AI) has evolved to include more sophisticated and capable agents, such as virtual assistants, autonomous robots, and conversational large language models (LLMs) agents. These agents can think, act, and collaborate to achieve complex goals. Agentic Reasoning Design Patterns help explain how these agents work by outlining the essential strategies that AI agents use for reasoning, decision-making, and interacting with their environment. What is an AI Agent? An AI agent, particularly in the context of LLM agents, is an autonomous software entity capable of perceiving its environment, making decisions, and taking actions to achieve specific goals. LLMs enable these agents to understand natural language and reason …
LLMs for Adaptive Learning & Personalized Education
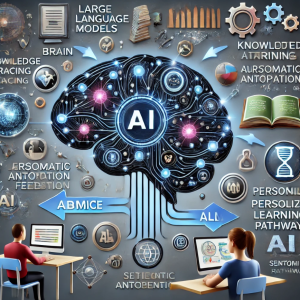
Adaptive learning helps in tailoring learning experiences to fit the unique needs of each student. In the quest to make learning more personalized, Large Language Models (LLMs) with their capability to understand and generate human-like text, offer unprecedented opportunities to adaptively support and enhance the learning process. In this blog, we will explore how adaptive learning can leverage LLMs by integrating Knowledge Tracing (KT), Semantic Representation Learning, and Automated Knowledge Concept Annotation to create a highly personalized and effective educational experience. What is Adaptive Learning? Adaptive learning refers to a method of education that levereges technology to adjust the type and difficulty of learning content based on individual student performance. …
Completion Model vs Chat Model: Python Examples
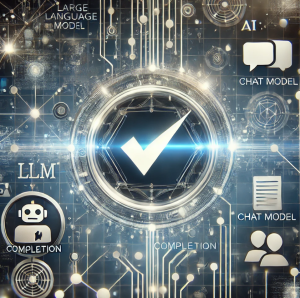
In this blog, we will learn about the concepts of completion and chat large language models (LLMs) with the help of Python examples. What’s the Completion Model in LLM? A completion model is a type of LLM that takes a text input and generates a text output, which is called a completion. In other words, a completion model is a type of LLM that generates text that continues from a given prompt or partial input. When provided with an initial piece of text, the model uses its trained knowledge to predict and generate the most likely subsequent text. A completion model can generate summaries, translations, stories, code, lyrics, etc depending on …
LLM Hosting Strategy, Options & Cost: Examples
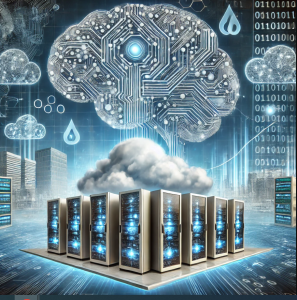
As part of laying down application architecture for LLM applications, one key focus area is LLM deployments. Related to LLM deployment is laying down LLM hosting strategy as part of which different hosting options need to be looked at, and evaluated based on various criteria including cost and appropriate hosting should be selected. In this blog, we will learn about different hosting options for different kinds of LLM and related strategies. LLM Hosting Cost depends on the type of LLM Needed What is going to be the cost related to LLM hosting depends upon the type of LLM we need for our application. LLM Hosting Cost for Proprietary Models If …
Application Architecture for LLM Applications: Examples
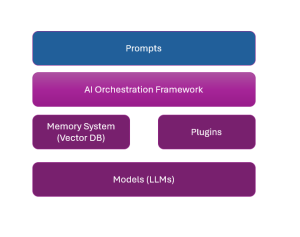
Large language models (LLMs), also termed large foundation models (LFMs), in recent times have been enabling the creation of innovative software products that are solving a wide range of problems that were unimaginable until recent times. Different stakeholders in the software engineering and AI arena need to learn about how to create such LLM-powered software applications. And, the most important aspect of creating such apps is the application architecture of such LLM applications. In this blog, we will learn about key application architecture components for LLM-based applications. This would be helpful for product managers, software architects, LLM architects, ML engineers, etc. LLMs in the software engineering landscape are also termed …
Attention Mechanism in Transformers: Examples
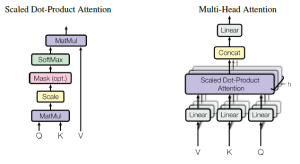
Last updated: 1st Feb, 2024 The attention mechanism allows the model to focus on relevant words or phrases when performing NLP tasks such as translating a sentence or answering a question. It is a critical component in transformers, a type of neural network architecture used in NLP tasks such as those related to LLMs. In this blog, we will delve into different aspects of the attention mechanism (also called an attention head), common approaches (such as self-attention, cross attention, etc.) to calculating and implementing attention, and learn the concepts with the help of real-world examples. You can get good details in this book: Generative Deep Learning by David Foster. You …
Large Language Models (LLMs): Types, Examples
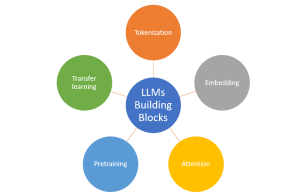
Last updated: 31st Jan, 2024 Large language models (LLMs), being the key pillar of generative AI, have been gaining traction in the world of natural language processing (NLP) due to their ability to process massive amounts of text and generate accurate results related to predicting the next word in a sentence, given all the previous words. These different LLM models are trained on a large or broad corpus of text datasets, which contain hundreds of millions to billions of words. LLMs, as they are known, rely on complex algorithms including transformer architectures that shift through large datasets and recognize patterns at the word level. This data helps the LLMs better understand …
LLM Optimization for Inference – Techniques, Examples
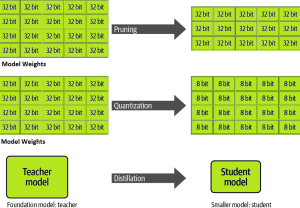
One of the common challenges faced with the deployment of large language models (LLMs) while achieving low-latency completions (inferences) is the size of the LLMs. The size of LLM throws challenges in terms of compute, storage, and memory requirements. And, the solution to this is to optimize the LLM deployment by taking advantage of model compression techniques that aim to reduce the size of the model. In this blog, we will look into three different optimization techniques namely pruning, quantization, and distillation along with their examples. These techniques help model load quickly while enabling reduced latency during LLM inference. They reduce the resource requirements for the compute, storage, and memory. …
LLM Training & GPU Memory Requirements: Examples
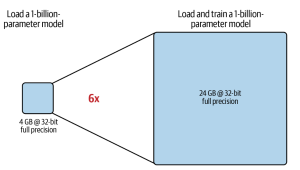
As data scientists and MLOps Engineers, you must have come across the challenges related to managing GPU requirements for training and deploying large language models (LLMs). In this blog, we will delve deep into the intricacies of GPU memory demands when dealing with LLMs. We’ll learn with the help of various examples to better understand how GPU memory impacts the performance and feasibility of training these LLMs. Whether you’re planning to train a foundation (pre-trained) model or fine-tuning an existing model, the insights are aimed to guide you through the crucial considerations of GPU memory allocation. Greater details can be found in this book: Generative AI on AWS. Understanding GPU …
Instruction Fine-tuning LLM Explained with Examples
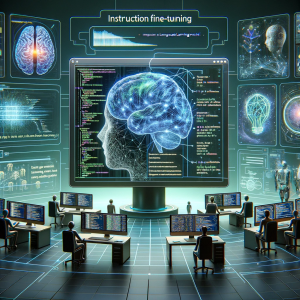
A pre-trained or foundation model is further trained (or fine-tuned) with instructions datasets to help them learn about your specific data and perform humanlike tasks. These models are called instruction fine-tuning LLMs. In this blog, we will learn about the concepts and different examples of instruction fine-tuning models. You might want to check out this book to learn more: Generative AI on AWS. What are Instruction fine-tuning LLMs? Instruction fine-tuning LLMs, also called chat or instruct models, are created by training pre-trained models with different types of instructions. Instruction fine-tuning can be defined as a type of supervised machine learning that improves the foundation model by continuously comparing the model’s …
Distributed LLM Training & DDP, FSDP Patterns: Examples
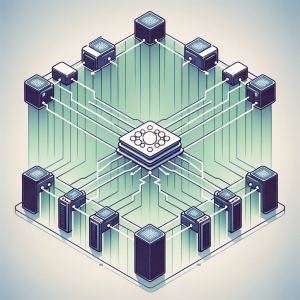
Training large language models (LLMs) like GPT-4 requires the use of distributed computing patterns as there is a need to work with vast amounts of data while training with LLMs having multi-billion parameters vis-a-vis limited GPU support (NVIDIA A100 with 80 GB currently) for LLM training. In this blog, we will delve deep into some of the most important distributed LLM training patterns such as distributed data parallel (DDP) and Fully sharded data parallel (FSDP). The primary difference between these patterns is based on how the model is split or sharded across GPUs in the system. You might want to check out greater details in this book: Generative AI on …
I found it very helpful. However the differences are not too understandable for me