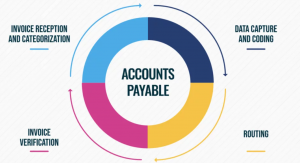
The machine learning for accounts payable market is expected to grow from $6.1 million in 2016 to $76.8 million by 2021, at a compound annual growth rate (CAGR) of 53 percent. The software industry is rapidly embracing machine learning for account payable. As account payable becomes more automated, it also becomes more data-driven. Machine learning is enabling account payables stakeholders to leverage powerful new capabilities in this arena. In this blog post, you will learn machine learning / deep learning / AI use cases for account payable.
What is Accounts Payable?
Account payable is a crucial part of the business process because it helps to ensure that businesses have the funds available to pay for goods and services when they are received. Account payable involves creating invoices, issuing payments, and maintaining records of payments. Account payable also helps to keep track of expenses and can be used to create financial reports.
Account payable is an amount which a company owes to its suppliers, whereas account receivables are the amount which a company receives from its customers. Account payable arises when a company purchases goods or services on credit from its supplier, and account receivables arise when a company sells goods or services on credit to its customers. The key difference between account payable and account receivables is that account payable is an amount which a company owes to its suppliers, while account receivables are the amount which a company receives from its customers.
Key Business Processes for Accounts Payable
Here are the key business processes in relation to Accounts Payable:
- Supplier onboarding: Supplier onboarding is the process of adding new suppliers to the system. It can be a time-consuming and manual process, requiring the input of data into multiple systems. Account payable departments can streamline the supplier onboarding process by automating the data entry. This will allow them to focus on more important tasks, such as negotiating payment terms with suppliers. In addition, automating the supplier onboarding process will ensure that all data is entered correctly and consistently, reducing the risk of errors.
- Maintain suppliers’ contract terms and conditions: One of the most important things to consider when setting up an account payable system is the terms and conditions of your supplier contracts. Account payable involves the payment of money to suppliers in exchange for goods or services. In order to ensure that this process runs smoothly, it is important to carefully review the contract terms and conditions before signing any agreements. This will help to avoid any misunderstandings or disagreements down the line. Payment terms should be clearly stated in the contract, and both parties should agree on a schedule for payments. It is also important to include language indicating that the organization has the right to inspections goods or services before making any payments. By taking these steps, organizations can protect themselves from supplier disputes and ensure that account payable runs smoothly.
- Purchase order (PO) creation & amendment: Create purchase orders for all transactions involving new vendor agreements. The purchase order is essentially the first step of the procurement process, which can impact costs depending on how it’s structured and where it fits in with any relevant compliance requirements. In addition to creating purchase orders, organizations also use the purchase order system to amend or cancel existing orders.
- Transaction approval/payment authorization: All finance professionals are familiar with the request to just approve this transaction so we can get it paid. The challenge is that these requests come infrequently, which means replying takes up a lot of time. An automated system would reduce the number of manual approvals required at this stage of the payables process and increase productivity.
- Match suppliers with specific transactions: Account payables stakeholders have to process thousands of invoices every month, so it’s important for them to find those that match specific transactions quickly. It can be challenging for employees to keep track of all the possible invoices and figure out which ones need to be paid, especially because many vendors use unique invoice numbers or codes.
- Reconcile suppliers’ invoices with purchases: This step of the payables process is familiar to anyone who works in an accounting department and it’s when each invoice submitted by a supplier is analyzed against transactions in the purchase order system, purchase requisition system, and so on before matching them up to ensure the numbers match up.
- Payment processing: Process all transactions that have been approved for payment. At this stage of the payables process, organizations generate invoices, credit memos, or debit memos before making any payments to vendors. These documents are then submitted into an electronic workflow system for approval by authorized users. Once they’re approved, organizations can use the transaction processing system to make the payments.
- Review or Amend suppliers’ account balances: The final step in the entire payables process is to review the balance owed by each vendor to ensure that it reflects any transactions that have occurred since the last time this information was collected. Once again, these changes are then submitted into an electronic workflow system for approval before they can be recorded in accounting systems where they’ll be used to generate any reports you might need for your own internal purposes.
- Detect duplicate payments to suppliers/vendors: This step of the payables process is also familiar to accounting professionals and it’s when you’re required to identify any transactions that might have been paid twice, which can happen if a payment gets split between two different accounts or if someone manually corrects an entry in your system before entering it into another one.
- Manage suppliers ratings and profiles: Organizations can use the information collected about their vendors in different stages of this process to generate reports that show which ones are more important than others in terms of how much they buy or sell or simply rate them on a scale to determine who’s acting in good faith when there are disagreements.
- Evaluate suppliers for redundancies: This step of the payables process is also familiar to accounting professionals and it’s when they are required to look for any opportunities to get rid of unnecessary expenses. The idea is to reduce expenses without hurting the business.
- Supplier fraud identification: The entire process of paying bills involves a lot of manual work which means it’s always at risk for human error. However, employees are also the first line of defense against fraudulent activity in your accounts payable department.
Machine learning techniques used for Accounts Payables
Machine learning techniques are being used in the ever-evolving world of accounts payables. Different machine learning models can be trained to address challenges across different business processes in accounts payables listed in the previous section. Here are some of the key machine learning use cases for account payables:
Invoice matching for payment
Machine learning can be used to match invoices with purchase orders (POs) and receipts based on the description within the invoice itself, which in turn will determine whether or not payment should be made. OCR techniques can be implemented to read information from POs, and an invoice, such as its date, amount, customer ID, and so on. Invoice matching involves sorting through invoices to find the correct one, or correct group of invoices, for payment. Machine learning algorithms used for invoice matching include clustering algorithms, classification algorithms such as ensemble techniques, SVM, etc. Clustering involves grouping similar invoices together to determine if payments should be made. Classification models are used to classify whether payment should be made.
Invoice matching is part of invoice automation which has seen lot of traction in recent times (post Covid) as part of digital transformation. Corcentric is one such company which is providing solutions in relation to invoice matching. Use of AI / machine learning for invoice matching is increasingly getting adopted as part of automation invoicing workflows. One of the key challenges in automation of invoice matching is exception handling when the POs and invoices are unable to be matched with very high confidence. AI / machine learning solutions should rather flag such instances for manual exception handling.
Invoice matching is key to enhancing strategic relationships with suppliers. AI-enabled invoice matching enables account payables department to make the payments in a swift manner. In addition, faster payments of invoices (within 24-48 hours) can provide leverage to the buyers to ask for discounts from the suppliers.
Matching suppliers for payment
Machine learning can be used to determine or rank the suppliers that should receive payment earlier others. The classification model will learn from historical invoice matching data within an account payable to understand which suppliers are likely to have invoices to be paid. Then, machine learning models can be trained to rank/sort these invoices to find the correct one, or set of invoices, to pay.
Fraudulent invoices
Machine learning models can be used to classify the invoices that are fraudulent, or potentially fraudulent. The classification model will learn from the account payable’s previous payment transactions to analyze if any invoice is suspicious. For example, an invoice might be flagged as a potential fraudster if it was paid by a certain customer in the past but is now being transferred to a different customer. Another example is related to classifying duplicate payment transactions. If an invoice has been paid before but is now being re-submitted, it could be a sign of fraudulent activity.
Supplier performance assessment
Machine learning models can be used to score the suppliers or vendors based on their invoices and related payment transactions. The machine learning model will learn from historical data, such as invoice amounts, late payments, etc., to forecast future supplier performances.
Redundant spend classification and supplier evaluation
Machine learning models can be used to classify the invoices that fall into certain categories, such as those that are frequently unpaid, those that have been paid frequently but should not be paid again, etc. The classification model will learn from historical transaction data within an account payable to understand which invoices should be placed into these categories.
Conclusion
Use cases for machine learning in accounts payable are increasing in number. There is a lot of manual work involved in account payables, and machine learning techniques can be used to make the process more efficient. It’s critical for your small business to stay competitive that you keep up with these trends by looking into machine learning possibilities for account payable procedures like invoice matching or fraud detection. We hope this blog post has helped you figure out how to get started training machine-learning models for account payable activities in your own company without spending too much time reading about it yourself. For assistance applying any of these machine-learning techniques to your company’s accounting processes, please get in touch with us right away.
- Questions to Ask When Thinking Like a Product Leader - July 3, 2025
- Three Approaches to Creating AI Agents: Code Examples - June 27, 2025
- What is Embodied AI? Explained with Examples - May 11, 2025
I found it very helpful. However the differences are not too understandable for me