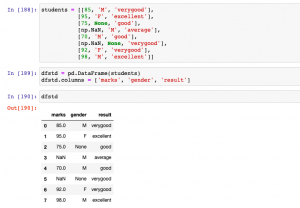
In this post, you will learn about how to use Python’s Sklearn SimpleImputer for imputing / replacing numerical & categorical missing data using different strategies. In one of the related article posted sometime back, the usage of fillna method of Pandas DataFrame is discussed. Handling missing values is key part of data preprocessing and hence, it is of utmost importance for data scientists / machine learning Engineers to learn different techniques in relation imputing / replacing numerical or categorical missing values with appropriate value based on appropriate strategies.
SimpleImputer Python Code Example
SimpleImputer is a class in the sklearn.impute module that can be used to replace missing values in a dataset, using a variety of input strategies. SimpleImputer is designed to work with numerical data, but can also handle categorical data represented as strings. SimpleImputer can be used as part of a scikit-learn Pipeline. The default strategy is “mean”, which replaces missing values with the median value of the column. Other options include “most_frequent” (which replaces missing values with the most common value in the column) and “constant” (which replaces missing values with a constant value). SimpleImputer can also be used to impute multiple columns at once by passing in a list of column names. SimpleImputer will then replace missing values in all of the specified columns. When using SimpleImputer, it is important to consider whether or not imputing is the best option for your data. In some cases, it may be better to drop rows or columns with missing values instead of imputing them.
SimpleImputer class is used to impute / replace the numerical or categorical missing data related to one or more features with appropriate values such as following:
- Mean: When SimpleImputer() is invoked without any arguments, it defaults to using the mean strategy. Missing values get replaced with the mean along each column. This strategy can only be used with numeric data.
- Median: Missing values get replaced with the median along each column. This strategy can only be used with numeric data.
- Most frequent (mode): Missing values get replaced with the most frequent value along each column. This strategy can be used with strings or numeric data.
- Constant: Missing values get replaced with the fill_value. This strategy canbe used with strings or numeric data. .
Each of the above type represents strategy when creating an instance of SimpleImputer. Here is the Python code sample representing the usage of SimpleImputor for replacing numerical missing value with the mean.
First and foremost, let’s create a sample Pandas Dataframe representing marks, gender and result of students.
import pandas as pd
import numpy as np
students = [[85, 'M', 'verygood'],
[95, 'F', 'excellent'],
[75, None,'good'],
[np.NaN, 'M', 'average'],
[70, 'M', 'good'],
[np.NaN, None, 'verygood'],
[92, 'F', 'verygood'],
[98, 'M', 'excellent']]
dfstd = pd.DataFrame(students)
dfstd.columns = ['marks', 'gender', 'result']
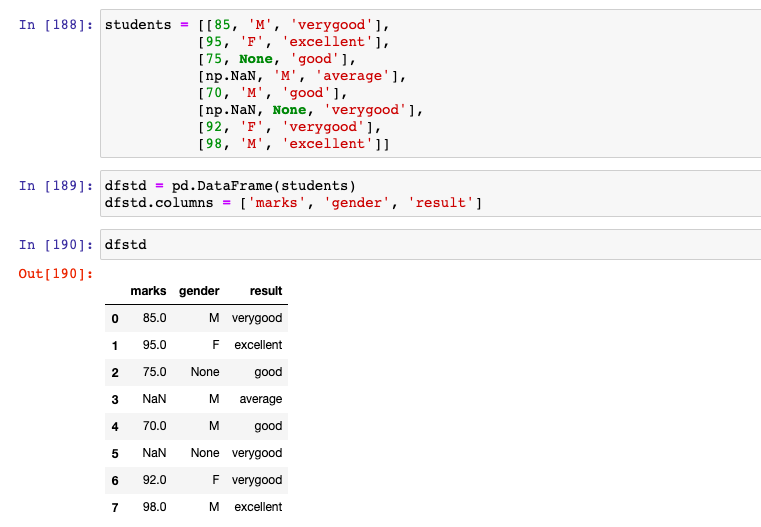
There are two columns / features (one numerical – marks, and another categorical – gender) which are having missing values and need to be imputed. In the code below, an instance of SimpleImputer is created with strategy as “mean”. The missing value is represented using NaN. Note some of the following:
- sklearn.impute package is used for importing SimpleImputer class.
- SimpleImputer takes two argument such as missing_values and strategy.
- fit_transform method is invoked on the instance of SimpleImputer to impute the missing values.
from sklearn.impute import SimpleImputer
#
# Missing values is represented using NaN and hence specified. If it
# is empty field, missing values will be specified as ''
#
imputer = SimpleImputer(missing_values=np.NaN, strategy='mean')
dfstd.marks = imputer.fit_transform(dfstd['marks'].values.reshape(-1,1))[:,0]
dfstd
Here is how the output would look like. Note that missing value of marks is imputed / replaced with the mean value, 85.83333
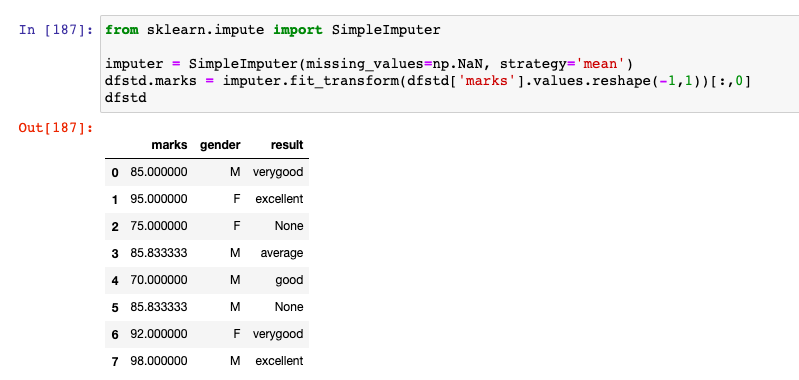
SimpleImputer for imputing Numerical Missing Data
For the numerical missing data, the following strategy can be used.
- Mean
- Median
- Most frequent (mode)
- Constant
The code example below represents the instantiation of SimpleImputer with appropriate strategies for imputing numerical missing data
#
# Imputing with mean value
#
imputer = SimpleImputer(missing_values=np.NaN, strategy='mean')
#
# Imputing with median value
#
imputer = SimpleImputer(missing_values=np.NaN, strategy='median')
#
# Imputing with most frequent / mode value
#
imputer = SimpleImputer(missing_values=np.NaN, strategy='most_frequent')
#
# Imputing with constant value; The command below replaces the missing
# value with constant value such as 80
#
imputer = SimpleImputer(missing_values=np.NaN, strategy='constant', fill_value=80)
SimpleImputer for imputing Categorical Missing Data
For handling categorical missing values, you could use one of the following strategies. However, it is the “most_frequent” strategy which is preferably used.
- Most frequent (strategy=’most_frequent’)
- Constant (strategy=’constant’, fill_value=’someValue’)
Here is how the code would look like when imputing missing value with strategy as most_frequent. In the code sample used in this post, gender is having missing values. Note how the missing value under gender column is replaced with ‘M’ which occurs most frequently.
from sklearn.impute import SimpleImputer
imputer = SimpleImputer(missing_values=None, strategy='most_frequent')
dfstd.gender = imputer.fit_transform(dfstd['gender'].values.reshape(-1,1))[:,0]
dfstd
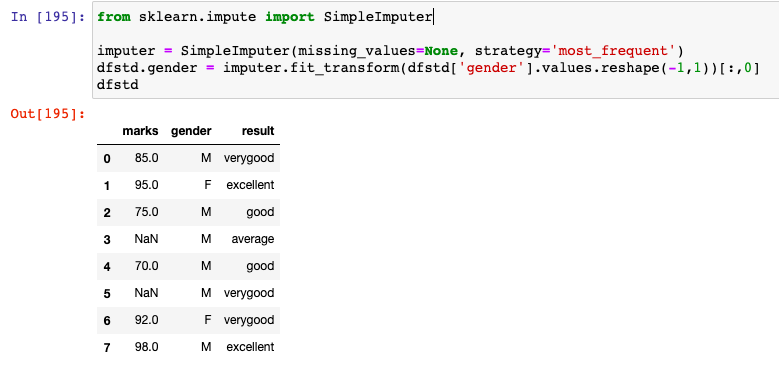
Here is how the code would look like when imputing missing value with strategy as constant. Note how the missing value under gender column is replaced with ‘F’ which is assigned using fill_value parameter.
from sklearn.impute import SimpleImputer
imputer = SimpleImputer(missing_values=None, strategy='constant', fill_value='F')
dfstd.gender = imputer.fit_transform(dfstd['gender'].values.reshape(-1,1))[:,0]
dfstd
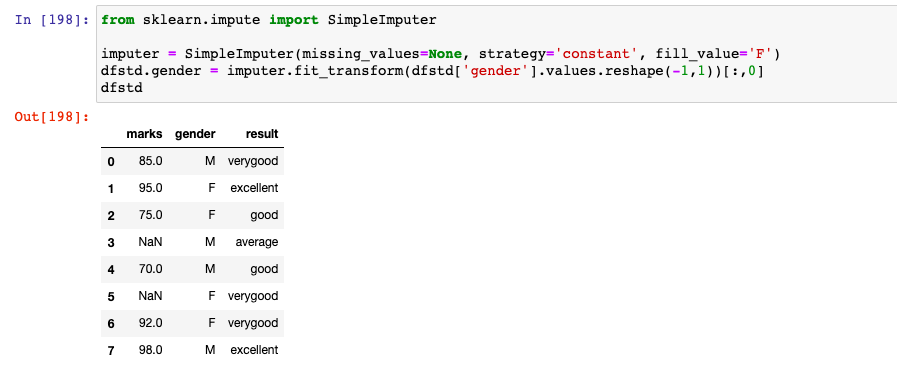
Conclusions
Here is the summary of what you learned in this post:
- You can use Sklearn.impute class SimpleImputer to impute / replace missing values for both numerical and categorical features.
- For numerical missing values, strategy such as mean, median, most frequent and constant can be used.
- For categorical features, strategy such as most frequent and constant can be used.
- What is Embodied AI? Explained with Examples - May 11, 2025
- Retrieval Augmented Generation (RAG) & LLM: Examples - February 15, 2025
- How to Setup MEAN App with LangChain.js - February 9, 2025
I found it very helpful. However the differences are not too understandable for me