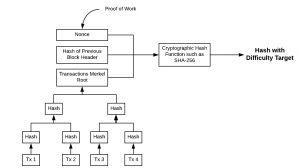
In this post, you will learn about what is proof of work in a Bitcoin Blockchain.
Simply speaking, the proof of work in computing is used to validate whether the user has put enough effort (such as computing power) or done some work for solving a mathematical problem of a given complexity, before sending the request. For example, Go to any online SHA 256 calculator tool and try using a random number with the text “Hello World” as shown in the below screenshot. If the difficulty target is set as the hash value starting with one zero, you could see that the random number 10 results in hash value starting with zero. The number 10 is guessed in the ransom manner.
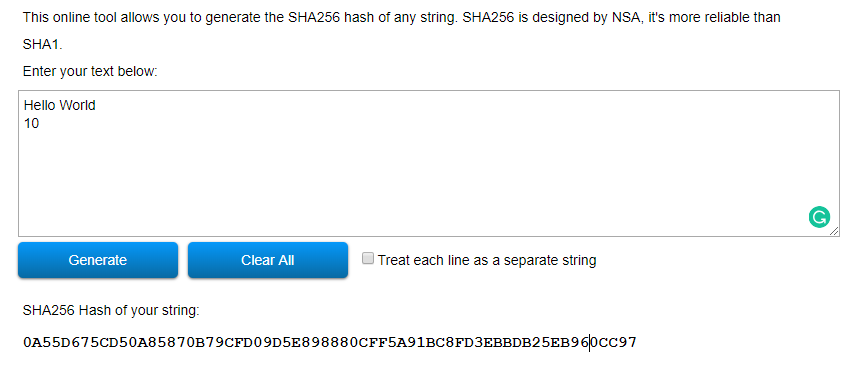
Figure 1. Hash String starting with one zero
In Bitcoin Blockchain, the proof of work is represented as a nonce (a random number) which is used with the transactions Merkel root and the hash of previous block (the reference to the previous block) and passed to the cryptographic hash function (SHA-256) to find the hash value with the pre-defined value of the difficulty target. The difficulty target is the leading number of zeros (0s) with which hash value starts. Each time a different value of nonce is passed until the difficulty target is met.
Hypothetically speaking, the following is the mathematical representation:
SHA-256(SHA-256(Previous Block Header), Merkel Root, Nonce) => Difficulty target
The following diagram represents the proof of work in Bitcoin Blockchain.
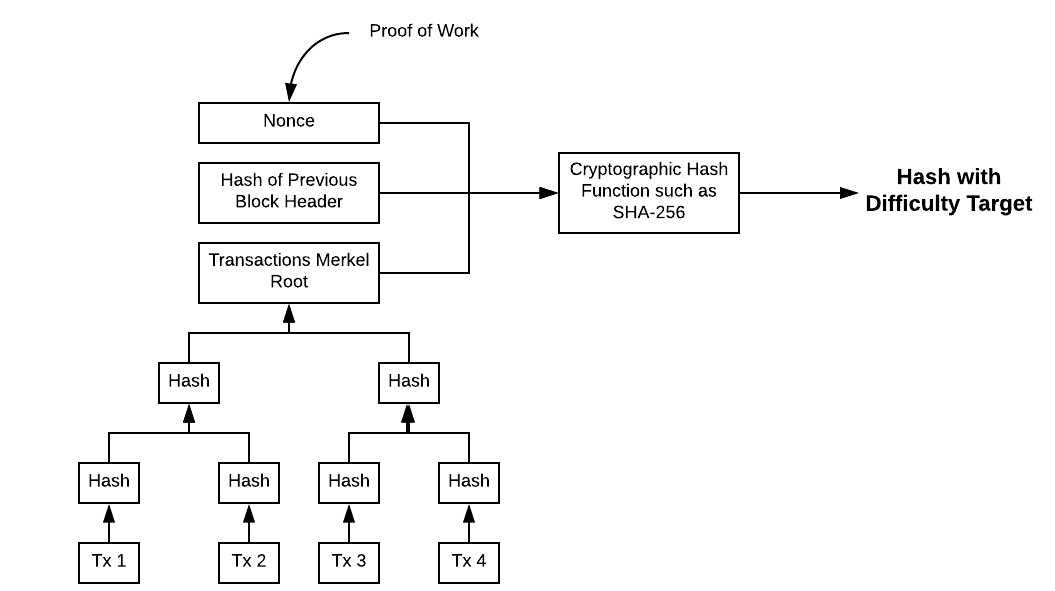
Figure 2. Proof of Work in Bitcoin Blockchain
The following are some important details:
- For the first block, the previous block header hash is a 32-byte field of just zeros and the transaction list is empty.
- The hash Id of the block, which acts as a hash of the previous block, is created using the hash of the block header (80 bytes) which comprises of the following information:
- Merkel root (32 bytes)
- Previous block header hash (32 bytes)
- Nonce (4 bytes)
- Timestamp (4 bytes)
- Version (4 bytes)
- Difficulty target (4 bytes)
References
- Feature Engineering in Machine Learning: Python Examples - May 3, 2024
- Feature Selection vs Feature Extraction: Machine Learning - May 2, 2024
- Model Selection by Evaluating Bias & Variance: Example - May 2, 2024
Leave a Reply