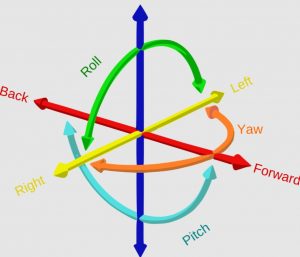
The degree of freedom (DOF) is a term that statisticians use to describe the degree of independence in statistical data. A degree of freedom can be thought of as the number of variables that are free to vary, given one or more constraints. When you have one degree, there is one variable that can be freely changed without affecting the value for any other variable. As a data scientist, it is important to understand the concept of degree of freedom, as it can help you do accurate statistical analysis and validate the results. In this blog, we will explore the meaning of degree of freedom in statistics, its importance in statistical analysis, and provide examples of how it is used in different statistical tests.
What is degree of freedom?
The degree of freedom is defined as the number of variables that are free to vary in a statistical setting. In statistical testing, degrees of freedom refer to the number of values in a sample that are free to vary without changing the number of samples or observations. For example, consider the following set of data:
Weights of 5 items in a box weighing 12KG = {4, 2.5, 3.5, 1, 1}
There are 5 items in the box with four degrees of freedom. This means that only four items are free to vary in terms of weights because the weight of fifth item must be equal to difference between 12 KG and sum of weights of four items. Thus, four things can vary in terms of its weights in above example.
Several statistical tests use the concept of degrees of freedom, including t-tests, F-tests, chi-squared tests, and ANOVA. Here are details:
- The T-test is a statistical test that is used to determine whether two groups are significantly different from each other. The degree of freedom in a t-test is the number of observations minus the number of parameters estimated, which is usually one for a two-sample t-test.
- In an F-test, degrees of freedom refer to the number of independent observations that are available to estimate the variance of a population. Specifically, in an F-test, there are two sets of degrees of freedom: the degrees of freedom for the numerator and the degrees of freedom for the denominator. The numerator degrees of freedom (DFn) represent the number of independent observations used to estimate the variance of the first group or population. The denominator degrees of freedom (DFd) represent the number of independent observations used to estimate the variance of the second group or population. The formula for calculating degrees of freedom for the numerator is n1 – 1, and denominator is n2 – 1, where n1 and n2 are the number of observations in the two groups (being compared) belonging to numerator and denominator respectively.
- The chi-squared test is used to determine whether there is a significant association between two categorical variables, and the degree of freedom in this test depends on the number of categories. The formula for calculating degrees of freedom in a chi-square test is (r – 1) x (c – 1), where r is the number of rows in the contingency table and c is the number of columns.
Example – Degrees of freedom when finding about the traffic light
Let’s say that you are waiting at the traffic signal and someone gave you a call to find out what signal is on at present. If the person knows which two out of three signals (red, orange or green) is not on, he or she would be able to tell the actual signal. Thus, the degree of freedom is two.
Example – Degrees of freedom for calculating mean
To calculate the mean of the sample data, the degrees of freedom is equal to count of the data in the sample that are free to vary. For example, in the example given below, the degrees of freedom is 5. This means that all 5 data is equally independent to vary.
Weights of 5 items in a box weighing 12KG = {4, 2.5, 3.5, 1, 1}
Thus, if there are N items and the ask is to find mean, the degrees of freedom will be N.
Example – Degrees of freedom for calculating standard deviation
To calculate the standard deviation of the sample data given the mean is provided, the degrees of freedom is equal to count of the data in the sample that are free to vary. For example, in the example given below, the degrees of freedom is 4. This means that Only 4 data is free to vary.
Mean of weights of 5 items in a box = 2.4 KG
If we know weights of four items, we will be able to calculate the standard deviation without knowing the fifth one. For example, lets say the weights of four items are {2, 3, 1, 1}. The weight of fifth item will be 5 x 2.4 – {2+3+1.5+2.5} = 3 KG.
Thus, if there are N items and the ask is to find standard deviation given the mean, the degrees of freedom will be (N – 1).
Example – Degrees of freedom for 1-sample t-test
Degrees of freedom for 1-sample t-test is calculated as = N – 1
If the mean of the sample and the population mean is known, only (N – 1) values are free to change.
Example – Degrees of freedom for 2-sample t-test
Degrees of freedom for 2-sample t-test is having N1 and N2 observations can be calculated as the following:
= (N1 – 1) + (N2 – 1)
= N1 + N2 – 2
If the mean of the both the samples and the population mean is known, only (N1 – 1) values from first sample and (N2 – 1) values from second sample are free to change.
A degree of freedom (DOF) is calculated as the number of independent observations or measurements that can be made in order to calculate some statistics such as mean, standard deviation, chi-square, t-score etc. There are many examples where degrees of freedom come up when calculating different statistics. In case, you would like to learn more details, please feel free to reach out or comment.
- Feature Engineering in Machine Learning: Python Examples - May 3, 2024
- Feature Selection vs Feature Extraction: Machine Learning - May 2, 2024
- Model Selection by Evaluating Bias & Variance: Example - May 2, 2024
Nice Article
Thank you
[…] is unknown and the sample size is small. The distribution used is T-distribution with certain degrees of freedom. A sample of size lesser than 30 observations is considered as a small […]