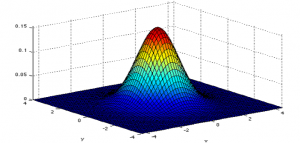
Following are the key points described later in this article:
- When to Use Linear Kernel
- When to Use Gaussian Kernel
When to Use Linear Kernel
In case there are large number of features and comparatively smaller number of training examples, one would want to use linear kernel. As a matter of fact, it can also be called as SVM with No Kernel. One may recall that SVM with no kernel acts pretty much like logistic regression model where following holds true:
- Predict Y = 1 when W.X >= 0. Note that, in the prior equation, W is actually W transpose and also includes bias factor.
- Predict Y = 0 when W.X < 0.
Simply speaking, one may want to use SVM with linear kernel when data distribution is linearly separable.
When to Use Gaussian Kernel
In scenarios, where there are smaller number of features and large number of training examples, one may use what is called Gaussian Kernel. When working with Gaussian kernel, one may need to choose the value of variance (sigma square). The selection of variance would determine the bias-variance trade-offs. Higher value of variance would result in High bias, low variance classifier and, lower value of variance would result in low bias/high variance classifier.
- Retrieval Augmented Generation (RAG) & LLM: Examples - February 15, 2025
- How to Setup MEAN App with LangChain.js - February 9, 2025
- Build AI Chatbots for SAAS Using LLMs, RAG, Multi-Agent Frameworks - February 8, 2025
I found it very helpful. However the differences are not too understandable for me