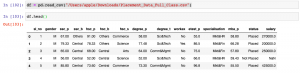
Last updated: 13 Sept, 2024
In this post, you will learn about the concept of encoding such as Label Encoding used for encoding categorical features while training machine learning models. Label encoding technique is implemented using sklearn LabelEncoder. You would learn the concept and usage of sklearn LabelEncoder using code examples, for handling encoding labels related to categorical features of single and multiple columns in Python Pandas Dataframe. The following are some of the points which will get covered:
- Background
- What are labels and why encode them?
- How to use LabelEncoder to encode single & multiple columns (all at once)?
- When not to use LabelEncoder?
Background
When working with dataset having categorical features, you come across two different types of features such as the following. Many machine learning algorithms require the categorical data (labels) to be converted or encoded in the numerical or number form.
- Ordinal features – Features which has some order. For example, t-shirt size feature can have values in [‘small’, ‘medium’, ‘large’, ‘extra large’]. You may note that there is an order to the values.
- Nominal features – Features which are just labels or names and don’t have any order. For example, if the color of car is a feature, color can take value such as [‘white’, ‘red’, ‘black’, ‘blue’]. You may note there is no order to the value of the color features.
Both of the above type of categorical features need to be converted into number / integer form as machine learning models can only be trained using numbers.
This is where encoding techniques such as label encoding and one-hot encoding comes into picture. Classes such as LabelEncoder or OneHotEncoder comes into picture which are part of sklearn.preprocessing module. It is recommended to use OneHotEncoder in place of LabelEncoder. OneHotEncoder is discussed in this post – One-hot Encoding Concepts & Python Examples.
What are Labels and Why Encode them?
Labels represents string labels of both ordinal and nominal features in relation to categorical feature set. Some labels may have order associated with them (ordinal features) while others may not have any orders associated with them (nominal features).
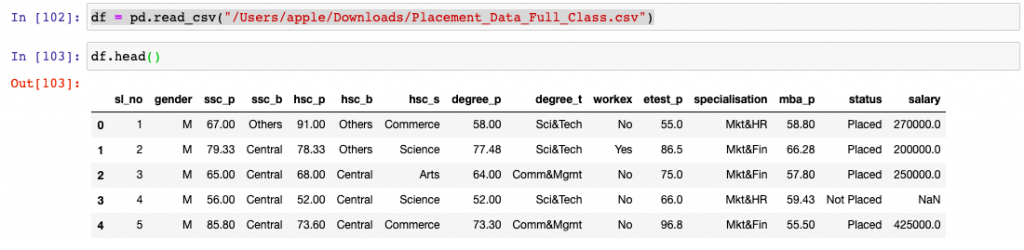
In the above table, note the categorical features such as hsc_s having labels such as Commerce, Science, Arts, etc. Another categorical features is workex which has value such as No, Yes. We need to encode labels of such categorical features with numbers. Thus, when we encode labels of hsc_s, the values assigned can be 0 for Commerce, 1 for Science, 2 for Arts, etc. Similarly, for workex feature, the values assigned after label encoding can be 0 for No and 1 for Yes.
It is an important part of data preprocessing to encode labels appropriately in numerical form in order to make sure that the learning algorithm interprets the features correctly. In the following section, you will see how you could use LabelEncoder class of sklearn.preprocessing module to encode labels of categorical features.
How to use LabelEncoder to encode single & multiple columns?
In this section, you will see the code example related to how to use LabelEncoder to encode single or multiple columns. LabelEncoder encodes labels by assigning them numbers. Thus, if the feature is color with values such as [‘white’, ‘red’, ‘black’, ‘blue’]., using LabelEncoder may encode color string label as [0, 1, 2, 3]. Here is an example.
The dataset used for illustration purpose is related campus recruitment and taken from Kaggle page on Campus Recruitment. As a first step, the data set is loaded. Here is the python code for loading the dataset once you downloaded it on your system.
import pandas as pd
import numpy as np
df = pd.read_csv("/Users/ajitesh/Downloads/Placement_Data_Full_Class.csv")
df.head()
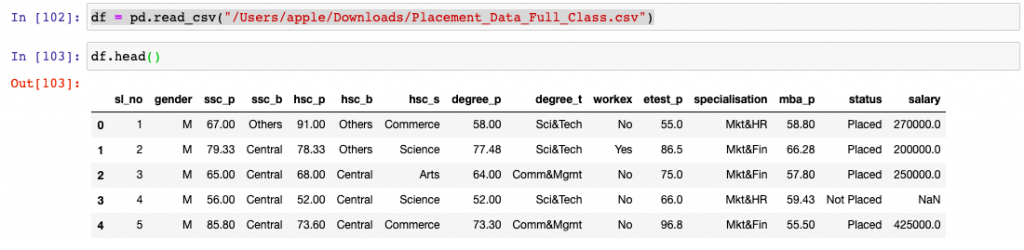
There are several categorical features as shown in the above picture. We will encode single and multiple columns.
Use LabelEncoder to Encode Single Columns
Here is the code which can be used to encode single column such as status.
from sklearn.preprocessing import LabelEncoder
#
# Instantiate LabelEncoder
#
le = LabelEncoder()
#
# Encode single column status
#
df.status = le.fit_transform(df.status)
#
# Print df.head for checking the transformation
#
df.head
Make a note of how the status column value changed from Placed and Not Placed to 1 and 0.
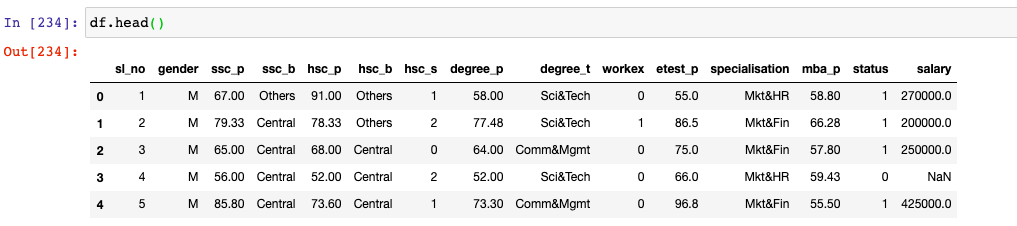
Use LabelEncoder to Encode Multiple Columns All at Once
Here is the code which can be used to encode multiple columns. Let’s say we will like to encode multiple columns such as worke’, status, hsc_s, degree_t. The ask is can we encode multiple columns all at once? LabelEncoder is used in the code given below. Python apply method is used to achieve this.
cols = ['workex', 'status', 'hsc_s', 'degree_t']
#
# Encode labels of multiple columns at once
#
df[cols] = df[cols].apply(LabelEncoder().fit_transform)
#
# Print head
#
df.head()
This is what gets printed. Make a note of how columns related to workex, status, hsc_s, degree_t got encoded with numerical / integer value.
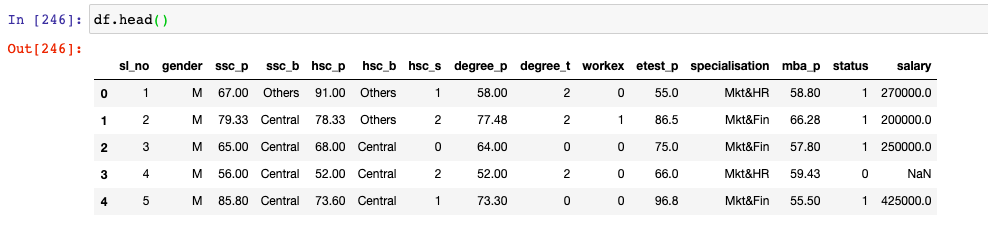
When not to use LabelEncoder?
When LabelEncoder is used with categorical features having multiple values, the integer value such as 0, 1, 2, 3… etc. For example, in above example, the feature hsc_s has three different types of value such as commerce, science and arts. When LabelEncoder is used, they get assigned value of 1, 2, and 0 for commerce, science and arts. The problem that happens is that learning algorithm may take the value of commerce to be larger than the value of science and arts. Although this assumption is incorrect, the algorithm could still produce useful results but not the optimal one. In such cases, it is recommended to use OneHotEncoder.
Differences between Label Encoding & One-hot Encoding
The following are the key differences between label encoding and one-hot encoding:
- One-hot encoding gives every unique value an equal weight, whereas the label encoding may imply that some values may be more important than others.
- Label encoding is more memory efficient. The number of columns is the same before and after encoding, whereas one-hot encoding adds one column per unique value. For very large datasets with thousands of unique values in a categorical column, label encoding requires substantially less memory.
The accuracy of a machine learning model is rarely impacted by the encoding methods including label encoding or one-hot encoding. If in doubt, go with one-hot encoding. However, it is always good to assert oneself by encoding the data both ways and compare the results after training the machine learning model.
- Questions to Ask When Thinking Like a Product Leader - July 3, 2025
- Three Approaches to Creating AI Agents: Code Examples - June 27, 2025
- What is Embodied AI? Explained with Examples - May 11, 2025
Thank you….for your support. you given a good solution for me.