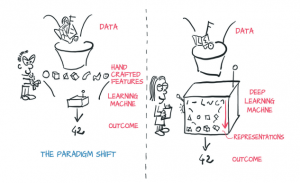
In this post, you learn about what is deep learning with a focus on feature engineering.
Here is a quick diagram which represents the idea behind deep learning that Deep learning is about learning features in an automatic manner while optimizing the algorithm.
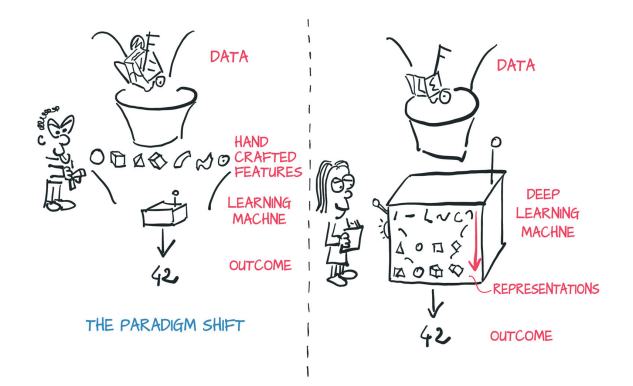
The above diagram is taken from the book, Deep learning with Pytorch. One could learn one of the key differences between training models using machine learning and deep learning algorithms.
With machine learning models, one need to engineer features (called as feature engineering) from the data (also called as representations) and feed these features in machine learning algorithms to train one or more models. The model performance depends on how good the features are. The focus of data scientists while working on machine learning models is to hand-craft some real good features and use most suitable algorithms to come up with high-performance models. The same is represented on the left side of the diagram.
With deep learning models, all one would need is feed data with some initial features / knowledge into deep learning algorithm and the model learns the features (representations) from raw data based on optimizing algorithms. The focus of deep learning practitioners is mostly on operating on a mathematical entity so that it discovers representations (features) from the training data autonomously. The same is represented on the right side of the diagram.
One of the most important reasons why deep learning took off instantly is that it completely automates what used to be the most crucial step in a machine-learning workflow: feature engineering
- How Indexing Works in LLM-Based RAG Applications - January 26, 2025
- Retrieval Augmented Generation (RAG) & LLM: Examples - January 26, 2025
- What are AI Agents? How do they work? - January 7, 2025
I found it very helpful. However the differences are not too understandable for me